Better Safe Than Never: A Survey on Adversarial Machine Learning Applications towards IoT Environment
APPLIED SCIENCES-BASEL(2023)
摘要
Internet of Things (IoT) technologies serve as a backbone of cutting-edge intelligent systems. Machine Learning (ML) paradigms have been adopted within IoT environments to exploit their capabilities to mine complex patterns. Despite the reported promising results, ML-based solutions exhibit several security vulnerabilities and threats. Specifically, Adversarial Machine Learning (AML) attacks can drastically impact the performance of ML models. It also represents a promising research field that typically promotes novel techniques to generate and/or defend against Adversarial Examples (AE) attacks. In this work, a comprehensive survey on AML attack and defense techniques is conducted for the years 2018-2022. The article investigates the employment of AML techniques to enhance intrusion detection performance within the IoT context. Additionally, it depicts relevant challenges that researchers aim to overcome to implement proper IoT-based security solutions. Thus, this survey aims to contribute to the literature by investigating the application of AML concepts within the IoT context. An extensive review of the current research trends of AML within IoT networks is presented. A conclusion is reached where several findings are reported including a shortage of defense mechanisms investigations, a lack of tailored IoT-based solutions, and the applicability of the existing mechanisms in both attack and defense scenarios.
更多查看译文
关键词
adversarial machine learning applications,iot,machine learning,safe
AI 理解论文
溯源树
样例
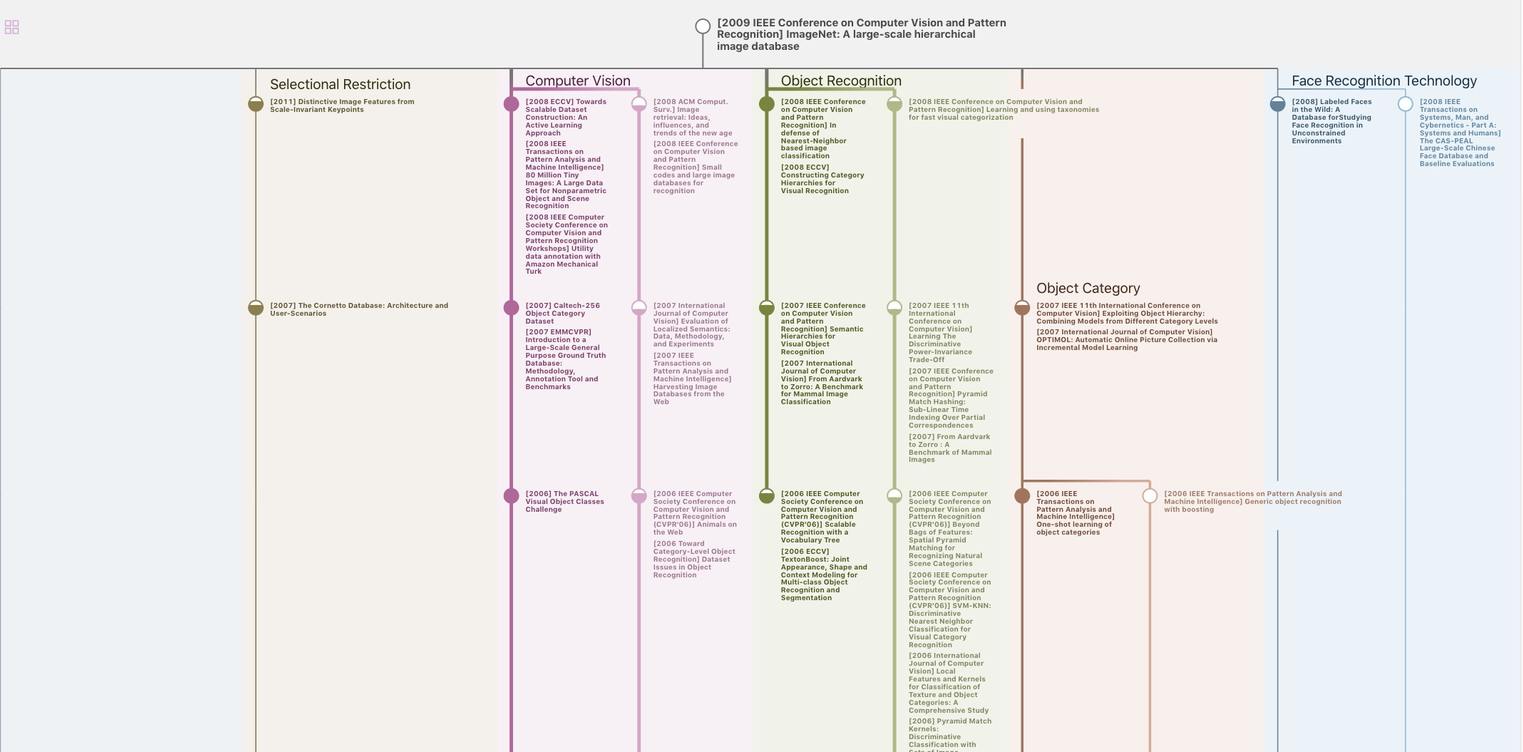
生成溯源树,研究论文发展脉络
Chat Paper
正在生成论文摘要