A Super Lightweight and Efficient SAR Image Ship Detector.
IEEE Geosci. Remote. Sens. Lett.(2023)
摘要
The realm of synthetic aperture radar (SAR) ship detection has witnessed widespread adoption of deep learning, due to its exceptional detection accuracy and end-to-end capabilities. Despite these advantages, the current SAR ship target detection methods still face the challenge of detecting small-scale targets and are difficult to be deployed on satellite platforms due to their complex models and huge computational effort. To overcome these problems, based on the YOLOv5 architecture, we present a super lightweight and efficient SAR ship target detection method named SLit-YOLOv5. Our proposed model comprises two essential components, lightweight feature extraction network (IMNet) and slim bidirectional feature pyramid network (Slim-BiFPN). IMNet serves as the backbone feature extraction network, significantly enhancing the feature extraction capability while reducing the number of parameters by half. Slim-BiFPN achieves adaptive fusion of multiscale features with fewer parameters. To validate the proposed model, we conducted an experimental evaluation on the SAR ship detection dataset (SSDD), and the results show that our SLit-YOLOv5 model outperforms the currently popular lightweight SAR ship target detection methods with high detection accuracy, low floating-point operations, and very few params.
更多查看译文
关键词
Lightweight,object detection,ship detection,synthetic aperture radar (SAR),you only look once (YOLO)
AI 理解论文
溯源树
样例
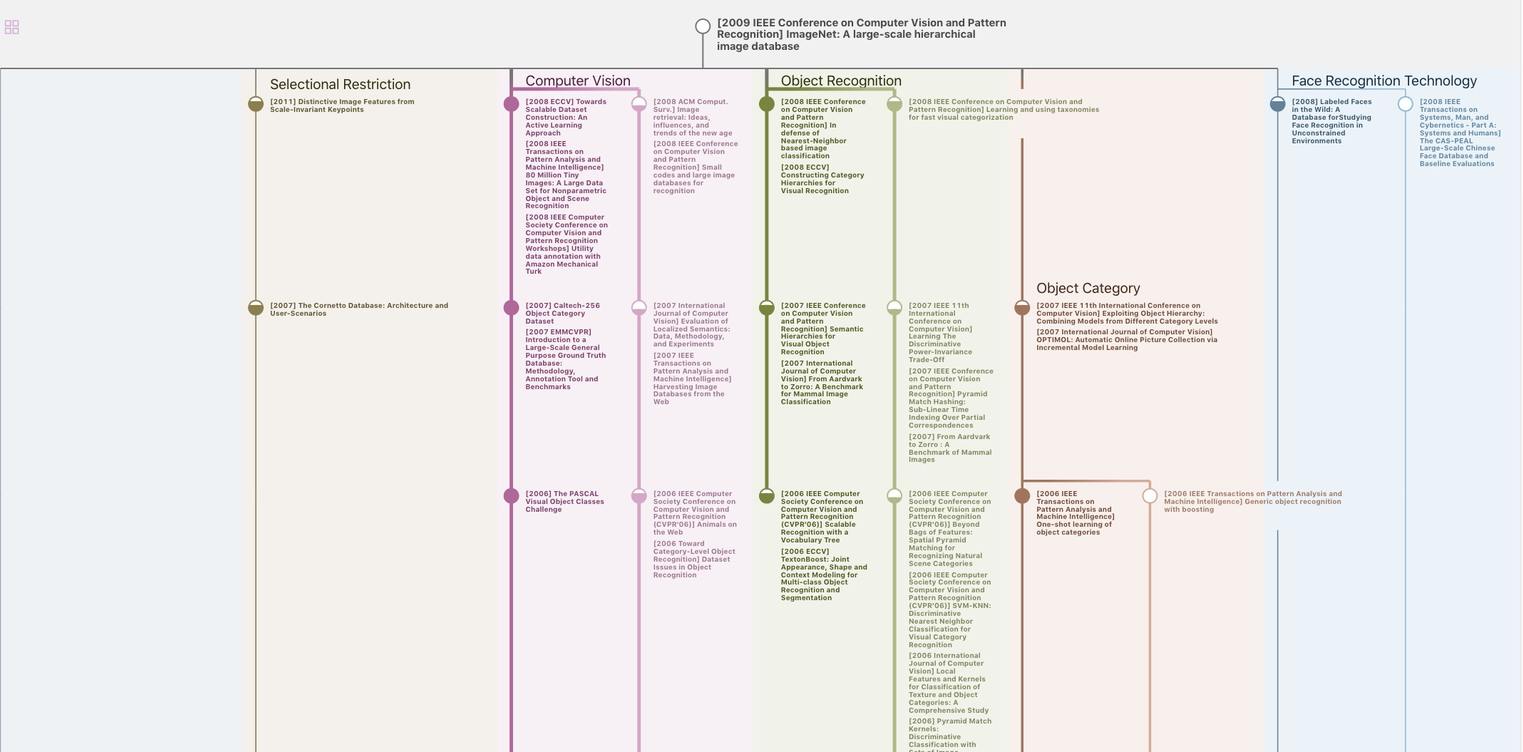
生成溯源树,研究论文发展脉络
Chat Paper
正在生成论文摘要