MMST: A Multi-Modal Ground-Based Cloud Image Classification Method.
Sensors(2023)
摘要
In recent years, convolutional neural networks have been in the leading position for ground-based cloud image classification tasks. However, this approach introduces too much inductive bias, fails to perform global modeling, and gradually tends to saturate the performance effect of convolutional neural network models as the amount of data increases. In this paper, we propose a novel method for ground-based cloud image recognition based on the multi-modal Swin Transformer (MMST), which discards the idea of using convolution to extract visual features and mainly consists of an attention mechanism module and linear layers. The Swin Transformer, the visual backbone network of MMST, enables the model to achieve better performance in downstream tasks through pre-trained weights obtained from the large-scale dataset ImageNet and can significantly shorten the transfer learning time. At the same time, the multi-modal information fusion network uses multiple linear layers and a residual structure to thoroughly learn multi-modal features, further improving the model's performance. MMST is evaluated on the multi-modal ground-based cloud public data set MGCD. Compared with the state-of-art methods, the classification accuracy rate reaches 91.30%, which verifies its validity in ground-based cloud image classification and proves that in ground-based cloud image recognition, models based on the Transformer architecture can also achieve better results.
更多查看译文
关键词
ground-based cloud image (GCI) classification, Swin Transformer, global features, feature fusion
AI 理解论文
溯源树
样例
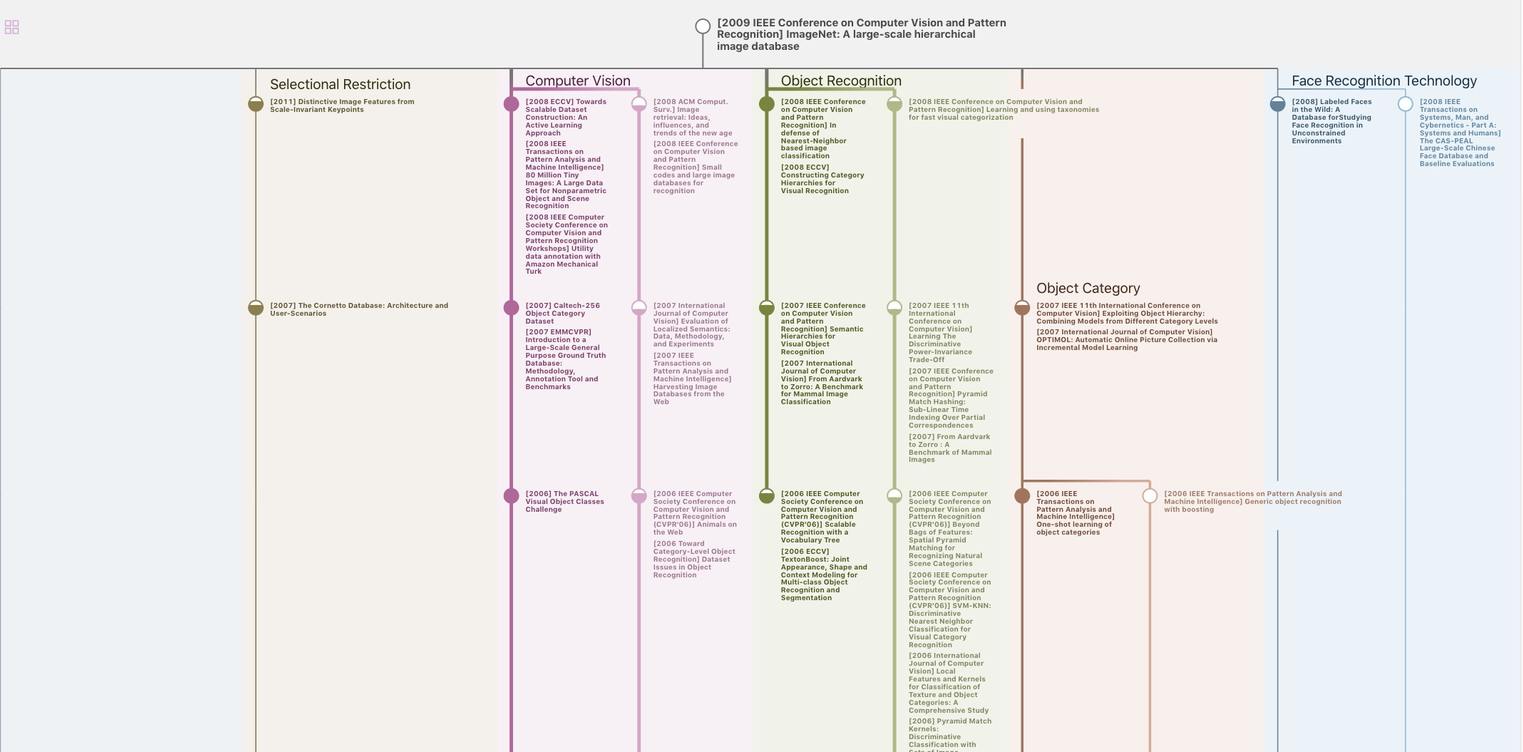
生成溯源树,研究论文发展脉络
Chat Paper
正在生成论文摘要