Robust smoothing of left-censored time series data with a dynamic linear model to infer SARS-CoV-2 RNA concentrations in wastewater
AIMS MATHEMATICS(2023)
Abstract
Wastewater sampling for the detection and monitoring of SARS-CoV-2 has been developed and applied at an unprecedented pace, however uncertainty remains when interpreting the measured viral RNA signals and their spatiotemporal variation. The proliferation of measurements that are below a quantifiable threshold, usually during non-endemic periods, poses a further challenge to interpretation and time-series analysis of the data. Inspired by research in the use of a custom Kalman smoother model to estimate the true level of SARS-CoV-2 RNA concentrations in wastewater, we propose an alternative left-censored dynamic linear model. Cross-validation of both models alongside a simple moving average, using data from 286 sewage treatment works across England, allows for a comprehensive validation of the proposed approach. The presented dynamic linear model is more parsimonious, has a faster computational time and is represented by a more flexible modelling framework than the equivalent Kalman smoother. Furthermore we show how the use of wastewater data, transformed by such models, correlates more closely with regional case rate positivity as published by the Office for National Statistics (ONS) Coronavirus (COVID-19) Infection Survey. The modelled output is more robust and is therefore capable of better complementing traditional surveillance than untransformed data or a simple moving average, providing additional confidence and utility for public health decision making.
MoreTranslated text
Key words
dynamic linear model,wastewater-based epidemiology,COVID-19,time series,left-censoring,Bayesian inference
AI Read Science
Must-Reading Tree
Example
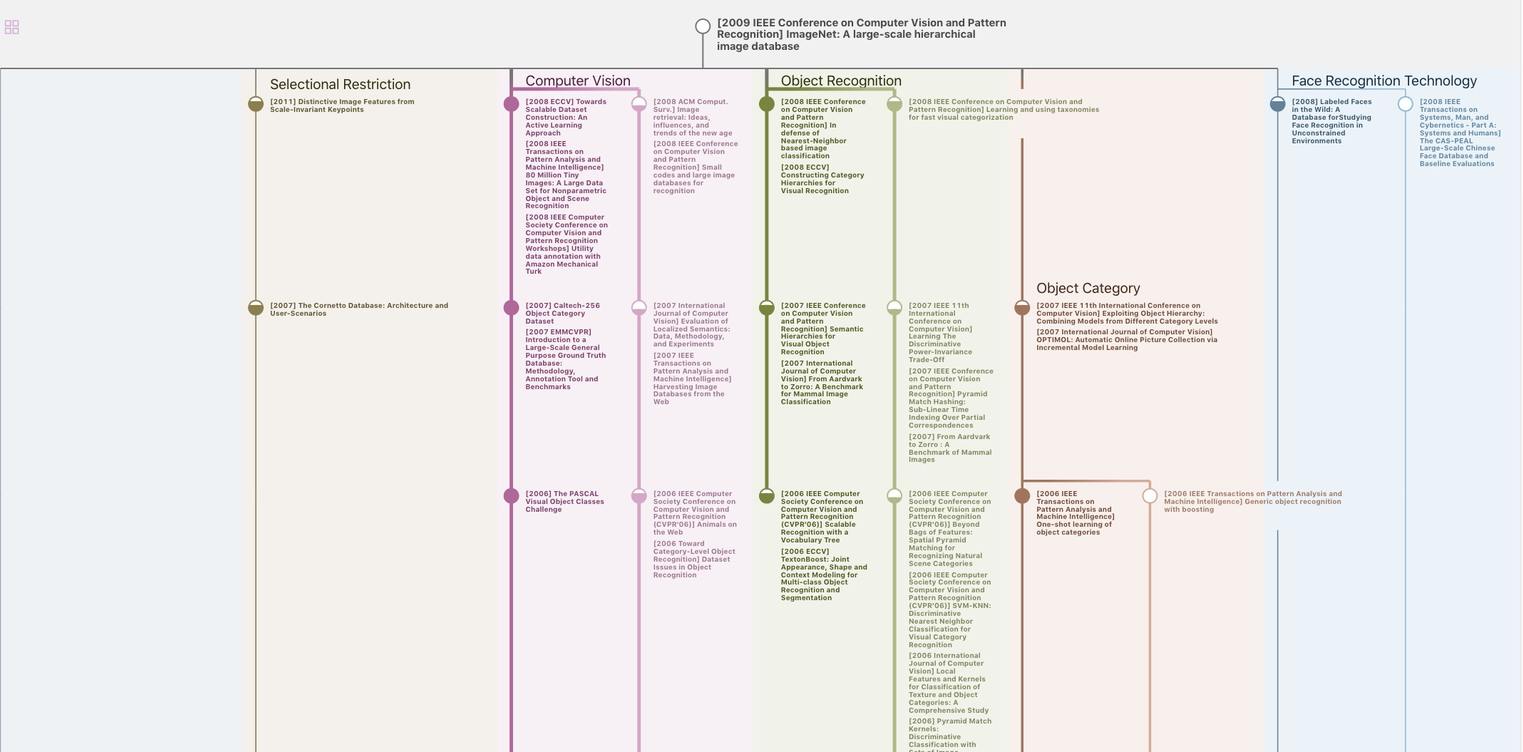
Generate MRT to find the research sequence of this paper
Chat Paper
Summary is being generated by the instructions you defined