Multimodal Transformer Network for Hyperspectral and LiDAR Classification.
IEEE Trans. Geosci. Remote. Sens.(2023)
摘要
The land cover classification of single-modal remote sensing (RS) data has recently reached a bottleneck. The joint use of multimodal RS data to improve the classification performances has received much attention. Convolutional neural networks are powerful tools in feature extraction and contextual modeling. While they have attendant drawbacks to capture the sequence attributes of spectral signatures and struggle to acquire discriminative spectral-spatial features from a global perspective due to limitations inherent in their network backbones. The transformer backbone is a promising approach for addressing these challenges and generating novel insights in the multimodal RS image classification. In this article, we present a new model called multimodal transformer network (MTNet) that leverages transformer advantages to capture both the specific and shared characteristics of hyperspectral (HS) and light detection and ranging (LiDAR) data. HS images contain a wide range of bands with rich spectral information and LiDAR data provide accurate elevation information without affecting by environmental factors. The well-designed module HS spectral transformer can learn spectrally local sequence information from neighboring bands of HS images, yielding groupwise spectral embeddings comprising rich diagnostic information about land covers. Furthermore, the HS and LiDAR spatial transformers aim to mine the pixelwise feature embedding relationships in a global manner, capturing spatial and elevation information of HS and LiDAR, respectively. Finally, the feature embedding tokens of two modalities are integrated jointly and a new transformer encoder is redesigned to explore the shared spatial characteristics between the two modalities. We evaluate the classification performances of the proposed MTNet on three public HS-LiDAR datasets by conducting extensive experiments, exhibiting superiority over conventional classifiers and state-of-the-art networks.
更多查看译文
关键词
Class tokens,hyperspectral (HS) images,light detection and ranging (LiDAR),multimodal image classification,transformers
AI 理解论文
溯源树
样例
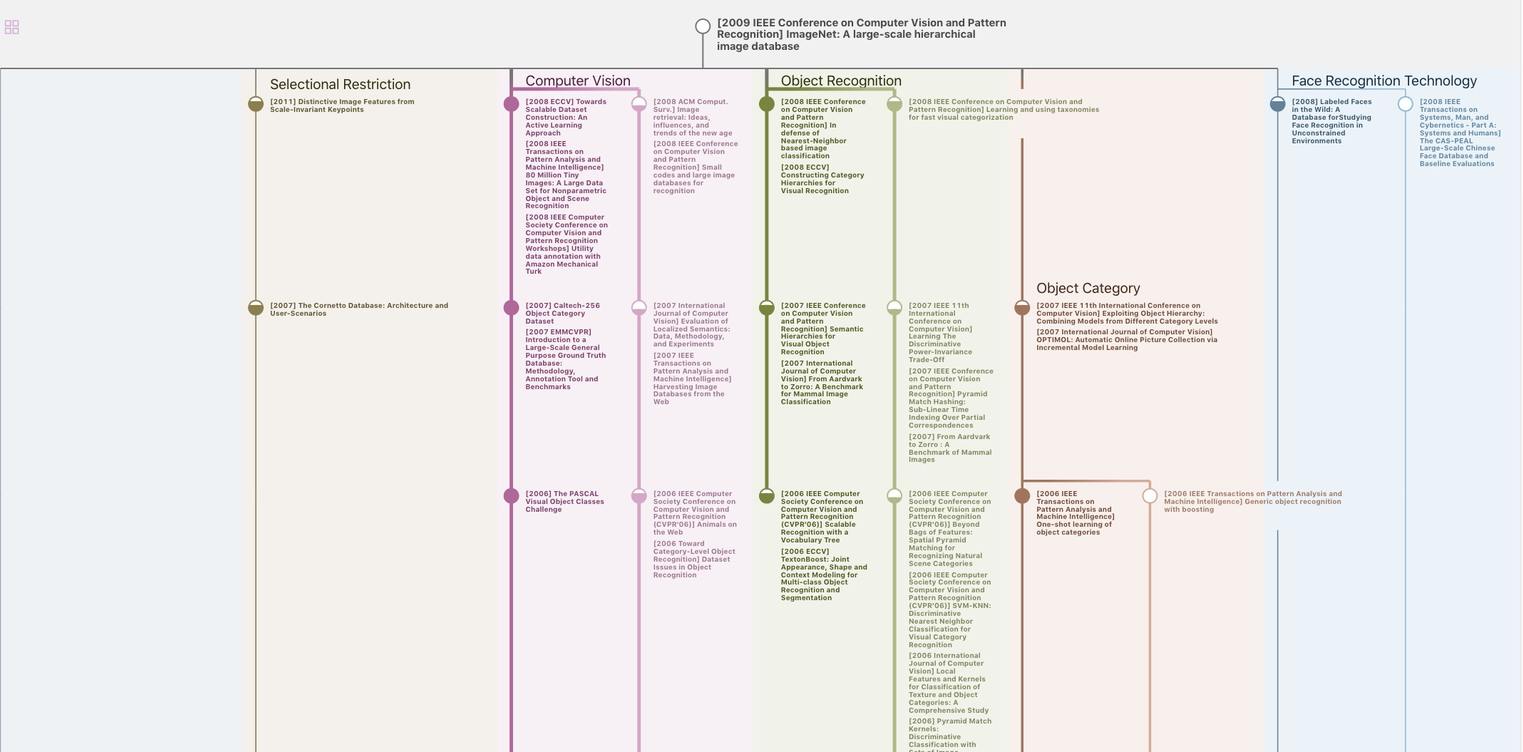
生成溯源树,研究论文发展脉络
Chat Paper
正在生成论文摘要