HDGN: Heat diffusion graph network for few-shot learning
PATTERN RECOGNITION LETTERS(2023)
Abstract
A heat diffusion graph network (HDGN) is proposed in this paper, which retains more similar graph sig-nals in the spectral domain, for few-shot learning. Convolution on the graph is essentially the filtering of the graph signal. Most existing graph-network-based few-shot learning methods process graph signals with high-pass filters to get the difference in information. However, the low-frequency similar informa-tion is usually more valuable in the few-shot tasks. A joint low-pass filter is constructed to filter low -frequency graph signals, that is, heat kernel convolution aggregates similar information from neighboring nodes. The obtained low-frequency similarity information is utilized to update the representations of nodes on the graph. In addition, a more robust mixed metric is designed to dynamically update the edge feature of the graph. Predicting Unknown Nodes on Graphs by Alternating Updates of Node Representa-tion and Edge Matrix. The experimental results also demonstrate that HDGN achieves better performance for the few-shot classification task.& COPY; 2023 Published by Elsevier B.V.
MoreTranslated text
Key words
Few-shot learning,Graph convolution network,Low-pass filter,Heat diffusion,Gait recognition,Image entropy,Extreme learning machine,Multi-view recognition
AI Read Science
Must-Reading Tree
Example
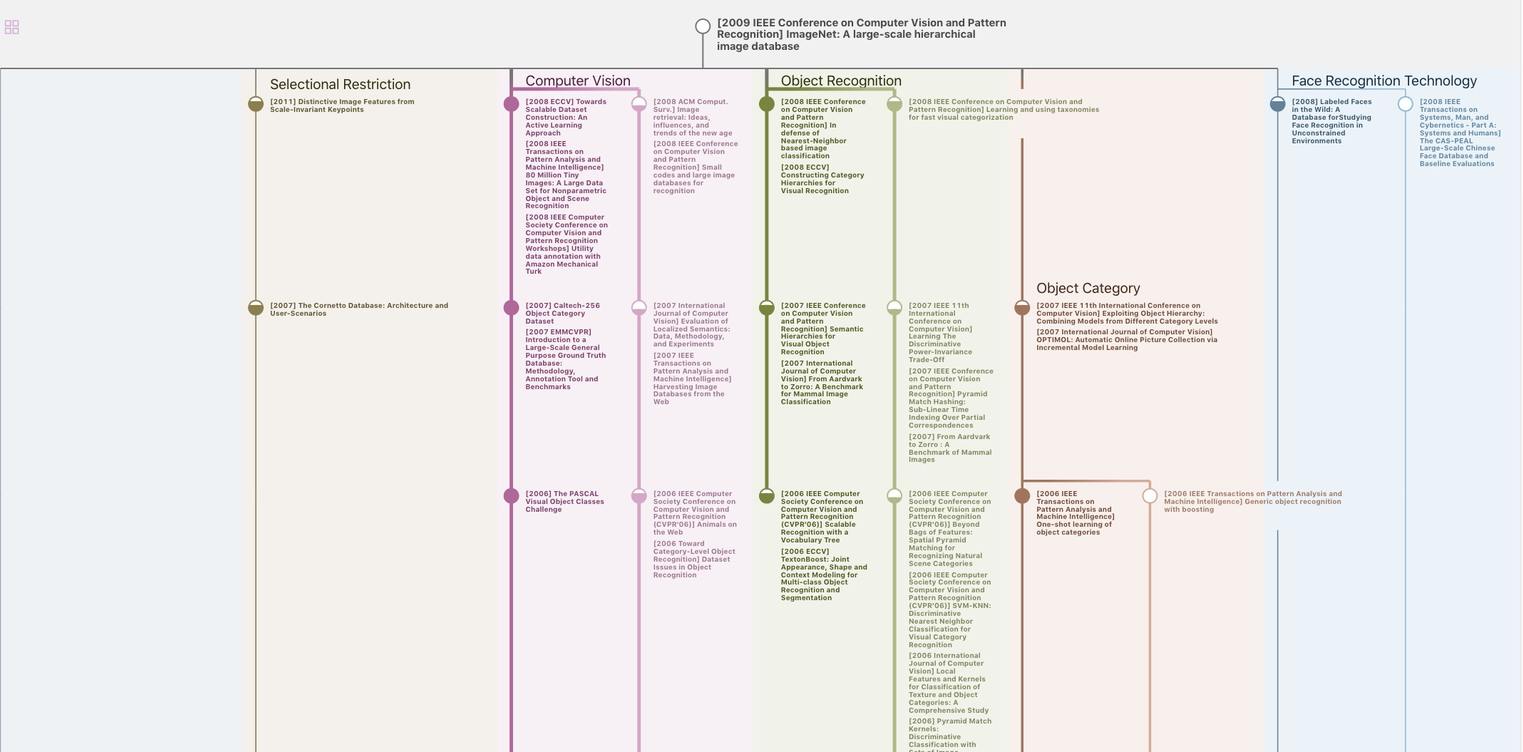
Generate MRT to find the research sequence of this paper
Chat Paper
Summary is being generated by the instructions you defined