Fast and Accurate ROI Extraction for Non-Contact Dorsal Hand Vein Detection in Complex Backgrounds Based on Improved U-Net.
Sensors(2023)
摘要
In response to the difficulty of traditional image processing methods to quickly and accurately extract regions of interest from non-contact dorsal hand vein images in complex backgrounds, this study proposes a model based on an improved U-Net for dorsal hand keypoint detection. The residual module was added to the downsampling path of the U-Net network to solve the model degradation problem and improve the feature information extraction ability of the network; the Jensen-Shannon (JS) divergence loss function was used to supervise the final feature map distribution so that the output feature map tended to Gaussian distribution and improved the feature map multi-peak problem; and Soft-argmax is used to calculate the keypoint coordinates of the final feature map to realize end-to-end training. The experimental results showed that the accuracy of the improved U-Net network model reached 98.6%, which was 1% better than the original U-Net network model; the improved U-Net network model file was only 1.16 M, which achieved a higher accuracy than the original U-Net network model with significantly reduced model parameters. Therefore, the improved U-Net model in this study can realize dorsal hand keypoint detection (for region of interest extraction) for non-contact dorsal hand vein images and is suitable for practical deployment in low-resource platforms such as edge-embedded systems.
更多查看译文
关键词
accurate roi extraction,non-contact,u-net
AI 理解论文
溯源树
样例
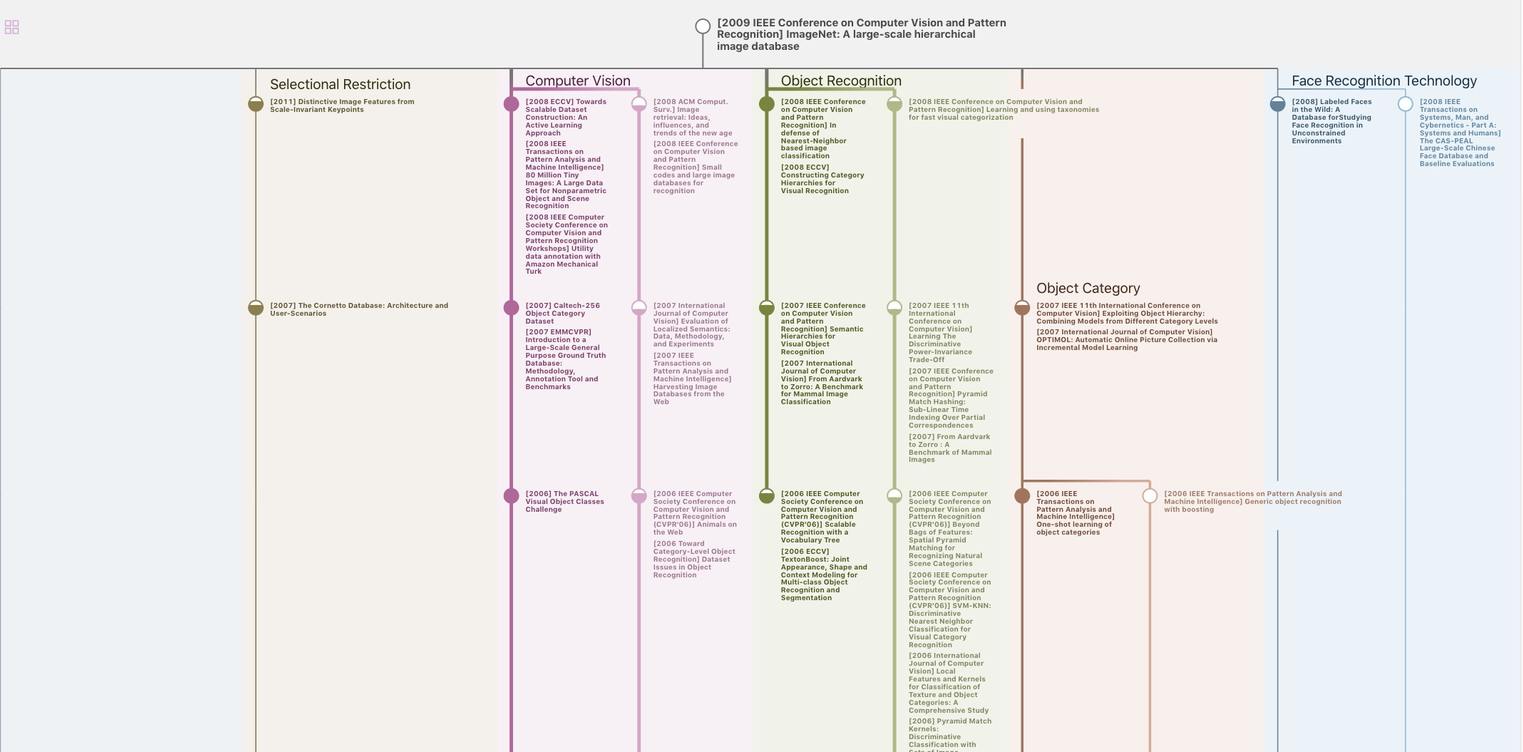
生成溯源树,研究论文发展脉络
Chat Paper
正在生成论文摘要