GPMT: Generating practical malicious traffic based on adversarial attacks with little prior knowledge.
Comput. Secur.(2023)
摘要
Machine learning (ML) is increasingly used for malicious traffic detection and proven to be effective. However, ML-based detections are at risk of being deceived by adversarial examples. It is critical to carry out adversarial attacks to evaluate the robustness of detections. Some research papers have studied adversarial attacks on ML-based detections, while most of them are in unreal scenarios. It mainly includes two aspects: (i) adversarial attacks gain extra prior knowledge about ML-based models, such as the datasets and features used by the model, which are unlikely to be available in reality; (ii) adversarial attacks generate unpractical examples, which are traffic features or traffic that doesn’t compliance with communication protocol rules.
更多查看译文
关键词
Machine learning, Malicious traffic detection, Adversarial attacks, WGAN, Black-box attacks
AI 理解论文
溯源树
样例
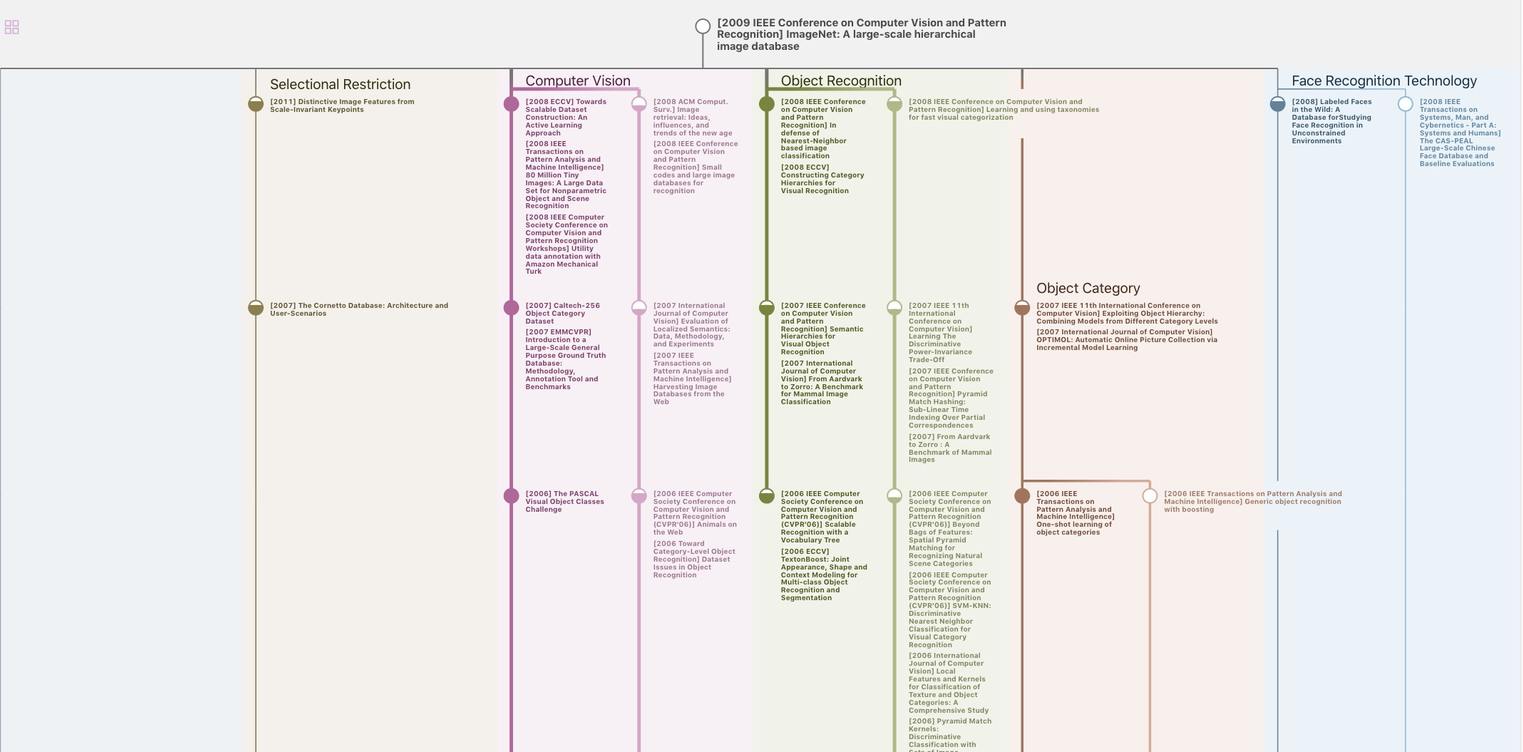
生成溯源树,研究论文发展脉络
Chat Paper
正在生成论文摘要