Thermal error prediction and control method combining residual-based one-dimensional convolution-minimum gate unit model with physical-data-edge-cloud terminal architecture
Neural Comput. Appl.(2023)
Abstract
The geometric precision of machined parts is greatly reduced due to thermal errors, thereby the thermal errors should be controlled and compensated timely to improve the machining accuracy. But the previously established thermal error models in related studies are not robust, and the predictive accuracy is not high, and the convergence is weak. Moreover, the error control delay is serious due to the network bandwidth limitation. To overcome the above challenges, the theoretical and data-driven methods are combined. The intrinsic long-term memory characteristic of thermal errors is revealed by the theoretical method and the finite element analysis, providing a theoretical guidance for the data-driven method. Then a residual-based one-dimensional convolution-minimum gate unit model is designed based on the residual connection. The designed one-dimensional convolution layer extracts the data features, and the residual connection with multiple pooling layers is used to compress the dimension of the error data. The minimal gate unit is used to improve the convergence rate. Then a new physical-data-edge-cloud terminal architecture is proposed by combining the advantages of the cloud computing and edge computing. Finally, the experiment was conducted to verify the designed physical-data-edge-cloud terminal architecture. The results indicate that the predictive accuracy is 98.18% and that the convergence time is shortened to 13.719 s compared with traditional thermal error models. Furthermore, the geometric error is reduced by more than 80%, and the total time consumption is 166 s, and the data transmission time is 25 s. The system efficiency of the designed physical-data-edge-cloud terminal system is far higher than that of physical-edge-cloud, physical-data-cloud, and physical-cloud systems.
MoreTranslated text
Key words
Thermal error,Time series prediction,Residual network,Convolutional network
AI Read Science
Must-Reading Tree
Example
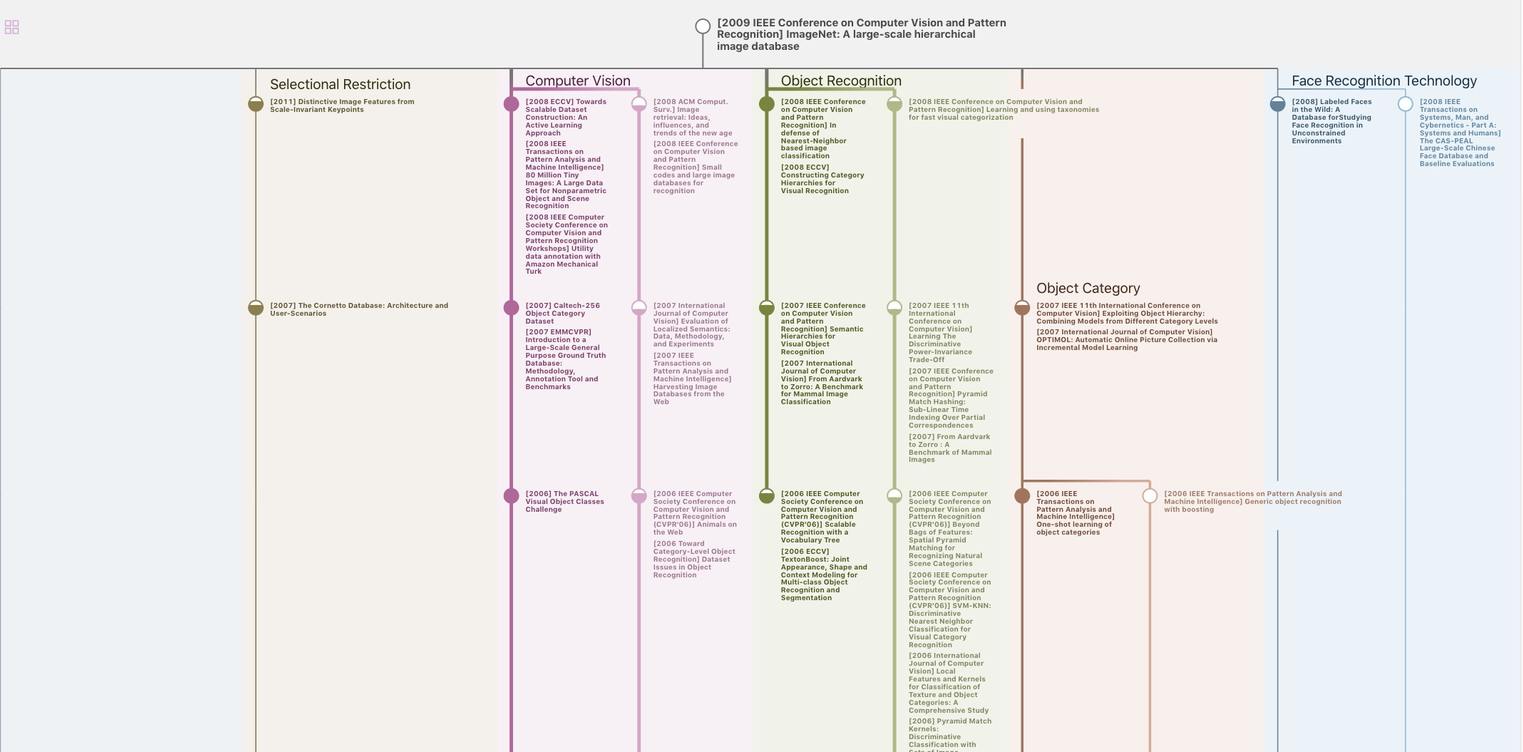
Generate MRT to find the research sequence of this paper
Chat Paper
Summary is being generated by the instructions you defined