Vehicle Re-Identification by Separating Representative Spatial Features
COGNITIVE COMPUTATION(2023)
摘要
As a complex image classification problem, re-identification (ReID) requires the model to capture diverse representative features of vehicles through different spatial orientation cameras. However, it has been observed that the existing models tend to focus on extracting features with strong discrimination, while ignoring other valuable spatial features. In addition, the existing methods lack effective suppression of noise caused by spatial variations. Inspired by the observation from the human cognition that, the view and direction of the vehicle can be correctly recognized by human beings with only partial representative spatial features observed, in this paper, we propose a novel method to effectively separate representative spatial (SRS) information and non-spatial region discriminative information of vehicles. First, we specifically use an effective network to extract the vehicle keypoint information (e.g., roof and left wheel), and capture the representative local spatial region via the keypoint information. Then, we use the representative spatial features in the local spatial region and the distinguishing discriminative features in the non-spatial region to eliminate the interference arising from the spatial shift while enhancing the robustness of the model. Finally, the global discriminative information and representative spatial information are combined for vehicle re-identification to enhance the performance of the model. We validate the effectiveness of our proposed approach on the vehicle ReID datasets (VehicleID, VeRi-776 and VeRi-Wild). Experimental results show that our method achieves state-of-the-art performance.
更多查看译文
关键词
separating representative spatial features,vehicle,re-identification
AI 理解论文
溯源树
样例
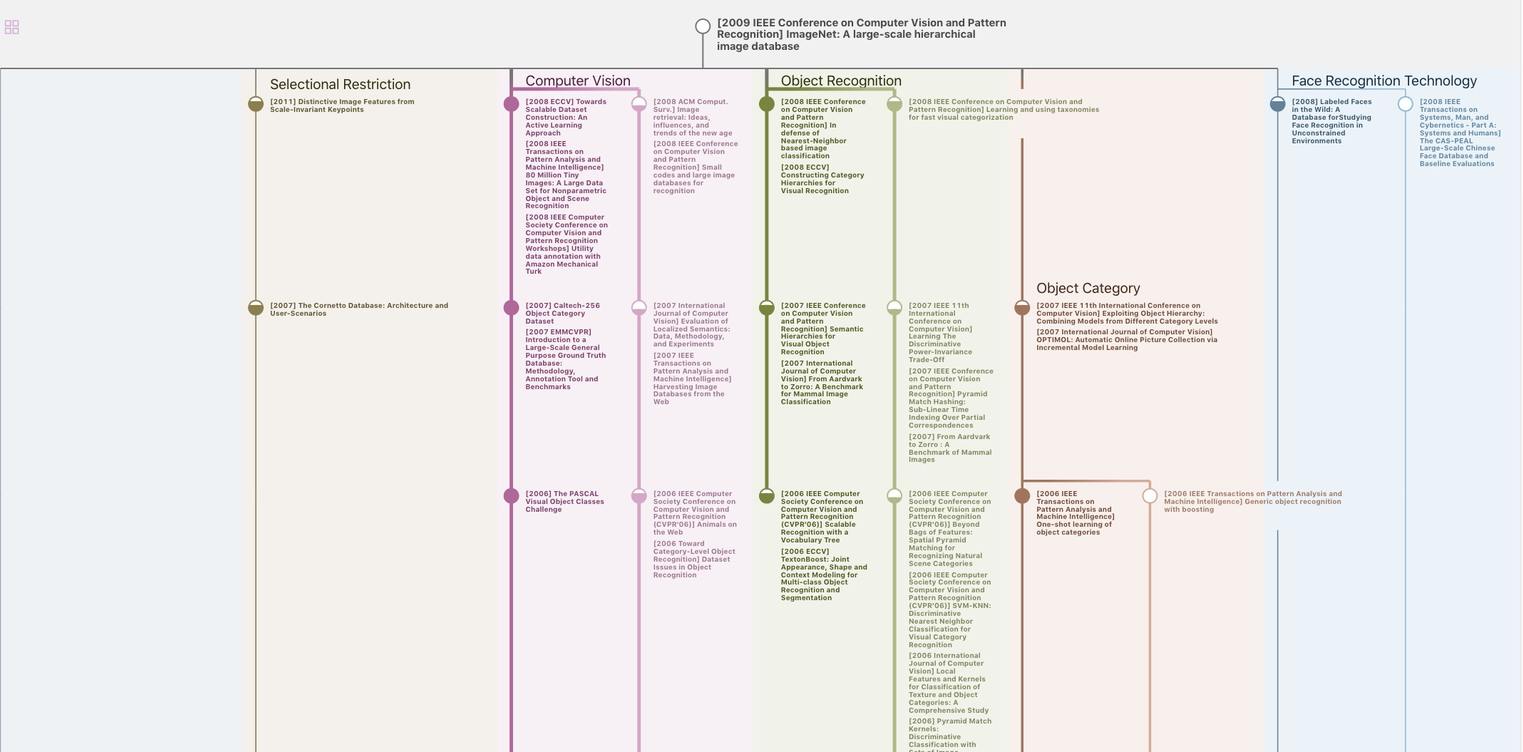
生成溯源树,研究论文发展脉络
Chat Paper
正在生成论文摘要