Weakly-Supervised 3D Spatial Reasoning for Text-Based Visual Question Answering
IEEE Trans. Image Process.(2023)
摘要
Text-based Visual Question Answering (TextVQA) aims to produce correct answers for given questions about the images with multiple scene texts. In most cases, the texts naturally attach to the surface of the objects. Therefore, spatial reasoning between texts and objects is crucial in TextVQA. However, existing approaches are constrained within 2D spatial information learned from the input images and rely on transformer-based architectures to reason implicitly during the fusion process. Under this setting, these 2D spatial reasoning approaches cannot distinguish the fine-grained spatial relations between visual objects and scene texts on the same image plane, thereby impairing the interpretability and performance of TextVQA models. In this paper, we introduce 3D geometric information into the spatial reasoning process to capture the contextual knowledge of key objects step-by-step. Specifically, (i) we propose a relation prediction module for accurately locating the region of interest of critical objects; (ii) we design a depth-aware attention calibration module for calibrating the OCR tokens’ attention according to critical objects. Extensive experiments show that our method achieves state-of-the-art performance on TextVQA and ST-VQA datasets. More encouragingly, our model surpasses others by clear margins of 5.7% and 12.1% on questions that involve spatial reasoning in TextVQA and ST-VQA valid split. Besides, we also verify the generalizability of our model on the text-based image captioning task.
更多查看译文
关键词
3d,weakly-supervised,text-based
AI 理解论文
溯源树
样例
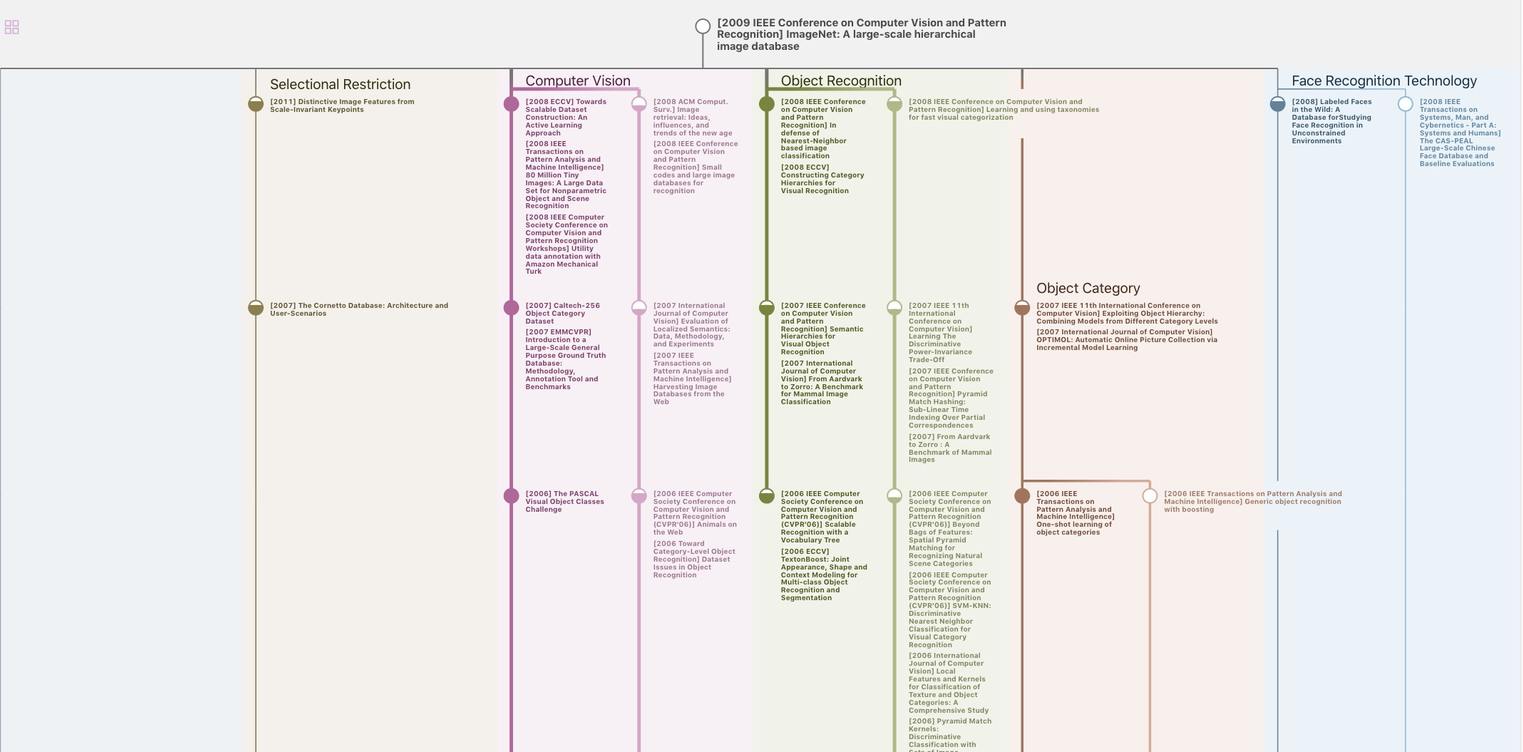
生成溯源树,研究论文发展脉络
Chat Paper
正在生成论文摘要