A survey of class-imbalanced semi-supervised learning
MACHINE LEARNING(2023)
Abstract
Semi-supervised learning(SSL) can substantially improve the performance of deep neural networks by utilizing unlabeled data when labeled data is scarce. The state-of-the-art(SOTA) semi-supervised algorithms implicitly assume that the class distribution of labeled datasets and unlabeled datasets are balanced, which means the different classes have the same numbers of training samples. However, they can hardly perform well on minority classes when the class distribution of training data is imbalanced. Recent work has found several ways to decrease the degeneration of semi-supervised learning models in class-imbalanced learning. In this article, we comprehensively review class-imbalanced semi-supervised learning (CISSL), starting with an introduction to this field, followed by a realistic evaluation of existing class-imbalanced semi-supervised learning algorithms and a brief summary of them.
MoreTranslated text
Key words
Deep learning,Class-imbalanced supervised learning,Semi-supervised learning,Class-imbalanced semi-supervised learning
AI Read Science
Must-Reading Tree
Example
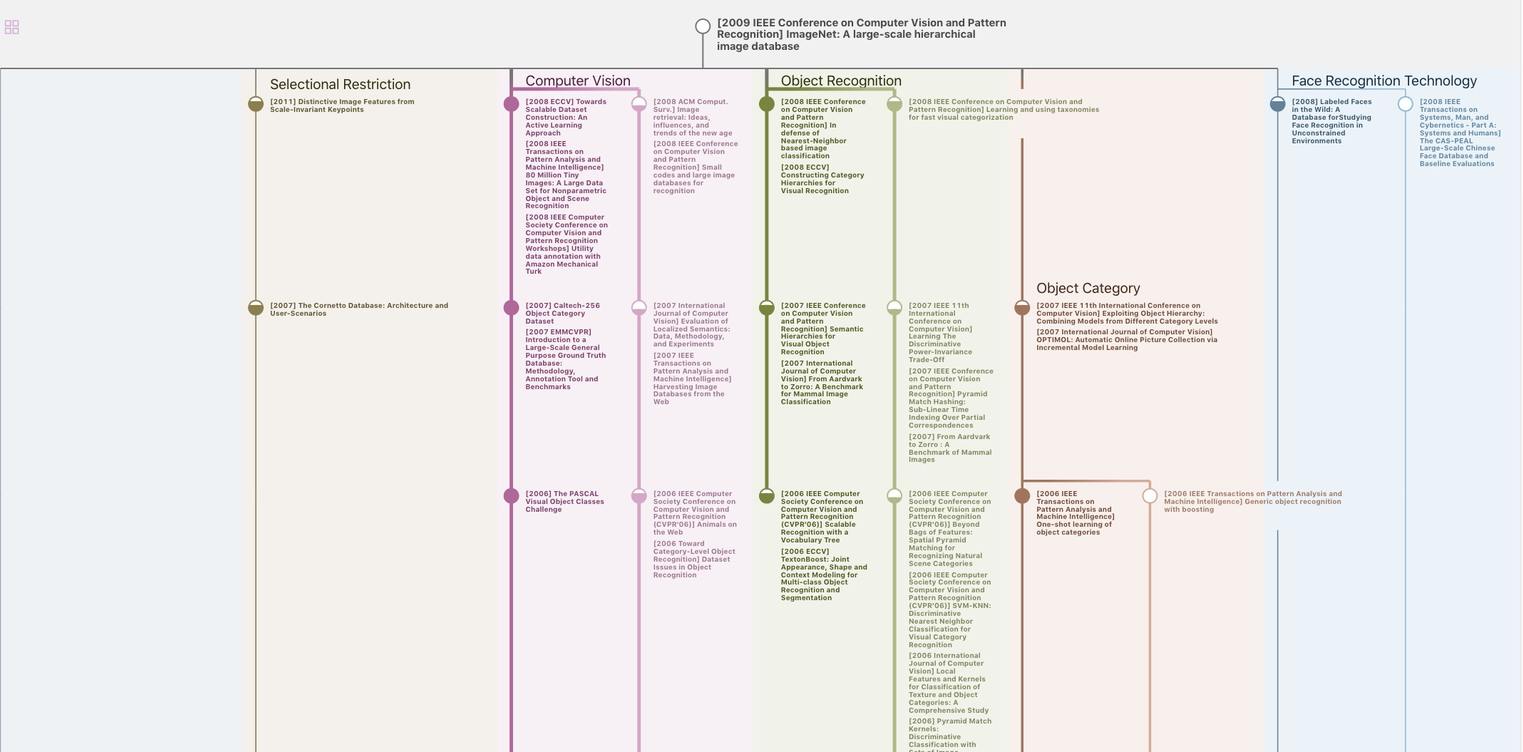
Generate MRT to find the research sequence of this paper
Chat Paper
Summary is being generated by the instructions you defined