On the deficiency of intelligibility metrics as proxies for subjective intelligibility.
Speech Commun.(2023)
摘要
A recent trend in deep neural network (DNN)-based speech enhancement consists of using intelligibility and quality metrics as loss functions for model training with the aim of achieving high subjective speech intelligibility and perceptual quality in real-life conditions. In this study, we analyze a variety of loss functions, including some based on state-of-the-art intelligibility and quality metrics, to train an end-to-end speech enhancement system based on a fully convolutional neural network. The loss functions include perceptual metric for speech quality evaluation (PMSQE), scale-invariant signal-to-distortion ratio (SI-SDR), SI-SDR integrating speech pre-emphasis, short-time objective intelligibility (STOI), extended STOI (ESTOI), spectro-temporal glimpsing index (STGI), and a composite loss function combining STGI and SI-SDR. While DNNs trained with these loss functions produce notable speech intelligibility (and quality) gains according to pertinent objective metrics, we conduct a subjective intelligibility test that contradicts this result, showing no intelligibility improvement. From the results of this study, our conclusion is twofold: (1) subjective intelligibility evaluation is currently not replaceable by objective intelligibility evaluation, and (2) both the development of meaningful intelligibility metrics and DNN-based speech enhancement systems that can consistently improve the intelligibility of noisy speech for human listening remain open problems.
更多查看译文
关键词
Speech enhancement,Speech intelligibility,Deep learning,Loss function,Intelligibility test
AI 理解论文
溯源树
样例
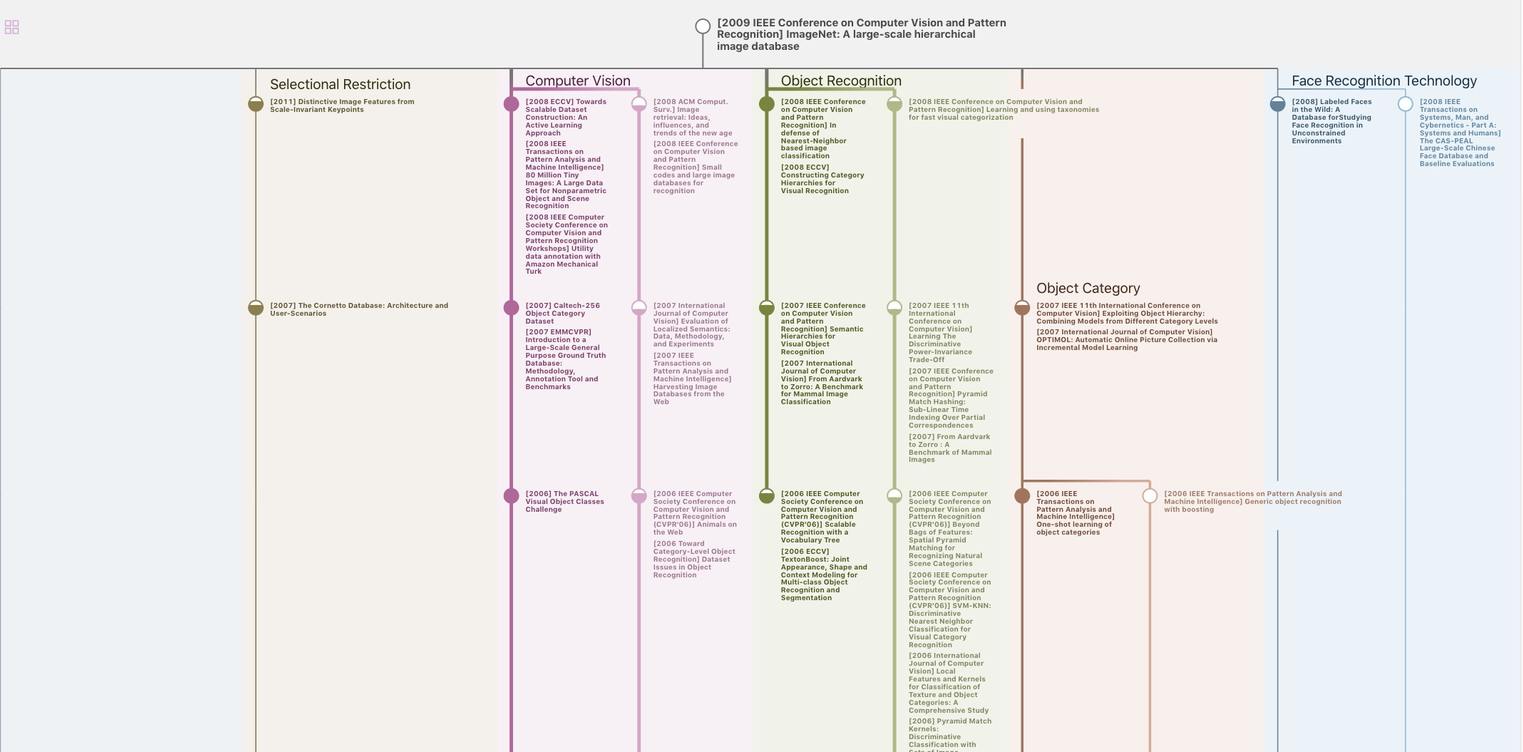
生成溯源树,研究论文发展脉络
Chat Paper
正在生成论文摘要