Noise Prior Knowledge Informed Bayesian Inference Network for Hyperspectral Super-Resolution
IEEE Trans. Image Process.(2023)
摘要
Well-known deep learning (DL) is widely used in fusion based hyperspectral image super-resolution (HS-SR). However, DL-based HS-SR models have been designed mostly using off-the-shelf components from current deep learning toolkits, which lead to two inherent challenges: i) they have largely ignored the prior information contained in the observed images, which may cause the output of the network to deviate from the general prior configuration; ii) they are not specifically designed for HS-SR, making it hard to intuitively understand its implementation mechanism and therefore uninterpretable. In this paper, we propose a noise prior knowledge informed Bayesian inference network for HS-SR. Instead of designing a "black-box" deep model, our proposed network, termed as BayeSR, reasonably embeds the Bayesian inference with the Gaussian noise prior assumption to the deep neural network. In particular, we first construct a Bayesian inference model with the Gaussian noise prior assumption that can be solved iteratively by the proximal gradient algorithm, and then convert each operator involved in the iterative algorithm into a specific form of network connection to construct an unfolding network. In the process of network unfolding, based on the characteristics of the noise matrix, we ingeniously convert the diagonal noise matrix operation which represents the noise variance of each band into the channel attention. As a result, the proposed BayeSR explicitly encodes the prior knowledge possessed by the observed images and considers the intrinsic generation mechanism of HS-SR through the whole network flow. Qualitative and quantitative experimental results demonstrate the superiority of the proposed BayeSR against some state-of-the-art methods.
更多查看译文
关键词
Bayes methods,Spatial resolution,Deep learning,Superresolution,Mathematical models,Linear programming,Gaussian noise,Hyperspectral image,super-resolution,image fusion,Bayesian inference,deep unfolding network,interpretable
AI 理解论文
溯源树
样例
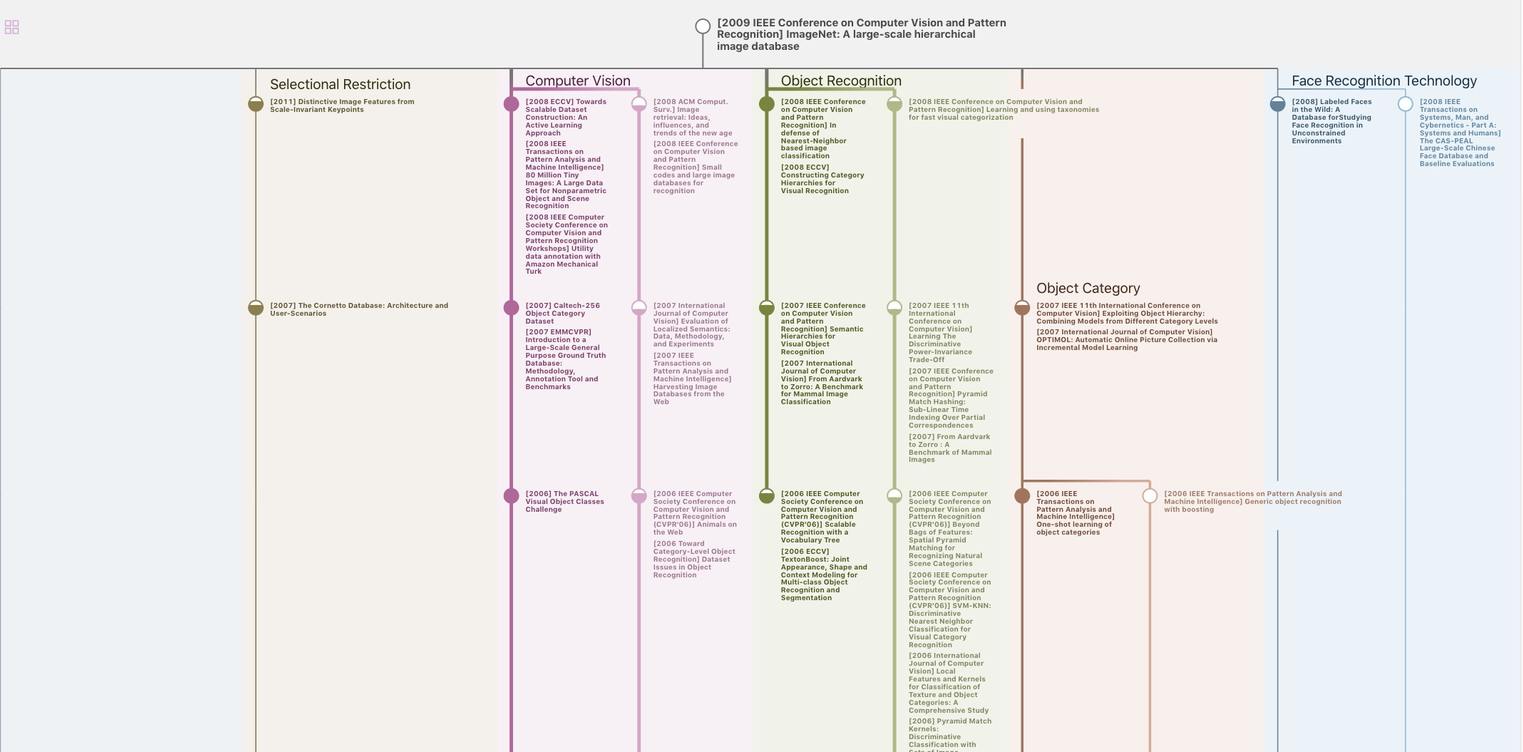
生成溯源树,研究论文发展脉络
Chat Paper
正在生成论文摘要