A Generally Regularized Inversion for NMR Applications and Beyond
IEEE Trans. Instrum. Meas.(2023)
摘要
Nuclear magnetic resonance (NMR) technique presents a powerful analytical tool in the fields of chemistry, biology, and material science. The performance of the NMR technique highly relies on post-data processing methods, which are diverse according to different applications. In this article, we propose a general processing method that can cover a wide range of applications from molecular dynamics study to biomedical engineering. We analyze the computation performance of the method by comparing it to other well-known algorithms and demonstrate its application in various NMR data processing problems, i.e., diffusion-ordered spectroscopy processing, under-sampled magnetic resonance imaging (MRI) reconstruction, Laplace NMR (LNMR) inversion, and spectrum denoising. The results corroborate that the method performs effectively and efficiently compared to state-of-the-art methods. Moreover, we construct the method as a light graphic-based toolbox for convenient use. More detail instructions of the toolbox for chemists will be given in future works.
更多查看译文
关键词
Alternative direction multiplier method (ADMM),inverse problem,low-rank,magnetic resonance imaging (MRI),magnetic resonance spectroscopy,prior knowledge,regularization,sparsity
AI 理解论文
溯源树
样例
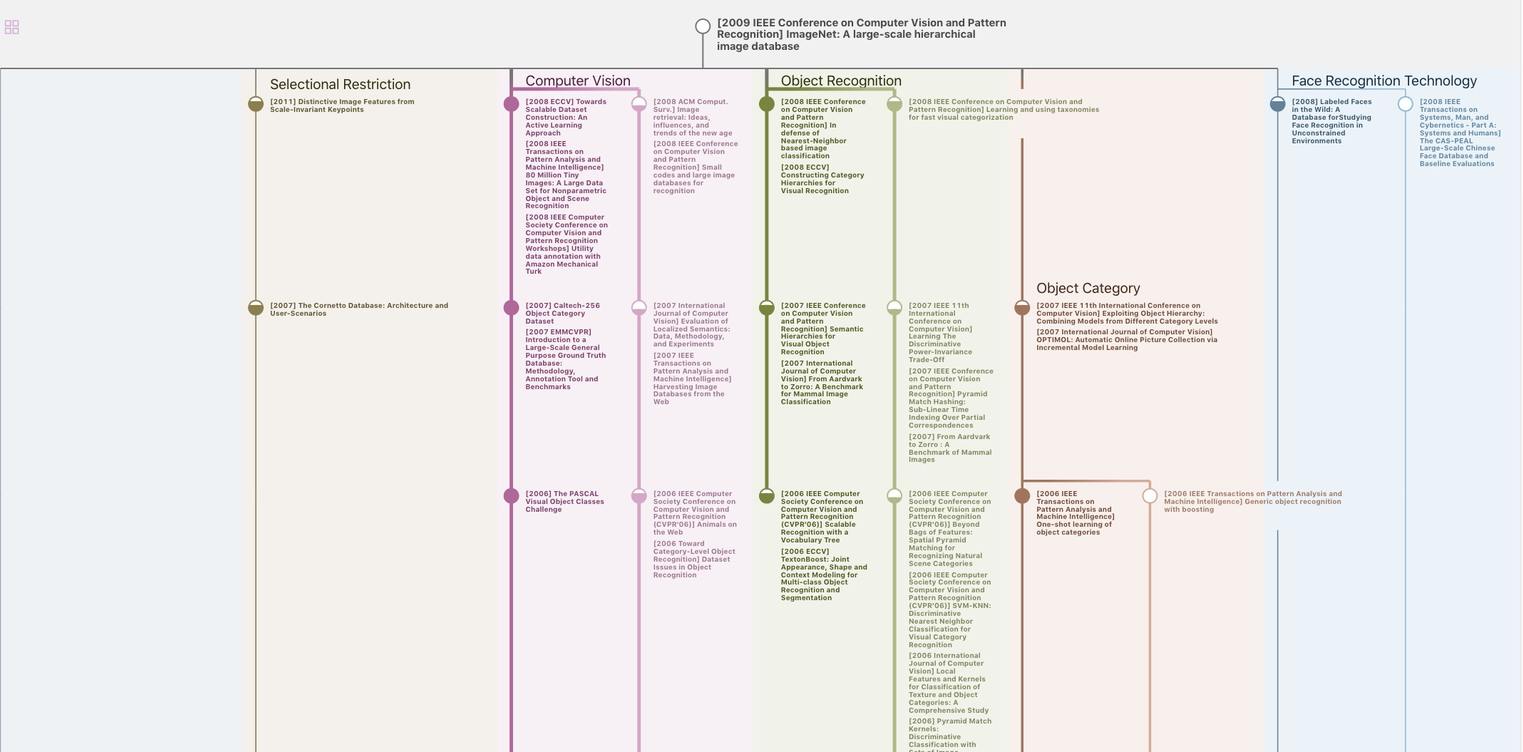
生成溯源树,研究论文发展脉络
Chat Paper
正在生成论文摘要