A novel fairness-aware ensemble model based on hybrid sampling and modified two-layer stacking for fair classification
INTERNATIONAL JOURNAL OF MACHINE LEARNING AND CYBERNETICS(2023)
摘要
Fair classification is an important field in machine learning, especially in ensemble learning research. However, traditional machine learning methods neither consider the bias in datasets nor the unfairness while training the ensemble model. Therefore, in this paper, a novel fairness-aware ensemble model (FAEM) based on hybrid sampling and modified two-layer stacking is proposed to achieve more equitable predictive performance. To reduce the bias caused by the imbalanced dataset, a new hybrid sampling-based bias-alleviation method is proposed, which removes majority samples through cross-validation-based under-sampling and adds generated minority samples through sensitive attribute-based over-sampling. The fairness of the proposed FAEM is further improved by the proposed new two-layer stacking-based fairness-aware ensemble learning method, which modifies the individual prediction results of the base classifiers in the first layer of stacking to alleviate the bias. Four datasets and five evaluation metrics were used to evaluate the classification performance and fairness of the model. The experiment results show that the proposed FAEM can effectively trade off accuracy for fairness and is 54.8% better than benchmark models in fairness metrics in average.
更多查看译文
关键词
Fairness-aware ensemble,Hybrid sampling,Machine learning,Stacking
AI 理解论文
溯源树
样例
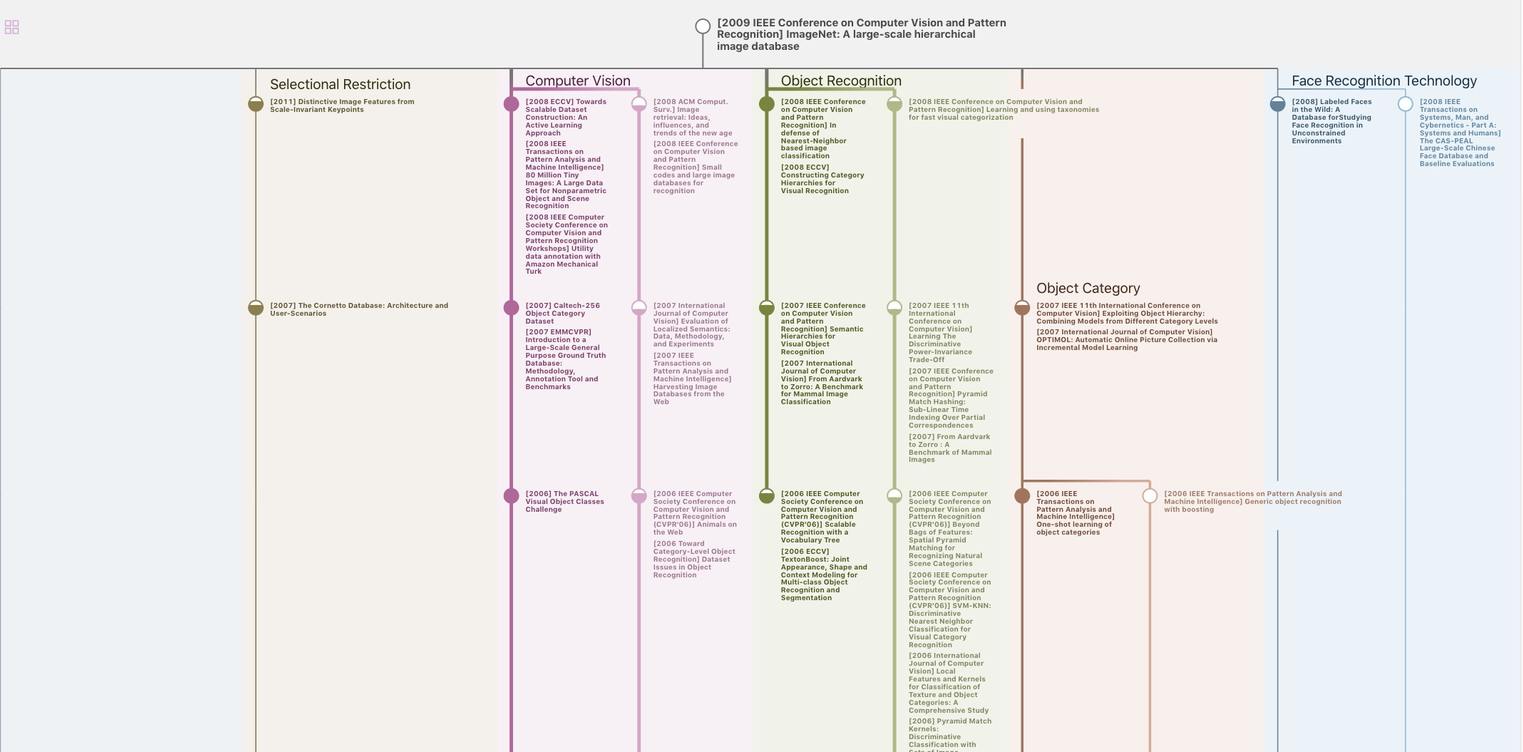
生成溯源树,研究论文发展脉络
Chat Paper
正在生成论文摘要