Multi-scale spatial-temporal attention graph convolutional networks for driver fatigue detection.
J. Vis. Commun. Image Represent.(2023)
摘要
With the development of deep learning, fatigue detection technology for drivers has achieved remarkable achievements. Although the image-based approach achieves good accuracy, it inevitably leads to greater model complexity, which is unsuitable for mobile terminal devices. Luckily, human skeletal data significantly reduces the impact of noise and input data volume while retaining valid information, and it can better deal with real-world driving scenarios with the benefit of robustness in complex driving situations. This paper proposes a lightweight multi-scale spatio-temporal attention graph convolutional network (MS-STAGCN) to efficiently utilize skeleton data to identify driver states by aggregating locally and globally valid face information, which achieves good performance even for lightweight design. The experimental results show that the method achieves 92.4% accuracy on the NTHU-DDD dataset, which can be applied to fatigue detection tasks of the driver in real-world driving scenarios in the future.
更多查看译文
关键词
41A05,41A10,65D05,65D17
AI 理解论文
溯源树
样例
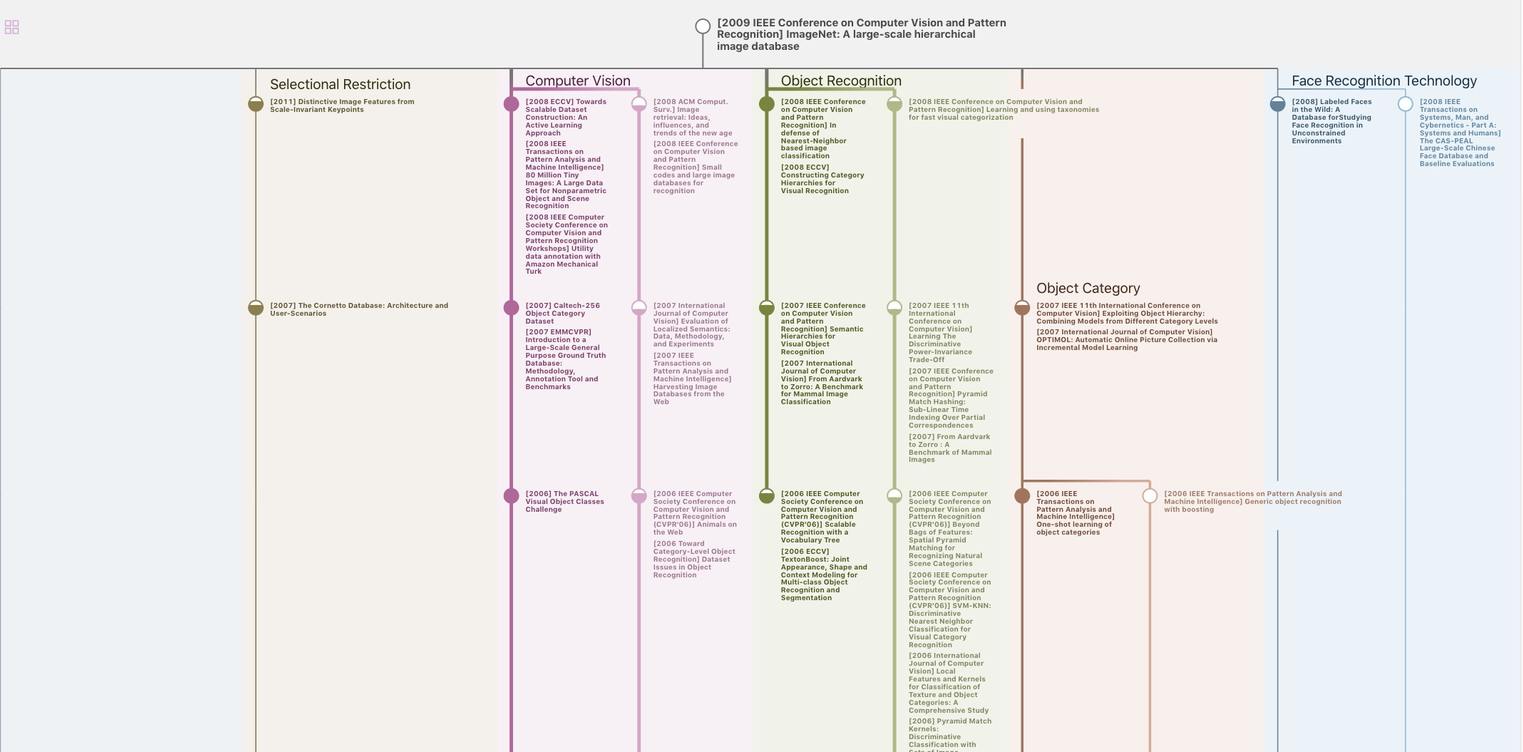
生成溯源树,研究论文发展脉络
Chat Paper
正在生成论文摘要