Transferable graph neural networks with deep alignment attention.
Inf. Sci.(2023)
摘要
When analyzing graph-structured data, graph neural networks (GNNs) use well-designed propagation mechanism to extract node features. However, these methods only incorporate data from nodes' fixed neighborhoods or whole graph, not accounting for the influence of important nodes outside of the neighborhoods. We offer transferable graph neural networks with deep alignment attention (TGDAA), which is a novel neural network framework that designs aligned attention to assign different weights to related important nodes. In such a scenario, we concurrently remove a number of graph neural network obstacles and make TGDAA accessible for transfer learning issues. Extensive experiments in four aspects: traditional metrics, attention-related methods, varying label rates and ablation study show that the suggested TGDAA performs better than ten state-of-the-art techniques on ten benchmark network datasets.
更多查看译文
关键词
GNNs, Transfer learning, Attention mechanism
AI 理解论文
溯源树
样例
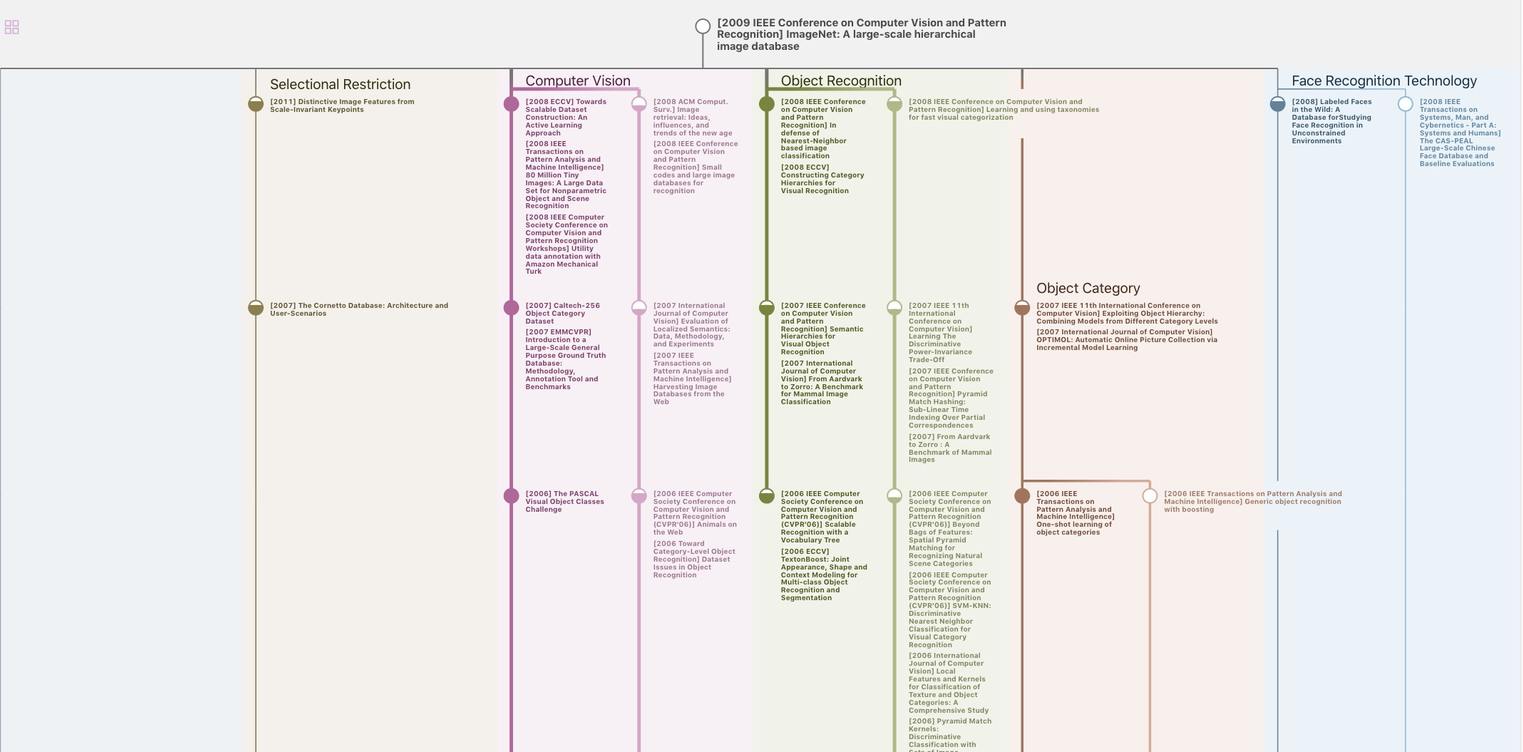
生成溯源树,研究论文发展脉络
Chat Paper
正在生成论文摘要