Self-attention reinforcement learning for multi-beam combining in mmWave 3D-MIMO systems
SCIENCE CHINA-INFORMATION SCIENCES(2023)
摘要
Machine learning (ML) has been empowering all aspects of the wireless communication system design, among which, the reinforcement learning (RL)-based approaches have attracted a lot of research attention since they can interact with the environment directly and learn from the collected experiences efficiently. In this paper, we propose a novel and efficient RL-based multi-beam combining scheme for future millimeter-wave (mmWave) three-dimensional (3D) multi-input multi-output (MIMO) communication systems. The proposed scheme does not require perfect channel state information (CSI) or precise user location information which both are generally difficult to obtain in practice, and well addresses the crucial challenge of computational complexity incurred by the extremely huge state and action spaces associated with multiple users, multiple paths, and multiple 3D beams. In particular, a self-attention deep deterministic policy gradient (DDPG)-based beam selection and combination framework is proposed to learn the 3D beamforming pattern without CSI adaptively. We aim to maximize the sum-rate of the mmWave 3D-MIMO system by optimizing the serving beam set and the corresponding combining weights for each user. To this end, the transformer is incorporated into the DDPG to obtain the global information of the input elements and capture the signal directions precisely, which leads to a near-optimal beamformer design. Simulation results verify the superiority of the proposed self-attention DDPG over conventional ML-based beamforming schemes in terms of sum-rate under various scenarios.
更多查看译文
关键词
reinforcement learning (RL),deep deterministic policy gradient (DDPG),self-attention,pre-coding,combining,millimeter-wave (mmWave),multi-input multi-output (MIMO)
AI 理解论文
溯源树
样例
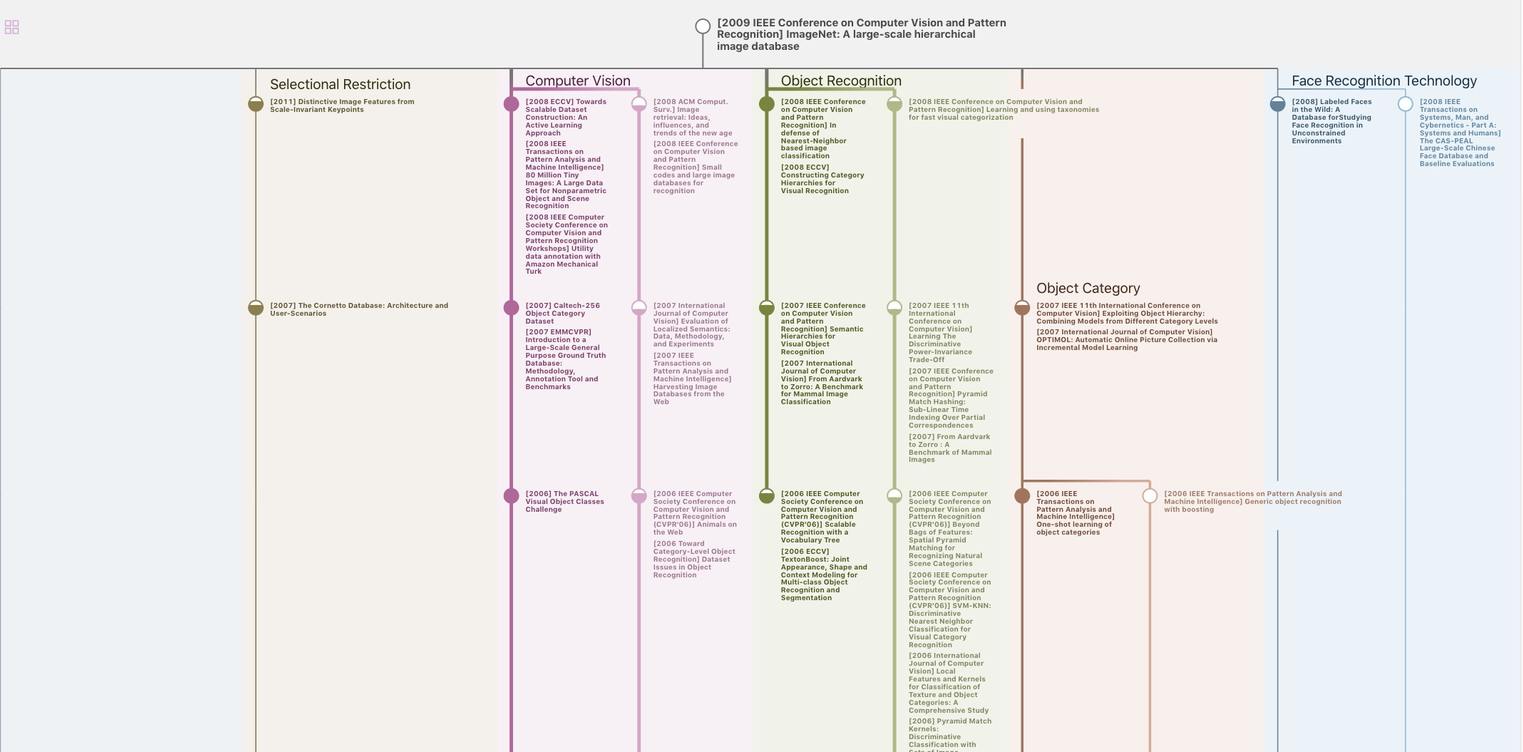
生成溯源树,研究论文发展脉络
Chat Paper
正在生成论文摘要