Sound event localization and detection using element-wise attention gate and asymmetric convolutional recurrent neural networks.
AI Commun.(2023)
摘要
There are problems that standard square convolution kernel has insufficient representation ability and recurrent neural network usually ignores the importance of different elements within an input vector in sound event localization and detection. This paper proposes an element-wise attention gate-asymmetric convolutional recurrent neural network (EleAttG-ACRNN), to improve the performance of sound event localization and detection. First, a convolutional neural network with context gating and asymmetric squeeze excitation residual is constructed, where asymmetric convolution enhances the capability of the square convolution kernel; squeeze excitation can improve the interdependence between channels; context gating can weight the important features and suppress the irrelevant features. Next, in order to improve the expressiveness of the model, we integrate the element-wise attention gate into the bidirectional gated recurrent network, which is to highlight the importance of different elements within an input vector, and further learn the temporal context information. Evaluation results using the TAU Spatial Sound Events 2019-Ambisonic dataset show the effectiveness of the proposed method, and it improves SELD performance up to 0.05 in error rate, 1.7% in F-score, 0.7 degrees in DOA error, and 4.5% in Frame recall compared to a CRNN method.
更多查看译文
关键词
Sound event localization and detection, asymmetric convolution, context gating, squeeze excitation, element-wise attention gate
AI 理解论文
溯源树
样例
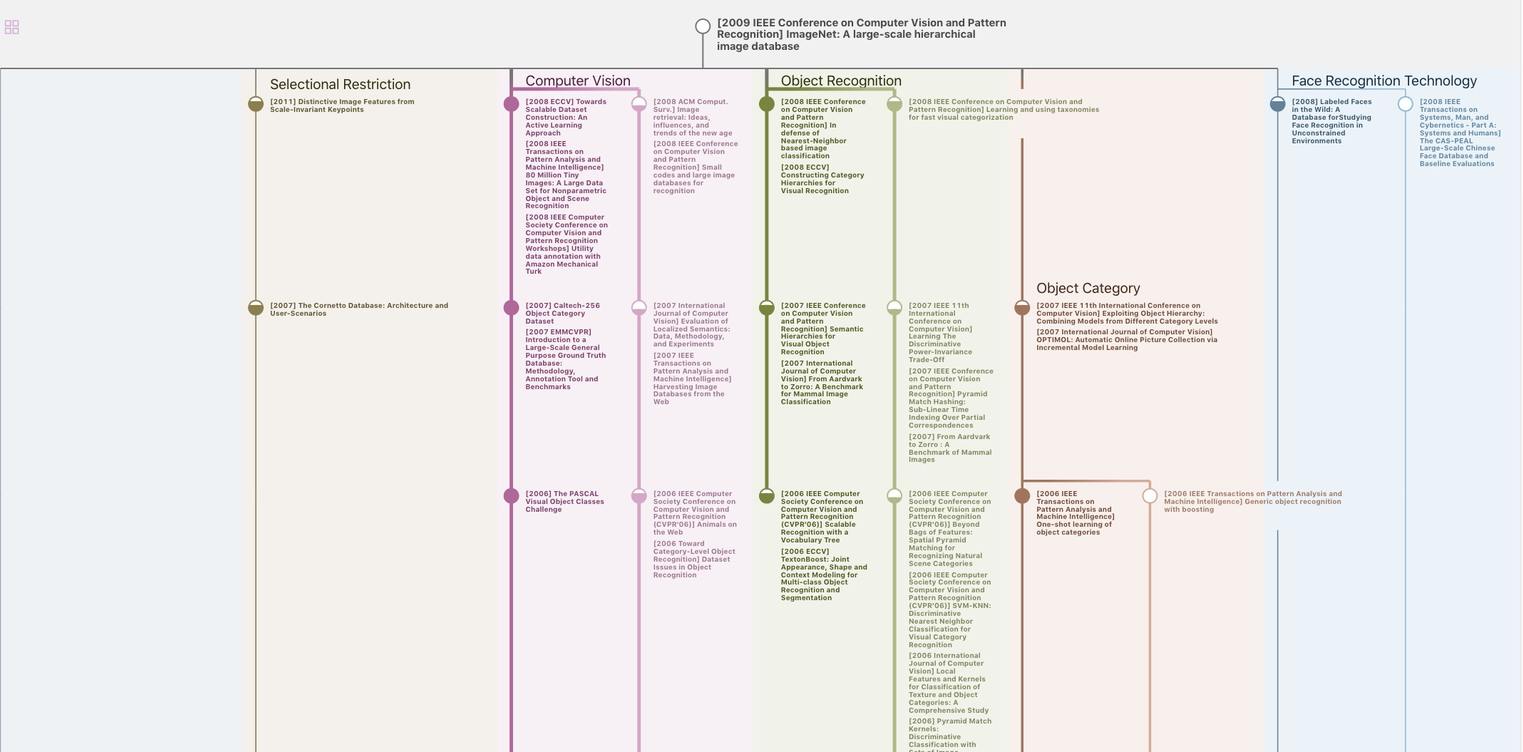
生成溯源树,研究论文发展脉络
Chat Paper
正在生成论文摘要