Stochastic DCA with Variance Reduction and Applications in Machine Learning.
J. Mach. Learn. Res.(2022)
摘要
We design stochastic Difference-of-Convex-functions Algorithms (DCA) for solving a class of structured Difference-of-Convex-functions (DC) problems. As the standard DCA re-quires the full information of (sub)gradients which could be expensive in large-scale set-tings, stochastic approaches rely upon stochastic information instead. However, stochas-tic estimations generate additional variance terms making stochastic algorithms unstable. Therefore, we integrate some novel variance reduction techniques including SVRG and SAGA into our design. The almost sure convergence to critical points of the proposed algorithms is established and the algorithms' complexities are analyzed. To study the effi-ciency of our algorithms, we apply them to three important problems in machine learning: nonnegative principal component analysis, group variable selection in multiclass logistic regression, and sparse linear regression. Numerical experiments have shown the merits of our proposed algorithms in comparison with other state-of-the-art stochastic methods for solving nonconvex large-sum problems.
更多查看译文
关键词
stochastic dca,variance reduction,machine learning
AI 理解论文
溯源树
样例
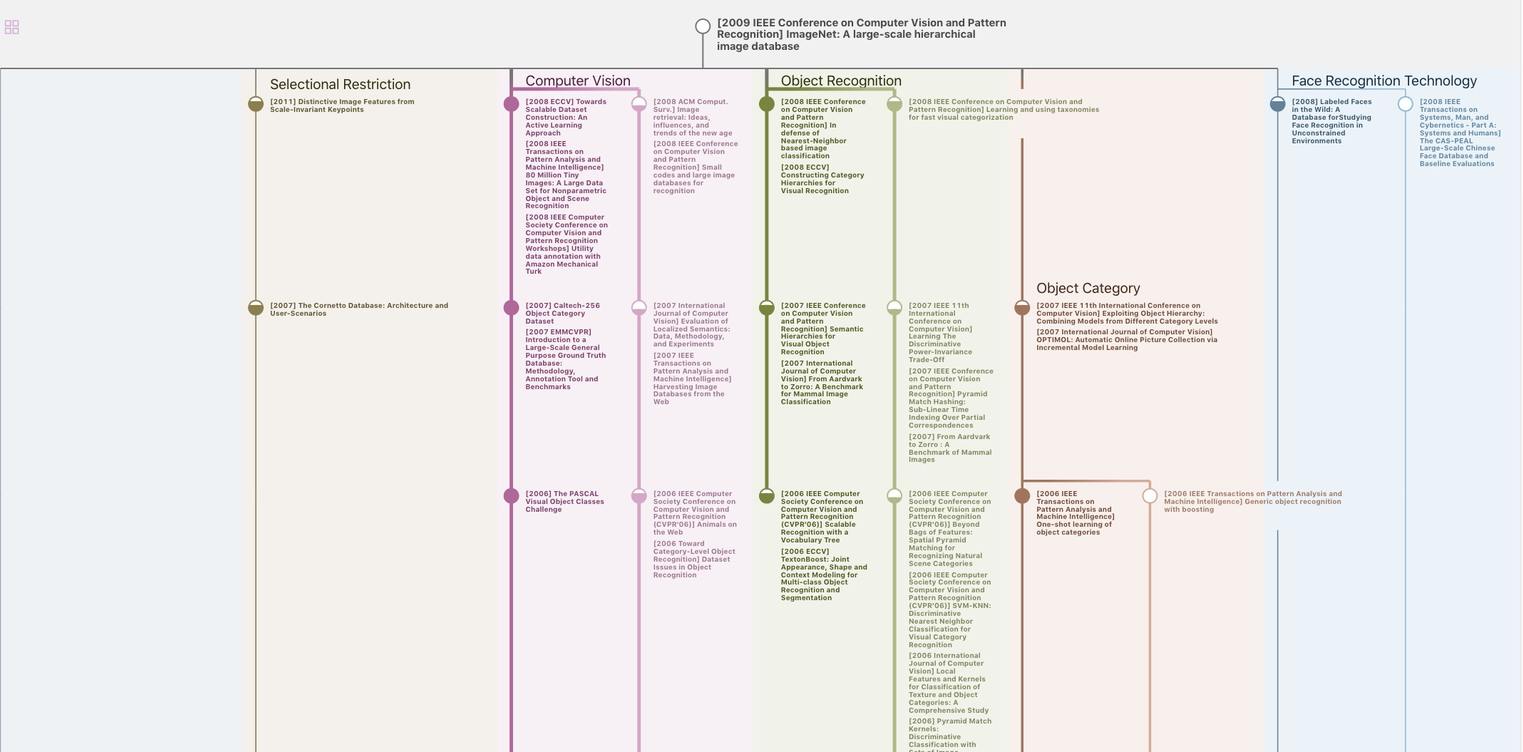
生成溯源树,研究论文发展脉络
Chat Paper
正在生成论文摘要