USWAVG-BS: Under-Sampled Weighted AVeraGed BorderlineSMOTE to handle data intrinsic difficulties
Expert Syst. Appl.(2023)
Abstract
In two-class classification problems, learning from imbalanced data is a challenging task due to the bias of machine learning algorithms towards the majority class. It has been shown in some studies that the imbalance problem is not the only reason for this difficulty, and other issues related to the nature of the data, such as small disjunct problems, borderline and rare examples, overlap between classes, and data shift, also contribute. To address this issue, an Under-Sampled Weighted AVeraGed BorderlineSMOTE (USWAVG-BS) is proposed in this paper, which consists of three phases: determining the types of examples, under-sampling, and over-sampling. In the first phase, the feature space is transformed into the Heterogeneous Value Distance Metric (HVDM) space, and a new formula based on the imbalance ratio is defined to identify noise examples from the majority class. In the second phase, noise examples from the majority class are either converted to the minority class or removed until a specific threshold between the two classes is reached. In the last phase, new examples are generated using a similar way to Safe-level-SMOTE and LN-SMOTE to further boost the minority class by emphasizing minority class regions. The proposed approach's performance is evaluated using recall, precision, and f1-score as per-formance criteria on 10 real-world data sets using the JRip algorithm. Moreover, USWAVG-BS is compared with other sampling methods including 3 under-sampling, 7 over-sampling, and 4 hybrid ones using f1-score metric. The obtained results indicate that the proposed approach outperforms other methods significantly using the Wilcoxon signed-rank test. It is worth mentioning that RandomUnderSampler, despite its simplicity, performs better than two other under-sampling methods on average and SMOTE performs better than its extensions such as BorderlineSMOTE and ASN-SMOTE on the examined data sets.
MoreTranslated text
Key words
Data intrinsic difficulties,Imbalance data set,Sampling,SMOTE,BorderlineSMOTE,HVDM
AI Read Science
Must-Reading Tree
Example
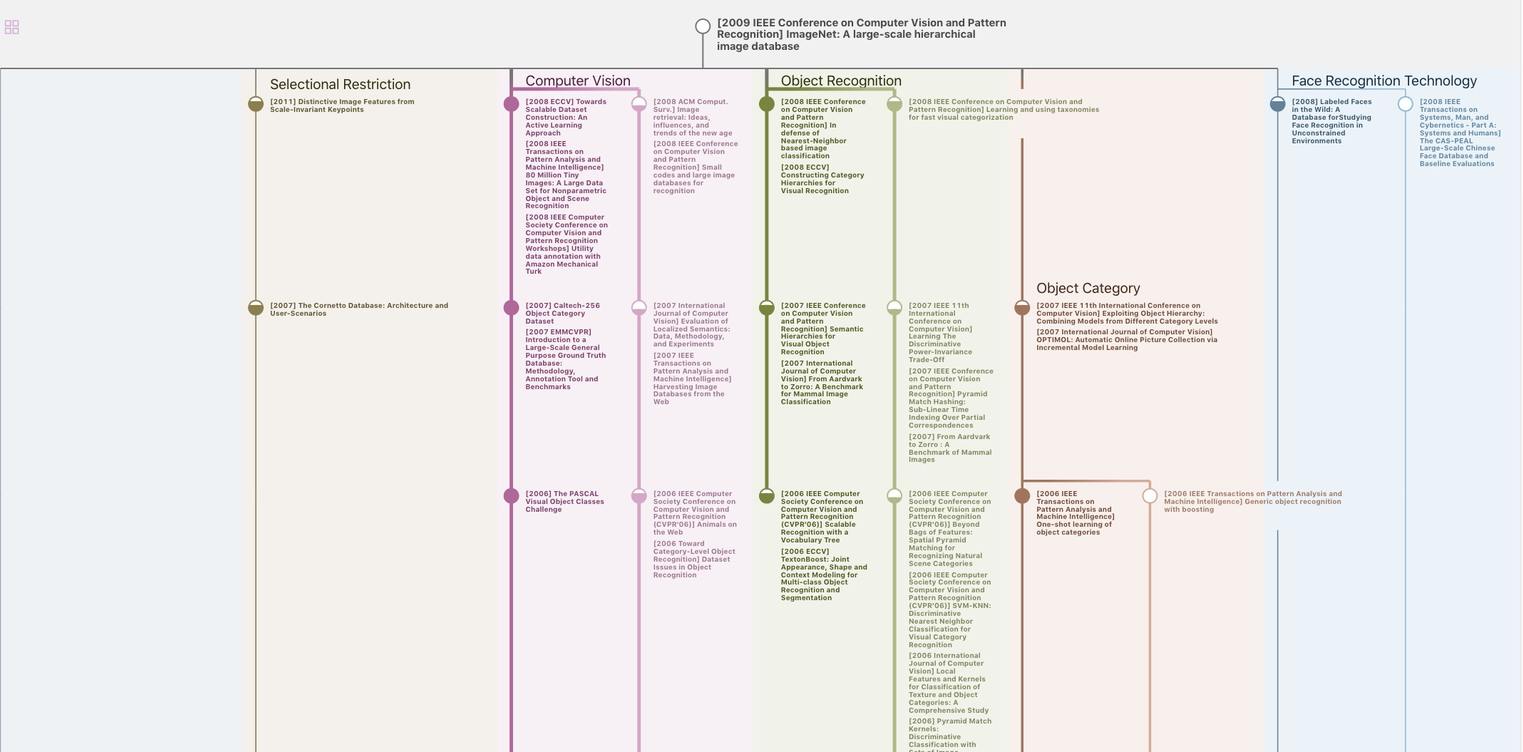
Generate MRT to find the research sequence of this paper
Chat Paper
Summary is being generated by the instructions you defined