IoTTFID: An Incremental IoT Device Identification Model Based on Traffic Fingerprint.
IEEE Access(2023)
摘要
Driven by 5G communication technology, IoT devices are widely deployed in various scenarios to provide automated services. However, a large number of IoT devices cannot install strong encryption suites and become the preferred target of cyber attackers. Specific vulnerabilities target specific types of IoT devices. Screening and repairing corresponding vulnerabilities based on device information can improve device protection capabilities. Traditional device identification models are static and have limitations in the identification range. The model needs to be trained from scratch to identity new types of devices, which consumes a lot of computing resources and training time. To overcome these limitations, we propose IoTTFID, an incremental IoT device identification model based on traffic fingerprint. Extract the traffic fingerprint of the new device, convert it into an input vector after preprocessing, and input it to the original model to update some network parameters, so that the model has the ability to identify new devices. The results of evaluation on two open datasets show that the accuracy of IoTTFID is 98.09% on UNSW dataset and 98.29% on Yourthings dataset, which outperforms the existing methods. IoTTFID has an accuracy rate of 80.4% after five incremental learning stages, and an F1 of over 96% for encrypted IoT devices. IoTTFID can dynamically adjust with the actual environment to increase the range of identifiable device types, providing strong support for the security management of IoT devices.
更多查看译文
关键词
traffic fingerprint,iottfid,iottfid,identification
AI 理解论文
溯源树
样例
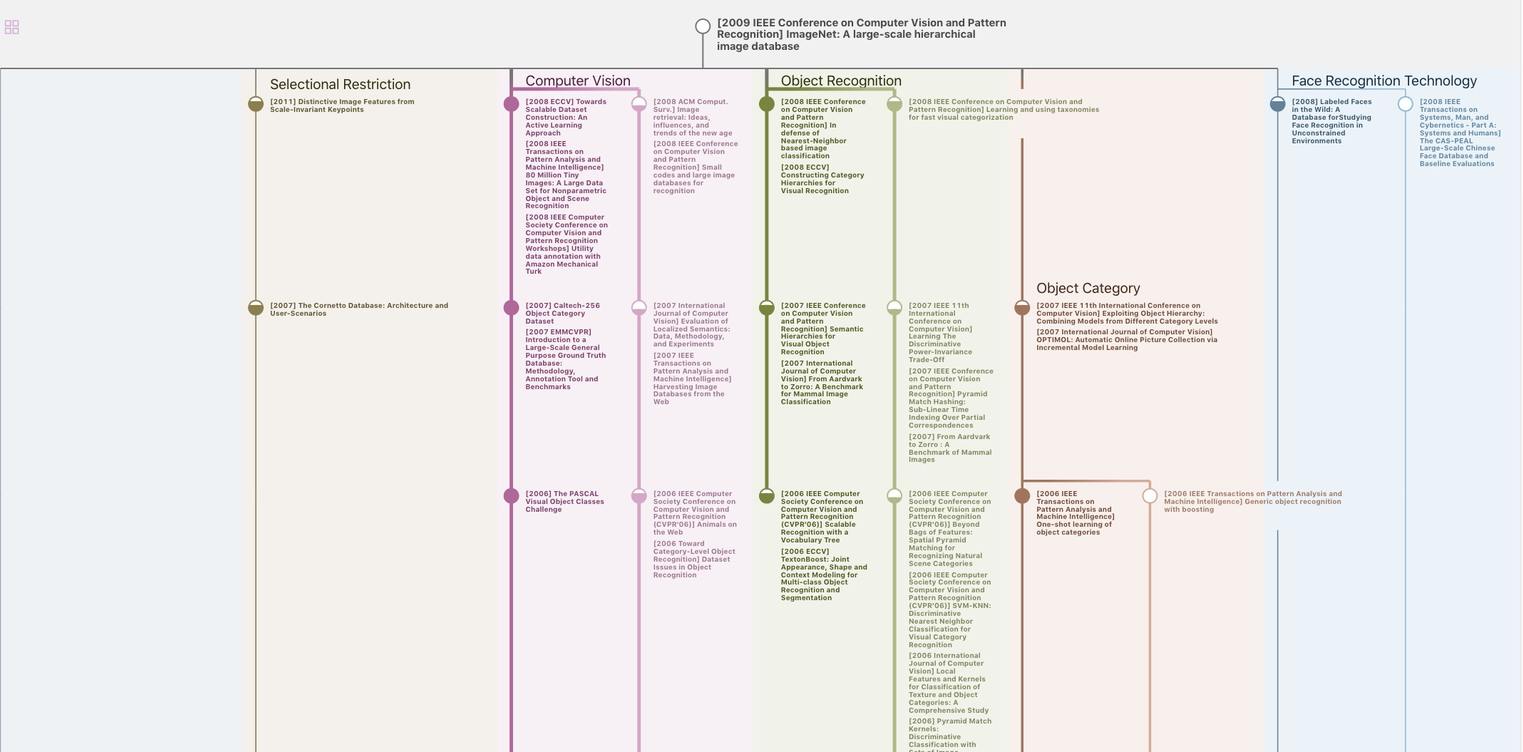
生成溯源树,研究论文发展脉络
Chat Paper
正在生成论文摘要