A spindle thermal error modeling based on 1DCNN-GRU-Attention architecture under controlled ambient temperature and active cooling
INTERNATIONAL JOURNAL OF ADVANCED MANUFACTURING TECHNOLOGY(2023)
摘要
Ambient temperature control and active internal cooling can effectively reduce the impact of spindle thermal error. At the same time, the residual spindle thermal error fluctuates violently, which makes it difficult to predict the thermal error accurately. To combat this issue, experiments to identify the spindle thermal error with constant and variable spindle speed spectrums were designed. A deep learning model is proposed for thermal error prediction, wherein speed parameter is incorporated as an input variable alongside temperature variables. The proposed model adopts a 1D convolutional neural network-gated recurrent unit-attention (1DCNN-GRU-Attention) architecture. Within this model, the 1DCNN module is responsible for managing the highly collinear input data and extracting spatial information, followed by the GRU module which extracts temporal information. Furthermore, the attention mechanism of the model can re-weight the learned features. Ultimately, the regression layer is responsible for predicting thermal error. To verify the proposed model’s effectiveness, it was compared against seven other thermal error prediction models. The experimental results revealed that the convolutional module of the proposed model is highly effective and can replace the traditional temperature-sensitive point selection (TPS) method. Under multiple coupled factors, the proposed method achieved a prediction accuracy of 81.53%, with root-mean-square error (RMSE) lower than the traditional method by 40%.
更多查看译文
关键词
Spindle thermal error,Error prediction,Deep learning model,Variable speed spectrum,Feature learning
AI 理解论文
溯源树
样例
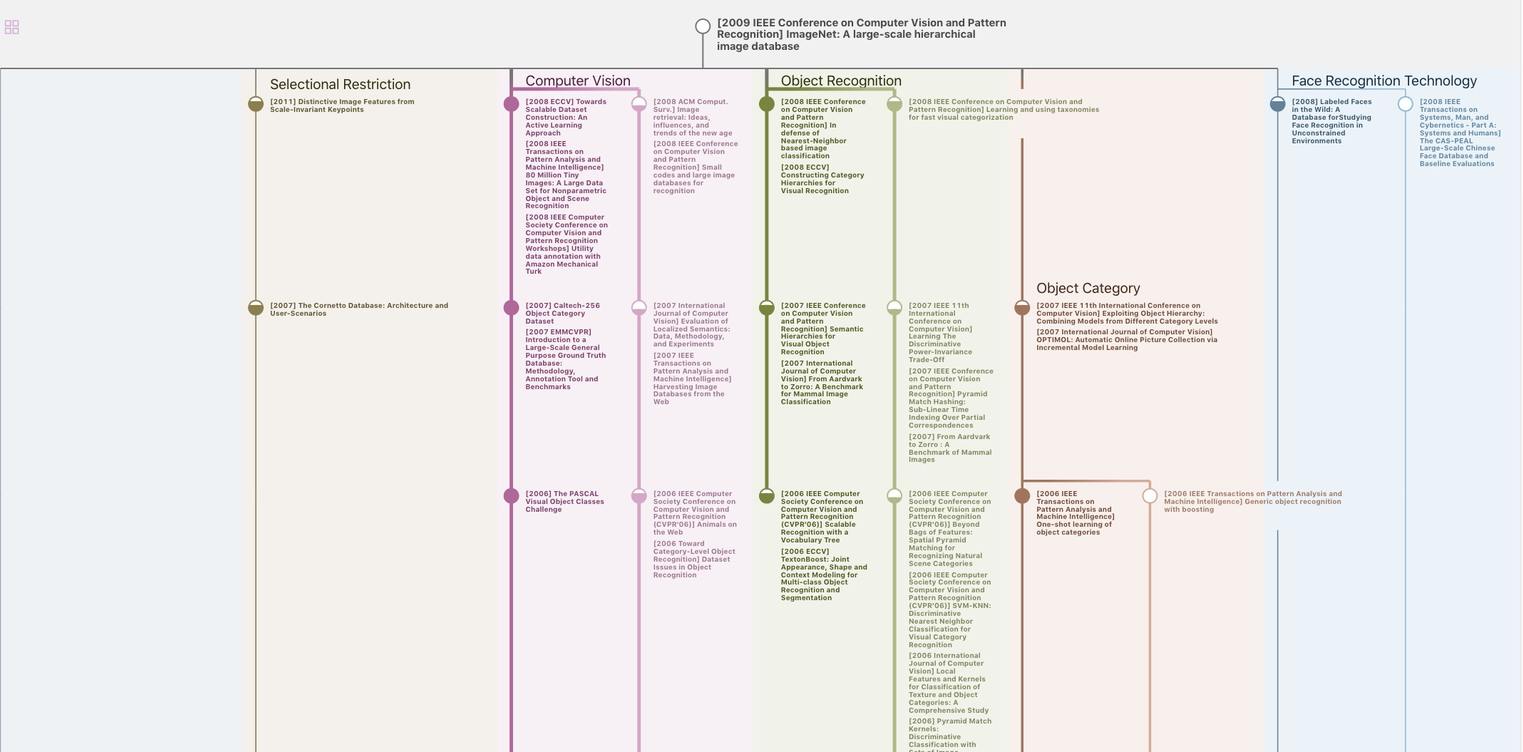
生成溯源树,研究论文发展脉络
Chat Paper
正在生成论文摘要