Efficient skeleton-based action recognition via multi-stream depthwise separable convolutional neural network.
Expert Syst. Appl.(2023)
摘要
Skeleton-based human action recognition has attracted considerable attention and achieved great success in several engineering fields, which is also one of the most active research topic in computer vision community. However, the existing methods may suffer from a large model size and slow execution speed. In addition, some useful information embedded in skeleton sequence, such as the motion information with different scales, had not been fully utilized, such that the performance of recognition is compromised or decreased. To this end, we propose an efficient skeleton-based action recognition approach based on multi-stream neural networks, namely Lightweight Double-feature Triple-scale motion Network (LDT-NET). Precisely, by endowing a lightweight network structure, i.e., multi-stream Depthwise Separable Convolutional Neural Network, LDT-NET is capable of being employed efficiently on a single CPU/GPU. The experimental results on several famous datasets tested by almost all studies in this field, such as SHREC (i.e., hand actions) and JHMDB (i.e., body actions), the proposed LDT-NET achieves promising performance against the state-of-the-art methods. More promisingly, compared to the latest representative work, such as DD-NET, LDT-NET achieves a speedup both in training and inferring by 25%, with only about 20% of its model parameter. In a nutshell, our method not only achieves higher recognition rate but can be used in practical application comparing to the state-of-the-art methods.
更多查看译文
关键词
Skeleton-based action recognition, Lightweight, Multi-scale motion information, Depthwise separable convolution
AI 理解论文
溯源树
样例
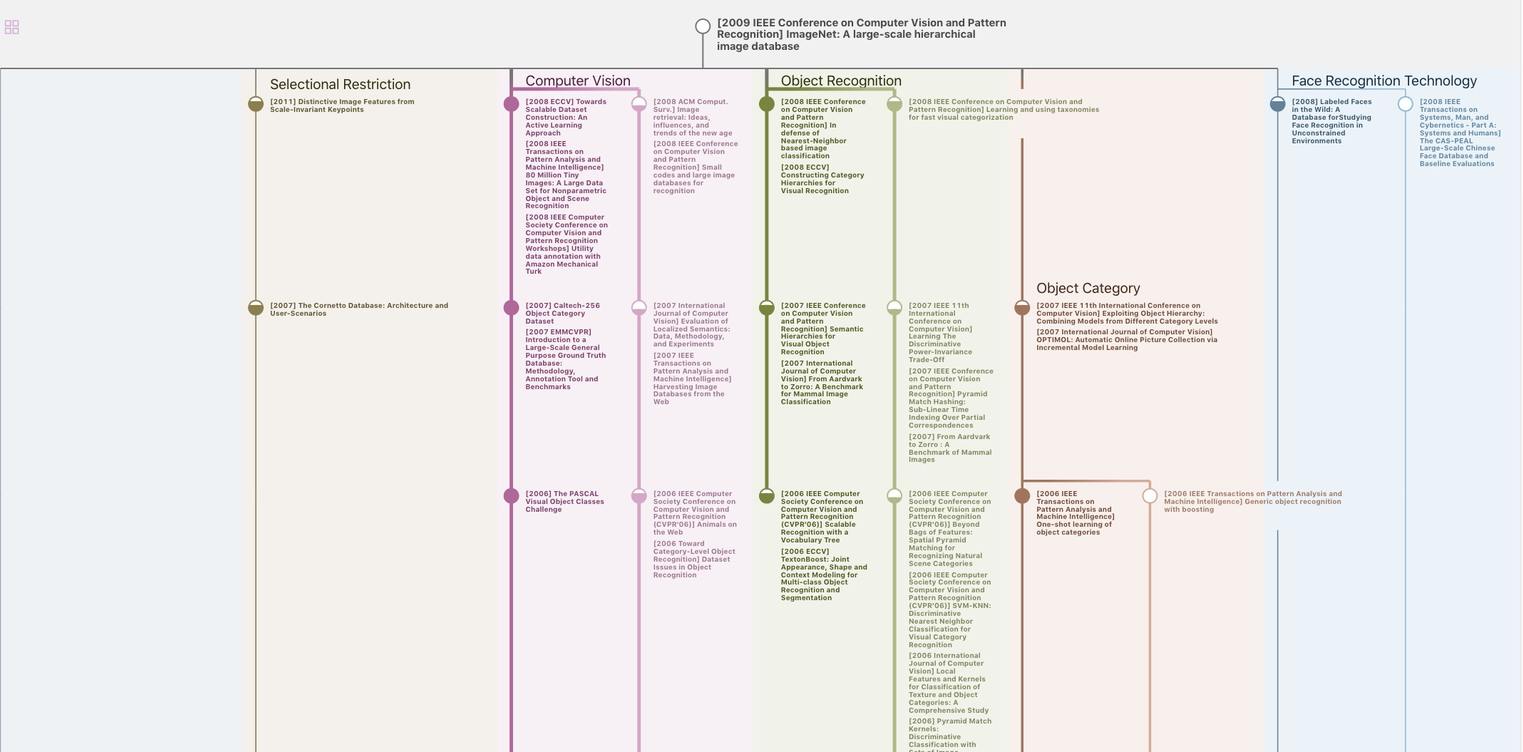
生成溯源树,研究论文发展脉络
Chat Paper
正在生成论文摘要