A New ECG Data Processing Approach to Developing an Accurate Driving Fatigue Detection Framework with Heart Rate Variability Analysis and Ensemble Learning.
Inf.(2023)
摘要
More than 1.3 million people are killed in traffic accidents annually. Road traffic accidents are mostly caused by human error. Therefore, an accurate driving fatigue detection system is required for drivers. Most driving fatigue detection studies concentrated on improving feature engineering and classification methods. We propose a novel driving fatigue detection framework concentrating on the development of the preprocessing, feature extraction, and classification stages to improve the classification accuracy of fatigue states. The proposed driving fatigue detection framework measures fatigue using a two-electrode ECG. The resampling method and heart rate variability analysis were used to extract features from the ECG data, and an ensemble learning model was utilized to classify fatigue states. To achieve the best model performance, 40 possible scenarios were applied: a combination of 5 resampling scenarios, 2 feature extraction scenarios, and 4 classification model scenarios. It was discovered that the combination of a resampling method with a window duration of 300 s and an overlap of 270 s, 54 extracted features, and AdaBoost yielded an optimum accuracy of 98.82% for the training dataset and 81.82% for the testing dataset. Furthermore, the preprocessing resampling method had the greatest impact on the model's performance; it is a new approach presented in this study.
更多查看译文
关键词
fatigue detection, resampling, electrocardiogram, fatigue driving, heart rate variability analysis
AI 理解论文
溯源树
样例
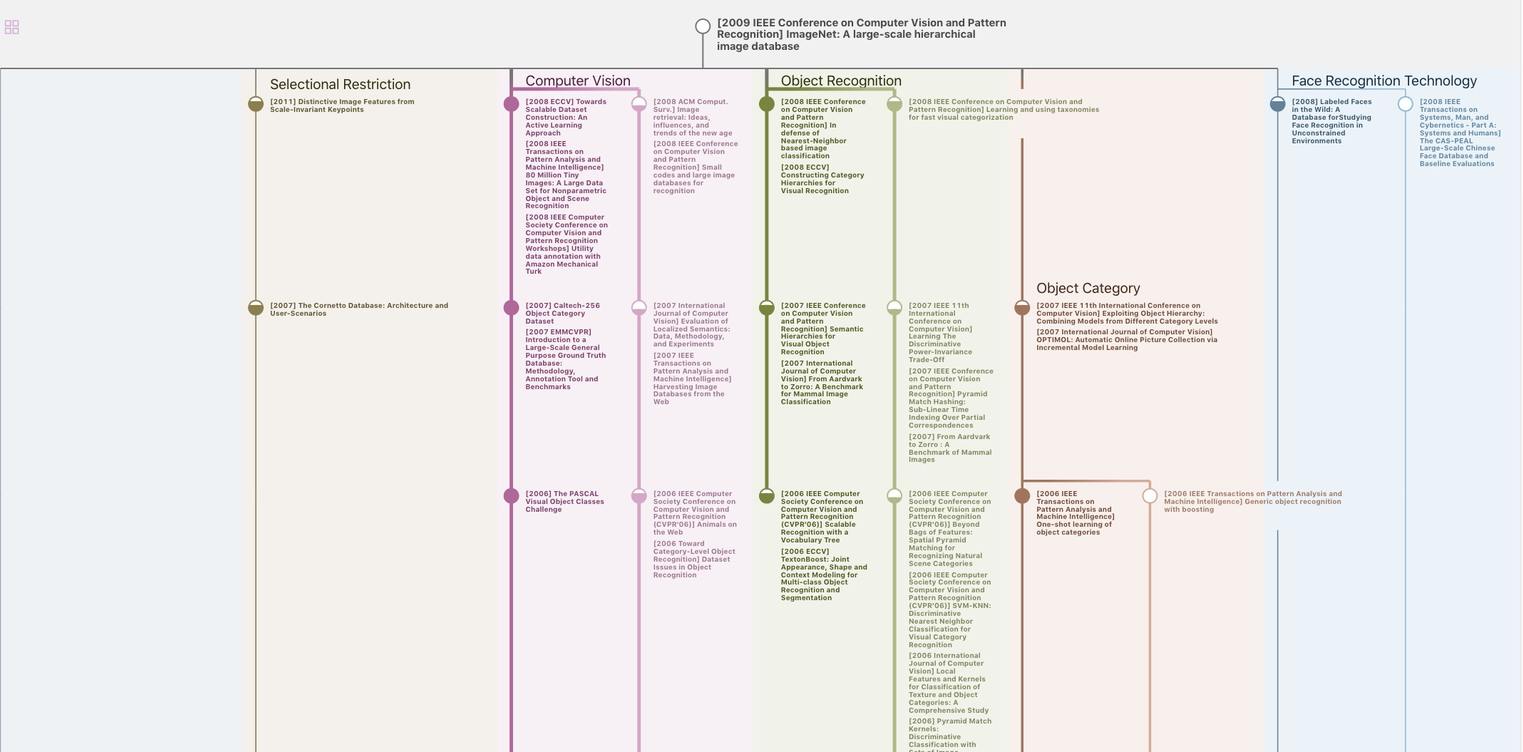
生成溯源树,研究论文发展脉络
Chat Paper
正在生成论文摘要