Real-Time sEMG Pattern Recognition of Multiple-Mode Movements for Artificial Limbs Based on CNN-RNN Algorithm
ELECTRONICS(2023)
摘要
Currently, sEMG-based pattern recognition is a crucial and promising control method for prosthetic limbs. A 1D convolutional recurrent neural network classification model for recognizing online finger and wrist movements in real time was proposed to address the issue that the classification recognition rate and time delay cannot be considered simultaneously. This model could effectively combine the advantages of the convolutional neural network and recurrent neural network. Offline experiments were used to verify the recognition performance of 20 movements, and a comparative analysis was conducted with CNN and LSTM classification models. Online experiments via the self-developed sEMG signal pattern recognition system were established to examine real-time recognition performance and time delay. Experiment results demonstrated that the average recognition accuracy of the 1D-CNN-RNN classification model achieved 98.96% in offline recognition, which is significantly higher than that of the CNN and LSTM (85.43% and 96.88%, respectively, p < 0.01). In the online experiments, the average accuracy of the real-time recognition of the 1D-CNN-RNN reaches 91% +/- 5%, and the average delay reaches 153 ms. The proposed 1D-CNN-RNN classification model illustrates higher performances in real-time recognition accuracy and shorter time delay with no obvious sense of delay in the human body, which is expected to be an efficient control for dexterous prostheses.
更多查看译文
关键词
artificial limbs,pattern recognition,real-time,multiple-mode,cnn-rnn
AI 理解论文
溯源树
样例
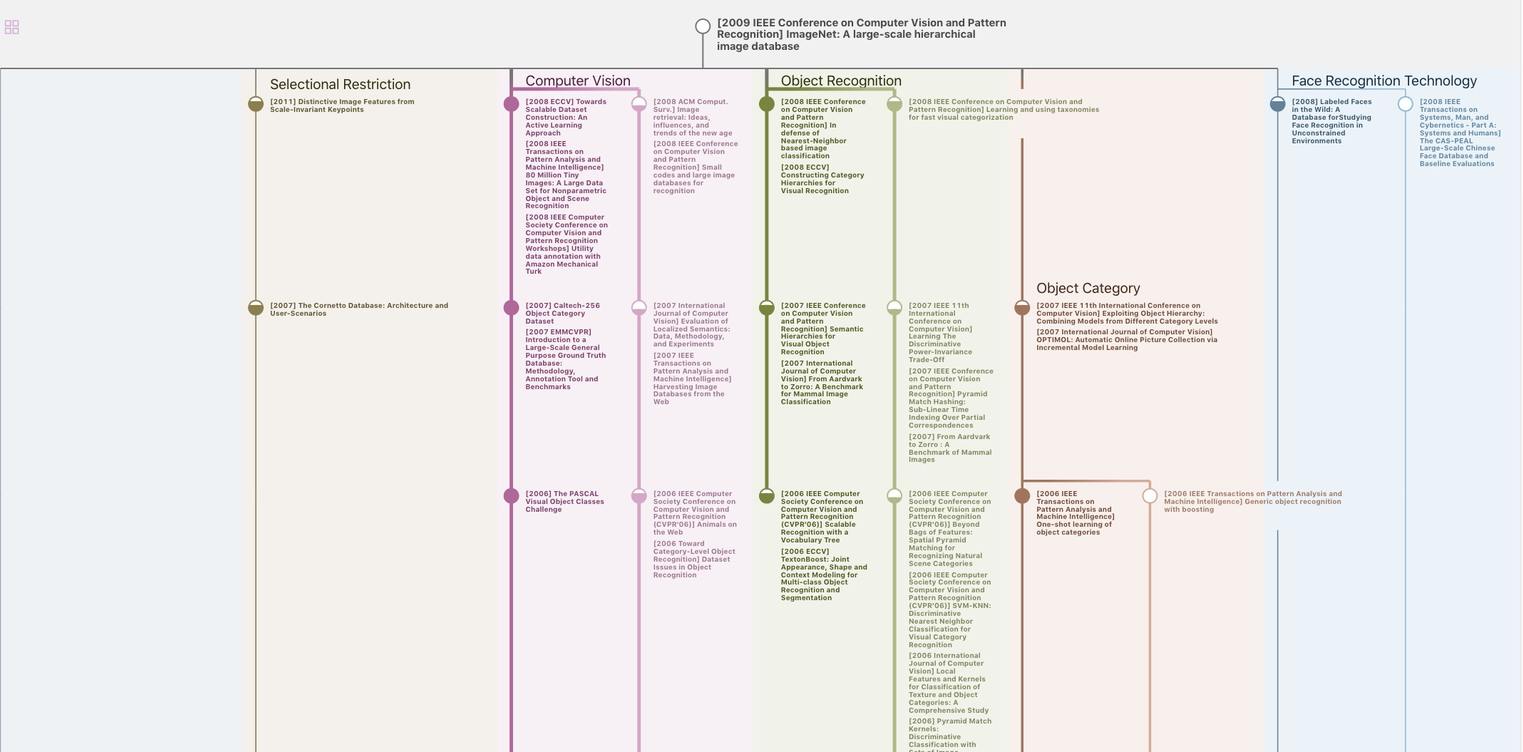
生成溯源树,研究论文发展脉络
Chat Paper
正在生成论文摘要