Efficient federated learning on resource-constrained edge devices based on model pruning
COMPLEX & INTELLIGENT SYSTEMS(2023)
摘要
Federated learning is an effective solution for edge training, but the limited bandwidth and insufficient computing resources of edge devices restrict its deployment. Different from existing methods that only consider communication efficiency such as quantization and sparsification, this paper proposes an efficient federated training framework based on model pruning to simultaneously address the problem of insufficient computing and communication resources. First, the framework dynamically selects neurons or convolution kernels before the global model release, pruning a current optimal subnet and then issues the compressed model to each client for training. Then, we develop a new parameter aggregation update scheme, which provides training opportunities for global model parameters and maintains the complete model structure through model reconstruction and parameter reuse, reducing the error caused by pruning. Finally, extensive experiments show that our proposed framework achieves superior performance on both IID and non-IID datasets, which reduces upstream and downstream communication while maintaining the accuracy of the global model and reducing client computing costs. For example, with accuracy exceeding the baseline, computation is reduced by 72.27% and memory usage is reduced by 72.17% for MNIST/FC; and computation is reduced by 63.39% and memory usage is reduced by 59.78% for CIFAR10/VGG16.
更多查看译文
关键词
Federated learning,Model pruning,Communication efficient,Edge intelligence
AI 理解论文
溯源树
样例
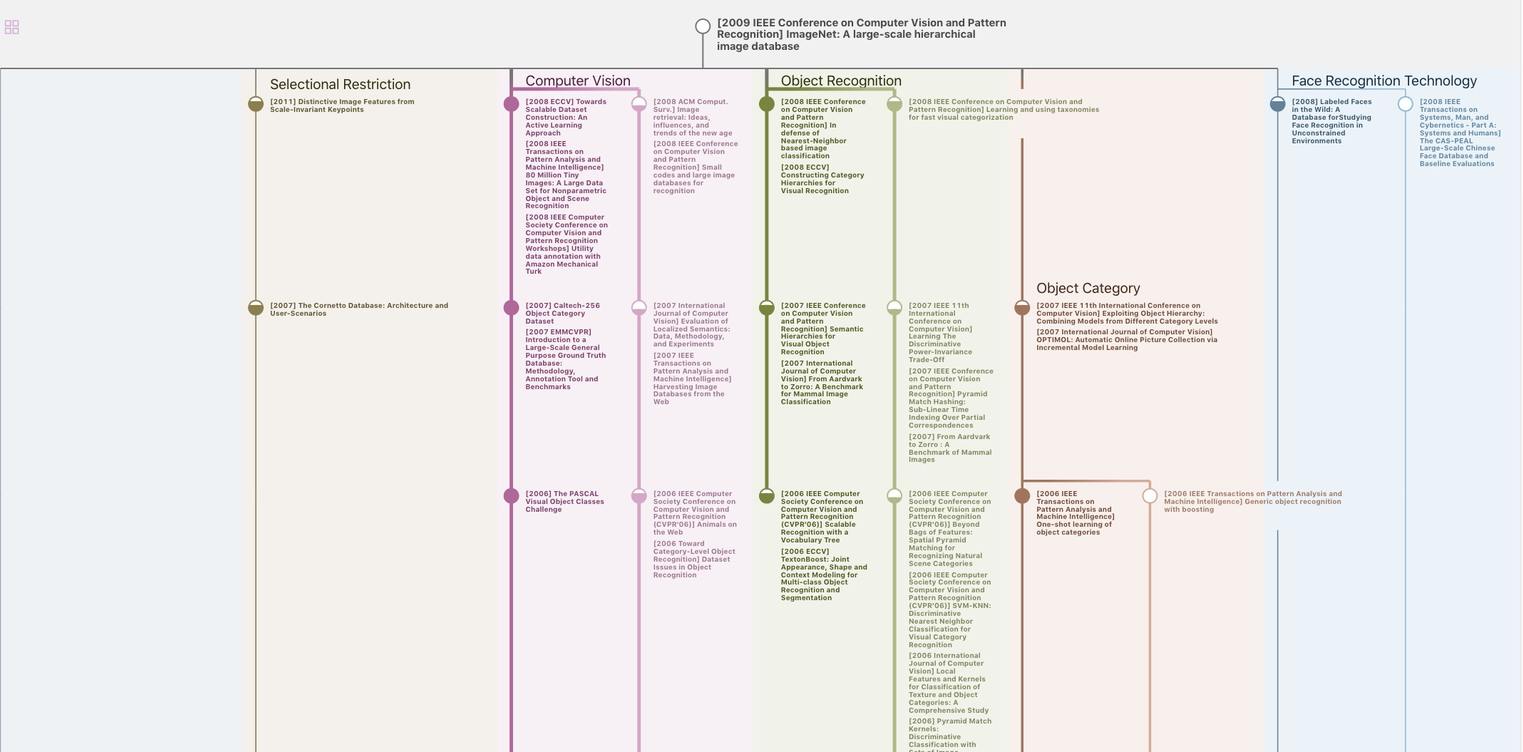
生成溯源树,研究论文发展脉络
Chat Paper
正在生成论文摘要