Dynamic threshold integrate and fire neuron model for low latency spiking neural networks
Neurocomputing(2023)
摘要
Spiking Neural Networks (SNNs) operate with asynchronous discrete events which enable lower power and greater computational efficiency on event-driven hardware than Artificial Neural Networks (ANNs). Conventional ANN-to-SNN conversion methods usually employ Integrate and Fire (IF) neuron model with a fixed threshold to act as Rectified Linear Unit (ReLU). However, there is a large demand for the input spikes to reach the fixed threshold and fire, which leads to high inference latency. In this work, we propose a Dynamic Threshold Integrate and Fire (DTIF) neuron model by exploiting the biolog-ical neuron threshold variability, where the threshold is inversely related to the neuron input. The spike activity is increased by dynamically adjusting the threshold at each simulation time-step to reduce the latency. Compared to the state-of-the-art conversion methods, the ANN-to-SNN conversion using DTIF model has lower latency with competitive accuracy, which has been verified by deep architecture on image classification tasks including MNIST, CAIFAR-10, and CIFAR-100 datasets. Moreover, it achieves 7.14 x faster inference under 0.44 x energy consumption than the typical method of maximum normalization. (c) 2023 Elsevier B.V. All rights reserved.
更多查看译文
关键词
Spiking neural networks,ANN-to-SNN conversion,Threshold variability,Image classification
AI 理解论文
溯源树
样例
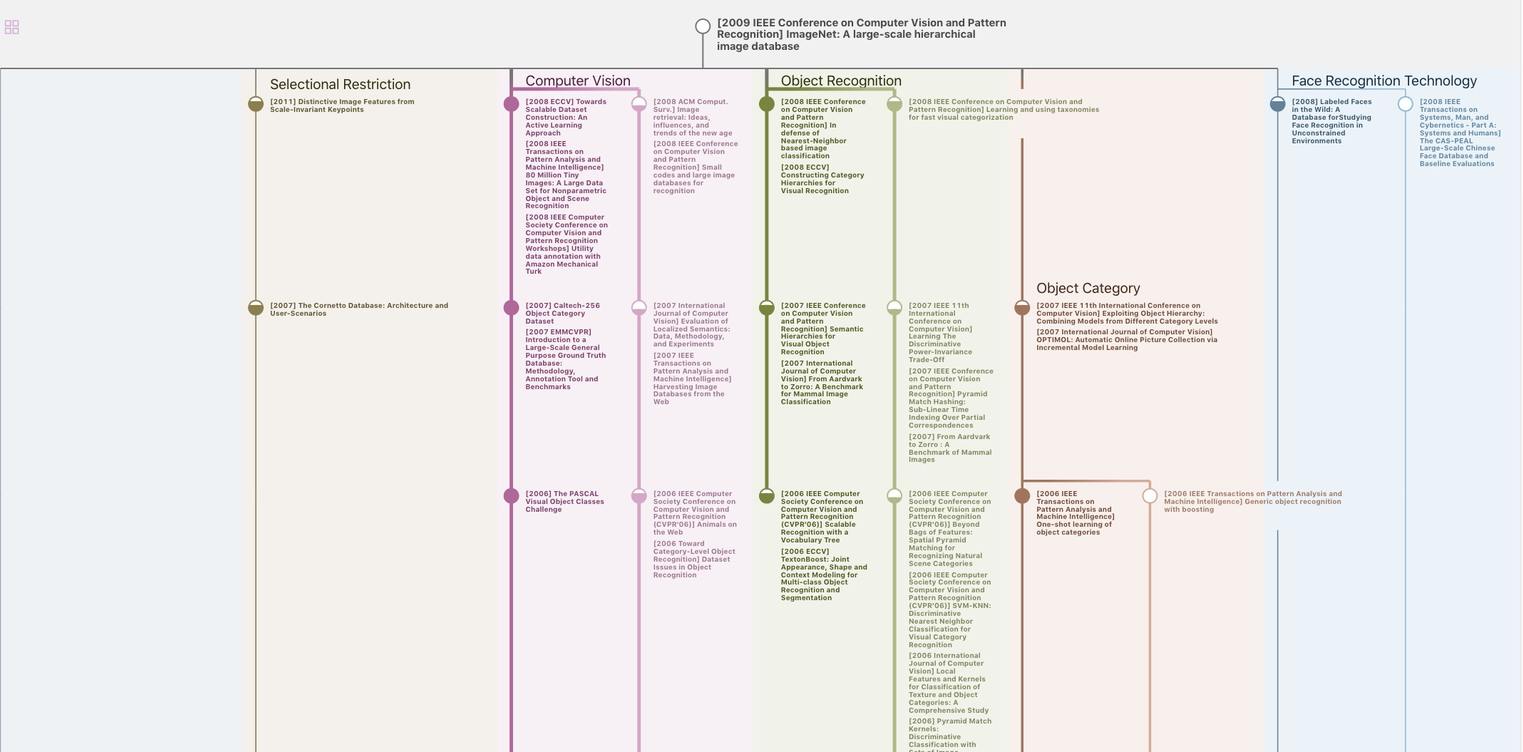
生成溯源树,研究论文发展脉络
Chat Paper
正在生成论文摘要