Establishment and validation of an AI-aid method in the diagnosis of myocardial perfusion imaging
BMC Medical Imaging(2023)
摘要
Background This study aimed to develop and validate an AI (artificial intelligence)-aid method in myocardial perfusion imaging (MPI) to differentiate ischemia in coronary artery disease. Methods We retrospectively selected 599 patients who had received gated-MPI protocol. Images were acquired using hybrid SPECT-CT systems. A training set was used to train and develop the neural network and a validation set was used to test the predictive ability of the neural network. We used a learning technique named “YOLO” to carry out the training process. We compared the predictive accuracy of AI with that of physician interpreters (beginner, inexperienced, and experienced interpreters). Results Training performance showed that the accuracy ranged from 66.20% to 94.64%, the recall rate ranged from 76.96% to 98.76%, and the average precision ranged from 80.17% to 98.15%. In the ROC analysis of the validation set, the sensitivity range was 88.9 ~ 93.8%, the specificity range was 93.0 ~ 97.6%, and the AUC range was 94.1 ~ 96.1%. In the comparison between AI and different interpreters, AI outperformed the other interpreters (most P -value < 0.05). Conclusion The AI system of our study showed excellent predictive accuracy in the diagnosis of MPI protocols, and therefore might be potentially helpful to aid radiologists in clinical practice and develop more sophisticated models.
更多查看译文
关键词
Artificial intelligence (AI), Machine learning, Coronary artery disease (CAD), Myocardial perfusion imaging (MPI), SPECT/CT
AI 理解论文
溯源树
样例
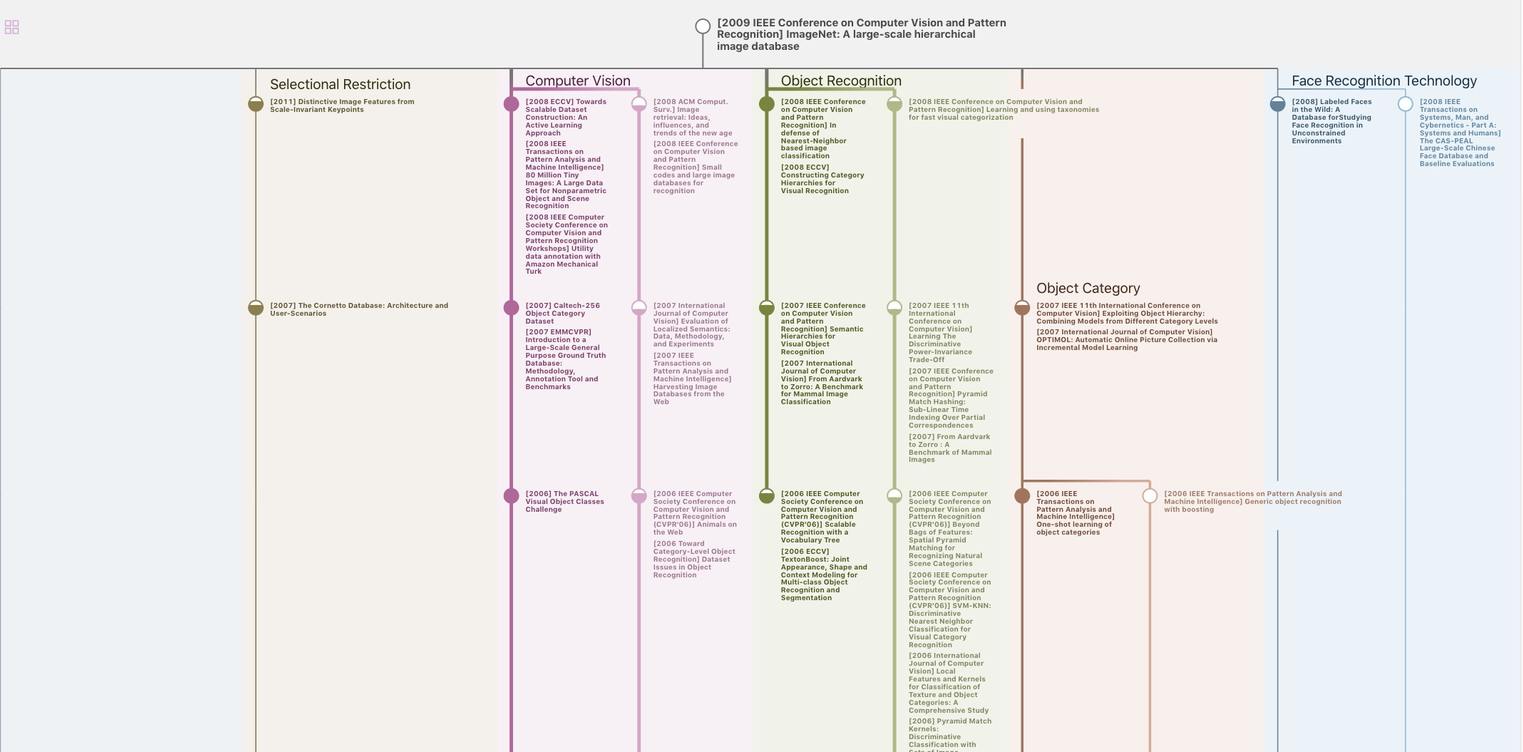
生成溯源树,研究论文发展脉络
Chat Paper
正在生成论文摘要