EnGauge: Engagement Gauge of Meeting Participants Estimated by Facial Expression and Deep Neural Network
IEEE Access(2023)
摘要
Measuring the level of engagement among participants in a meeting is crucial for evaluating collective understanding. While previous studies have utilized multiple sensors, such as wearable devices, to gauge engagement levels in offline environments, the shift to remote meetings due to the COVID-19 pandemic presents new challenges. In this study, we propose a method for measuring student engagement during online meetings using only the built-in web cameras on their devices. We collect high, middle, and low engagement level recording data from 24 students. We decided to collect data using the role-acting approach instead of conventional self-reporting or post-experiment annotation. With the feature extraction based approach, we achieved a classification rate of 46.7%. With a deep learning based approach, we achieved a classification rate of 89.5% using MobileNetV2 for leave-one-participant-out cross-validation, which demonstrated higher accuracy than previous studies. From the model, we implement an application EnGauge and conduct a pilot study. The results demonstrate a new approach to data collection, an optimal engagement level recognition model, and application scenarios.
更多查看译文
关键词
Cameras,Annotations,Support vector machines,Privacy,Observers,Feature extraction,Behavioral sciences,Engagement classification,camera,affective computing,facial expression,social interaction,deep neural network
AI 理解论文
溯源树
样例
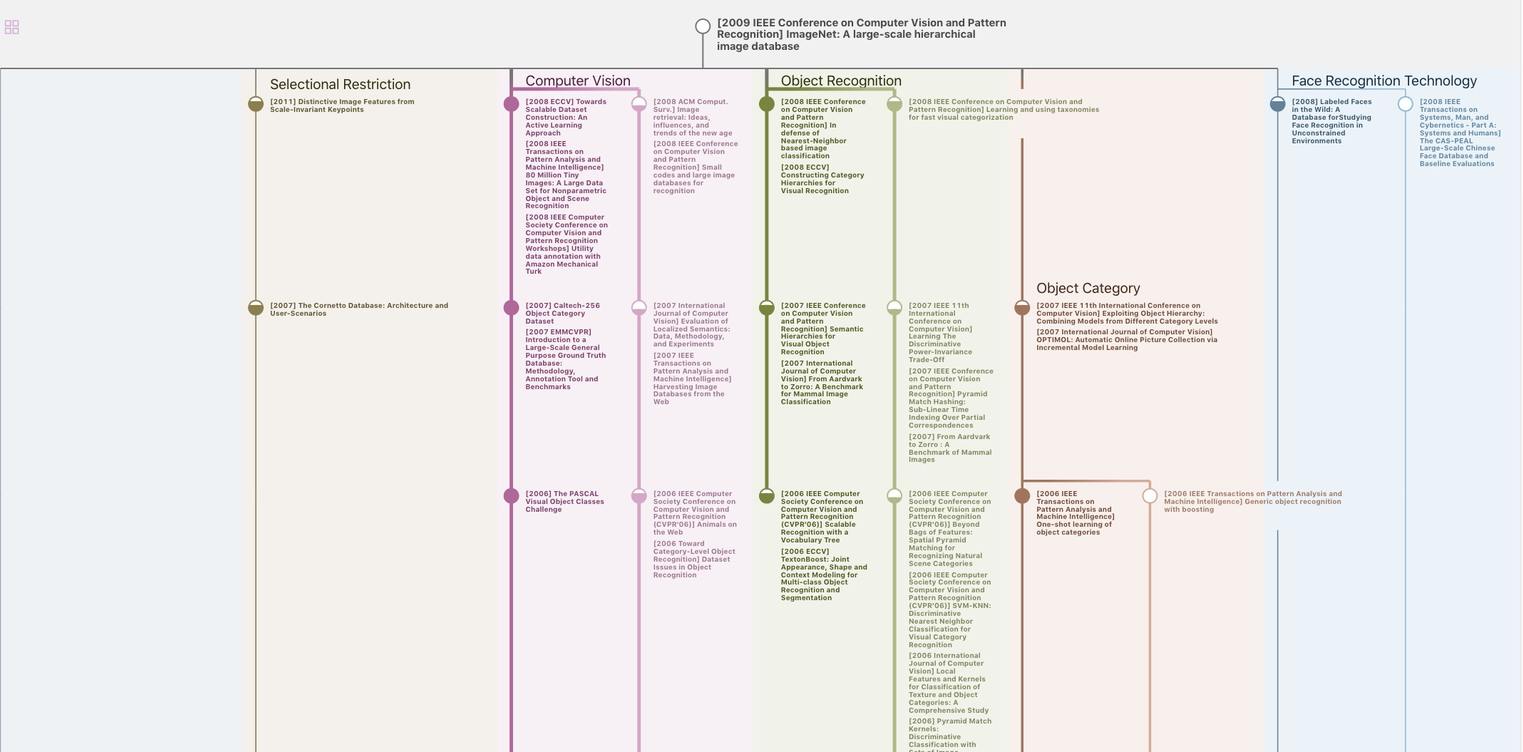
生成溯源树,研究论文发展脉络
Chat Paper
正在生成论文摘要