Data generation using geometrical edge probability for one-class support vector machines
Expert Syst. Appl.(2023)
摘要
In the tasks of data mining and machine learning with unlabeled data, one-class support vector machines (OCSVMs) are one of the most widely used methods for anomaly detection as one class classification. However, the choice of hyperparameters, a critical step for learning effective OCSVM decision boundaries, remains open as a post analysis step and is often undecided. To tackle this issue, this paper proposes a new data-generation method using geometrical edge probability suitable for OCSVM hyperparameter selection. Our method improves the limitations of existing methods in that the geometrical edge probability enables the generation of both pseudo target data and pseudo anomaly data. In addition, the proposed method combined with bootstrapping is able to determine the distribution of anomaly data. We evaluate the proposed method for datasets with 16 different dimensions and demonstrate the performance improvement in the classification of target and anomaly data.
更多查看译文
关键词
Outlier detection,Parameter selection,One-class classification,Sampling techniques
AI 理解论文
溯源树
样例
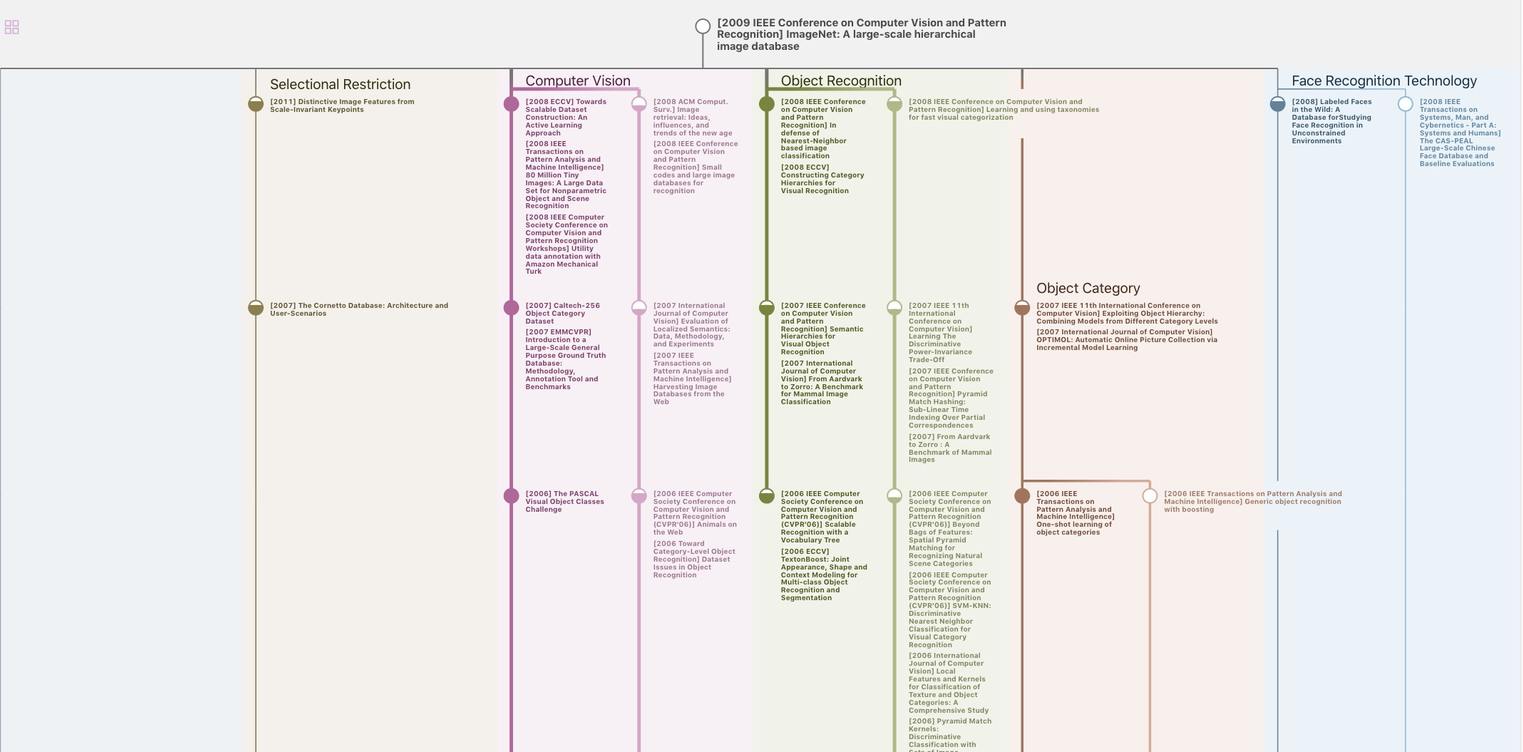
生成溯源树,研究论文发展脉络
Chat Paper
正在生成论文摘要