Global TEC Map Fusion Through a Hybrid Deep Learning Model: RFGAN
SPACE WEATHER-THE INTERNATIONAL JOURNAL OF RESEARCH AND APPLICATIONS(2023)
摘要
Timely, reliable and comprehensive global observation information is essential for space weather research. However, limited observation technology hinders the consecutive global coverage of observation data. For the integrity and continuity of the global observation data, deep learning can obtain a global Ionospheric total electron content (TEC) map by fusing multi-source TEC maps. Different from the previous methods, in the study, a deep learning hybrid model (RFGAN) based on Dual-Discriminator Conditional Generative Adversarial Network (DDcGAN) and Free-Form Image Inpainting with Gated Convolution (Deepfill v2) is proposed to fuse the Massachusetts Institute of Technology (MIT)-TEC, International Global Navigation Satellite System TEC (IGS-TEC) and altimetry satellite TEC. Throughout the RFGAN structure, we use an autoencoder model with gated convolution to inpaint the missing parts of MIT-TEC and altimetry satellite TEC. Meanwhile, DDcGAN fuses the inpainted MIT-TEC (MIT'-TEC) and IGS-TEC to get a global TEC map with high accuracy. To a certain extent, we inpainted the ocean area of MIT-TEC through RFGAN. At the same time, RFGAN keeps the consistency of RFGAN-TEC and MIT-TEC in the continent area. Our proposed deep learning hybrid model can be easily extended and widely applied to other fields of space science, especially in addressing observational data loss and multi-source data fusion.Plain Language Summary Ionospheric total electron content (TEC) maps are very important for satellite navigation, shortwave communications and space weather research. There are many TEC map data from different sources, and their TEC show significant difference. Massachusetts Institute of Technology (MIT)-TEC and altimetry satellite TEC have near-real continent and ocean observation data, respectively, and International Global Navigation Satellite System (IGS)-TEC has obtained global coverage data through extensive data processing. However, MIT-TEC has no observational data in ocean. Moreover, the observation data of the altimeter satellite TEC is limited to the ocean, and the altimeter satellite TEC is largely missing in ocean. Therefore, how to effectively fuse these data to obtain a more realistic TEC map will be a challenge. This study adopts a specially designed GAN to fuse MIT-TEC, IGS-TEC and altimetry satellite TEC by utilizing deep learning techniques such as gated convolution, Dual-Discriminator Conditional Generative Adversarial Network, etc. The finally results show that the fused TEC map achieves high accuracy in both continents and oceans.
更多查看译文
关键词
hybrid deep learning model,fusion,deep learning
AI 理解论文
溯源树
样例
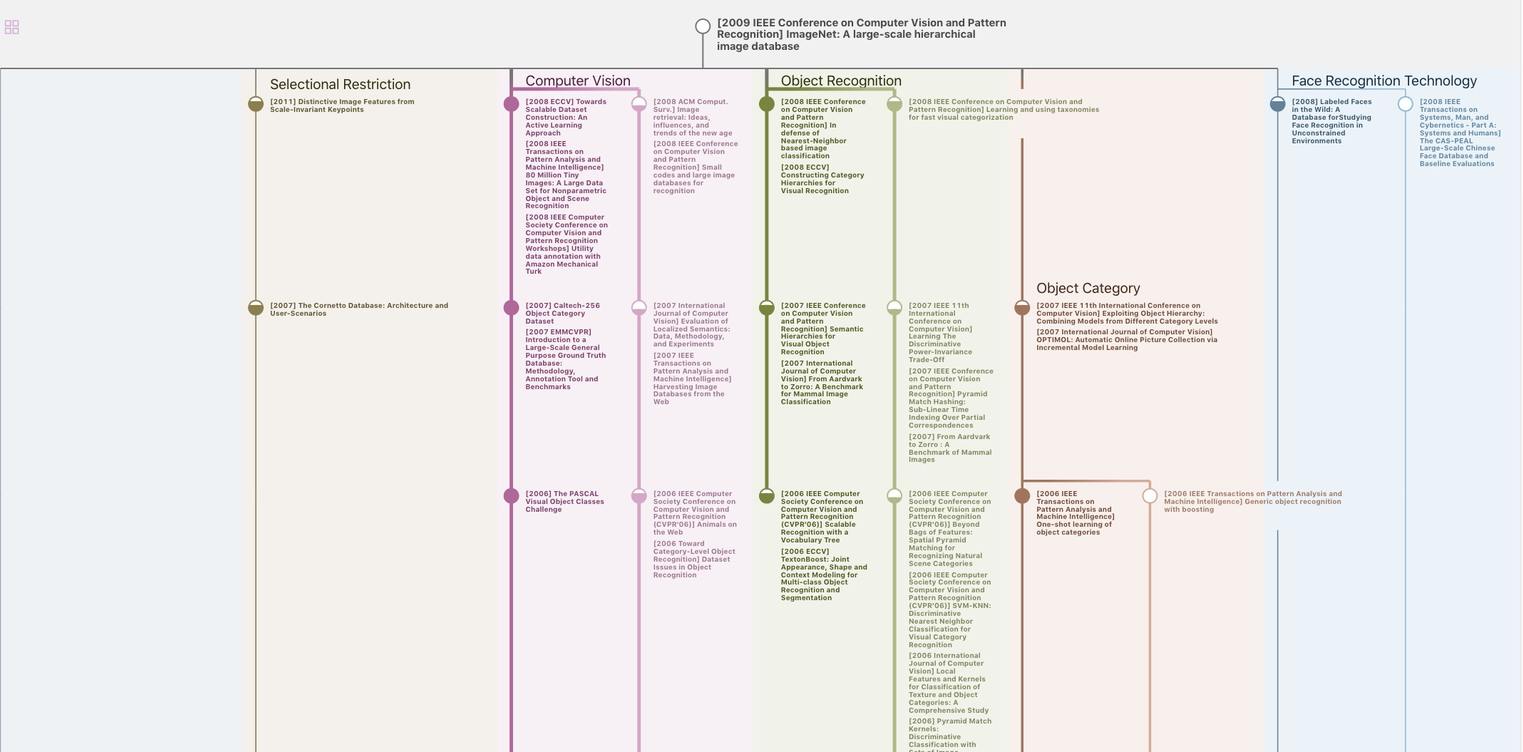
生成溯源树,研究论文发展脉络
Chat Paper
正在生成论文摘要