Magi-Net: Meta Negative Network for Early Activity Prediction
IEEE Trans. Image Process.(2023)
摘要
Early activity prediction/recognition aims to recognize action categories before they are fully conveyed. Compared to full-length action sequences, partial video sequences only provide insufficient discrimination information, which makes predicting the class labels for some similar activities challenging, especially when only very few frames can be observed. To address this challenge, in this paper, we propose a novel meta negative network, namely, Magi-Net, that utilizes a contrastive learning scheme to alleviate the insufficiency of discriminative information. In our Magi-Net model, the positive samples are generated by augmenting an input anchor conditioned on all observation ratios, while the negative samples are selected from a trainable negative look-up memory (LUM) table, which stores the training samples and the corresponding misleading categories. Furthermore, a meta negative sample optimization strategy (MetaSOS) is proposed to boost the training of Magi-Net by encouraging the model to learn from the most informative negative samples via a meta learning scheme. Extensive experiments are conducted on several public skeleton-based activity datasets, and the results show the efficacy of the proposed Magi-Net model.
更多查看译文
关键词
Predictive models,Video sequences,Task analysis,Optimization,Prediction algorithms,Adaptation models,Training,Early activity prediction,skeleton-based action recognition,meta learning
AI 理解论文
溯源树
样例
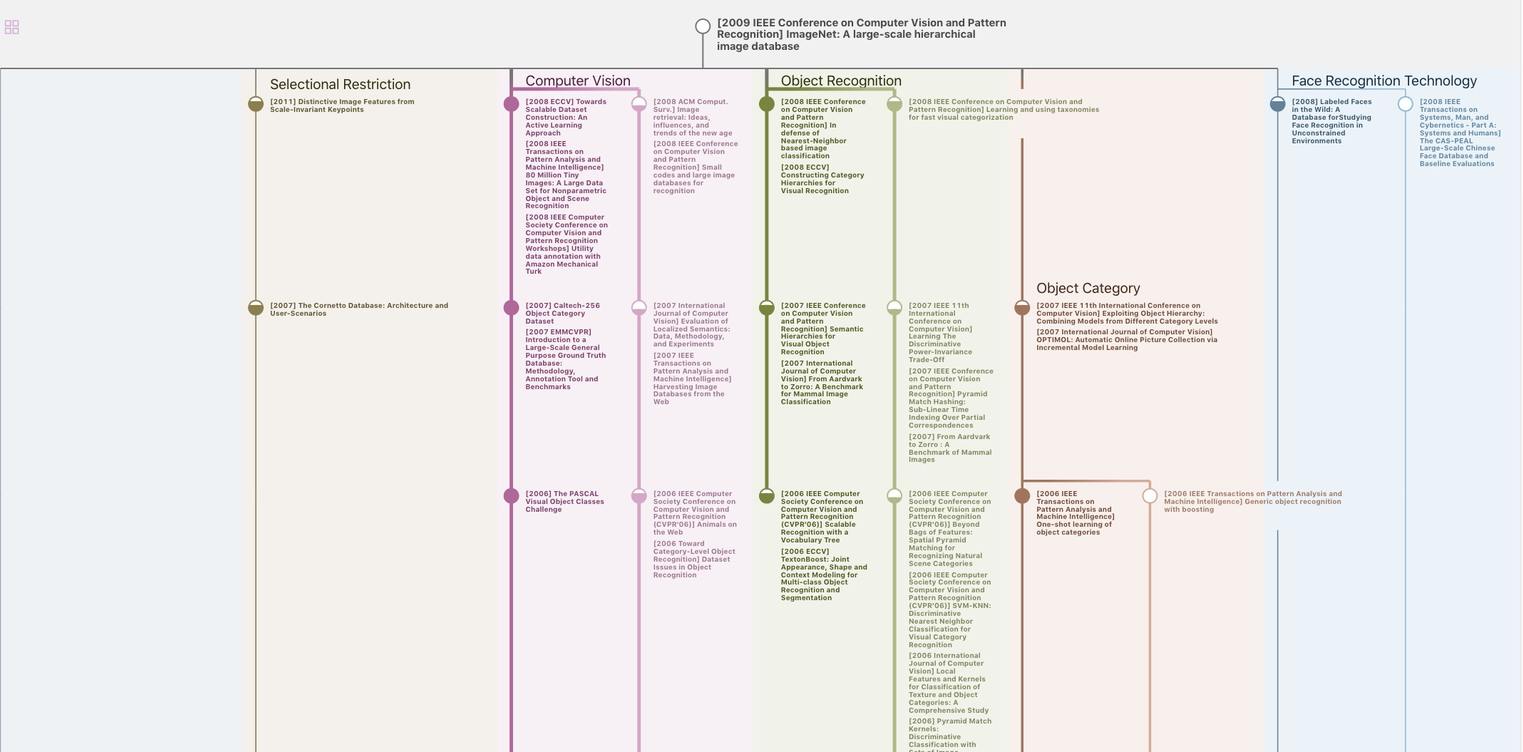
生成溯源树,研究论文发展脉络
Chat Paper
正在生成论文摘要