Energy-Based Temporal Summarized Attentive Network for Zero-Shot Action Recognition.
IEEE Trans. Multim.(2023)
摘要
Recently, Action Recognition (AR) is facing the scalability problem, since collecting and annotating data for the ever-growing action categories is exhausting and inappropriate. As an alternative to AR, Zero-Shot Action Recognition (ZSAR) is getting more and more attention in the community, as they could utilize a shared semantic/attribute space to recognize novel categories without annotated data. Different from the AR focuses on learning the correlation between actions, ZSAR needs to consider the correlation of action-action, label-label and action-label at the same time. However, as far as we know, there is no work to provide structural guidance for the framework design of ZSAR according to its task characteristics. In this paper, we demonstrate the rationality of using the Energy-Based Model (EBM) to guide the framework design of ZSAR based on their inference mechanism. Furthermore, under the guidance of EBM, we propose an Energy-based Temporal Summarized Attentive Network (ETSAN) to achieve ZSAR. Specifically, to ensure the effectiveness of cross-modal matching, EBM needs to capture the correlations of input-input, output-output and input-output, based on discriminative and focused input and output space. To this end, we first design the Temporal Summarized Attentive Mechanism (TSAM) to capture the correlation of action-action by constructing discriminative and focused input space. Then, a Label Semantic Adaptive Mechanism (LSAM) is proposed to learn the correlation of label-label by adjusting the semantic structure according to the target task. Finally, we devise an Energy Score Estimation Mechanism (ESEM) to measure the compatibility (i.e. energy score) between video representation and label semantic embedding. With end-to-end training, our framework can capture all three of the correlations mentioned above simultaneously by minimizing the energy score of the correct action-label pair. Experiments on the HMDB51 and UCF101 datasets show that the proposed architecture achieves comparable results among methods based on the spatial-temporal visual feature of sequence-level, which demonstrates the efficiency of the EBM in guiding the framework design of ZSAR.
更多查看译文
关键词
Zero-shot action recognition, energy-based model, representative frames selection, saliency detection
AI 理解论文
溯源树
样例
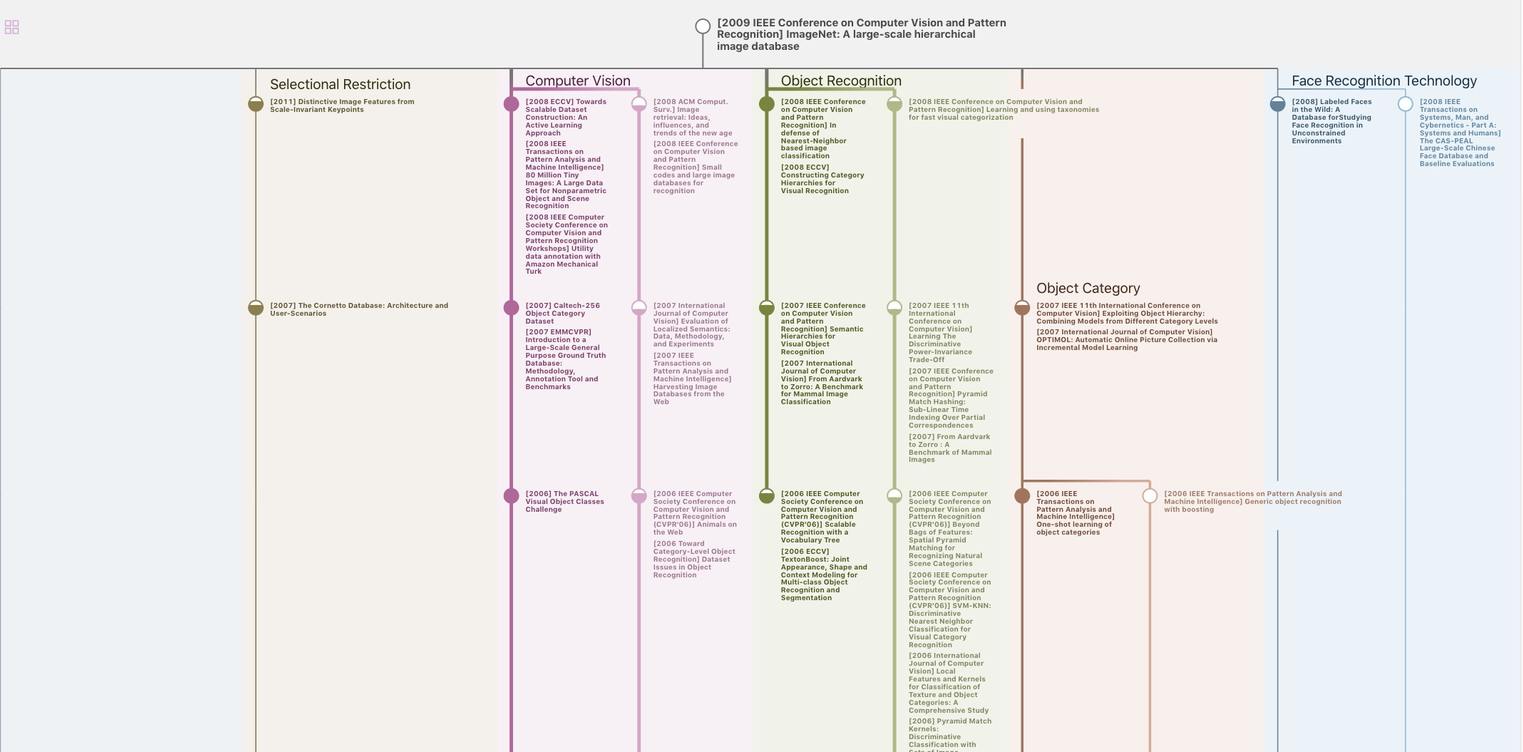
生成溯源树,研究论文发展脉络
Chat Paper
正在生成论文摘要