Online Process Monitoring Based on Proprioceptive Signals for Robotic Grinding.
IEEE Trans. Instrum. Meas.(2023)
摘要
Actual robotic grinding depth is difficult to control due to the robot and workpiece's weak rigidity. The online monitoring of the robotic grinding state is urgently needed for suppressing abnormal conditions and improving surface quality. Previous methods heavily relied on various additional sensors. Multisensor proprioceptive signals are not effectively used. Recently, data-driven methods have been used in machining monitoring. However, enormous samples with high costs are required for the training of the deep learning-based monitoring model. This article proposes a proprioceptive signal-based online process monitoring method for robotic grinding. First, a multisensor information fusion approach based on velocity synchronous linear chirplet transform (VSLCT) converts the proprioceptive current signals to the fused time-frequency (TF) images. It extracts the intrinsic features of nonstationary signals without user interventions. Second, the mix-up data augmentation approach derived from the constrained convolutional autoencoder (CCAE) enhances the machining dataset with a better sample generation quality. Third, a residual convolutional neural network (CNN) for classification is designed to complete the grinding monitoring task. Finally, the robotic grinding experiments demonstrate higher classification performance by up to 99.69% under laboratory machining conditions. In addition, the suggested approach performs better under various noises and small training-set proportions than other advanced methods.
更多查看译文
关键词
Convolutional neural network (CNN)-based monitoring model,multisensor information fusion,online grinding monitoring,robot proprioceptive signals,small samples and noises condition
AI 理解论文
溯源树
样例
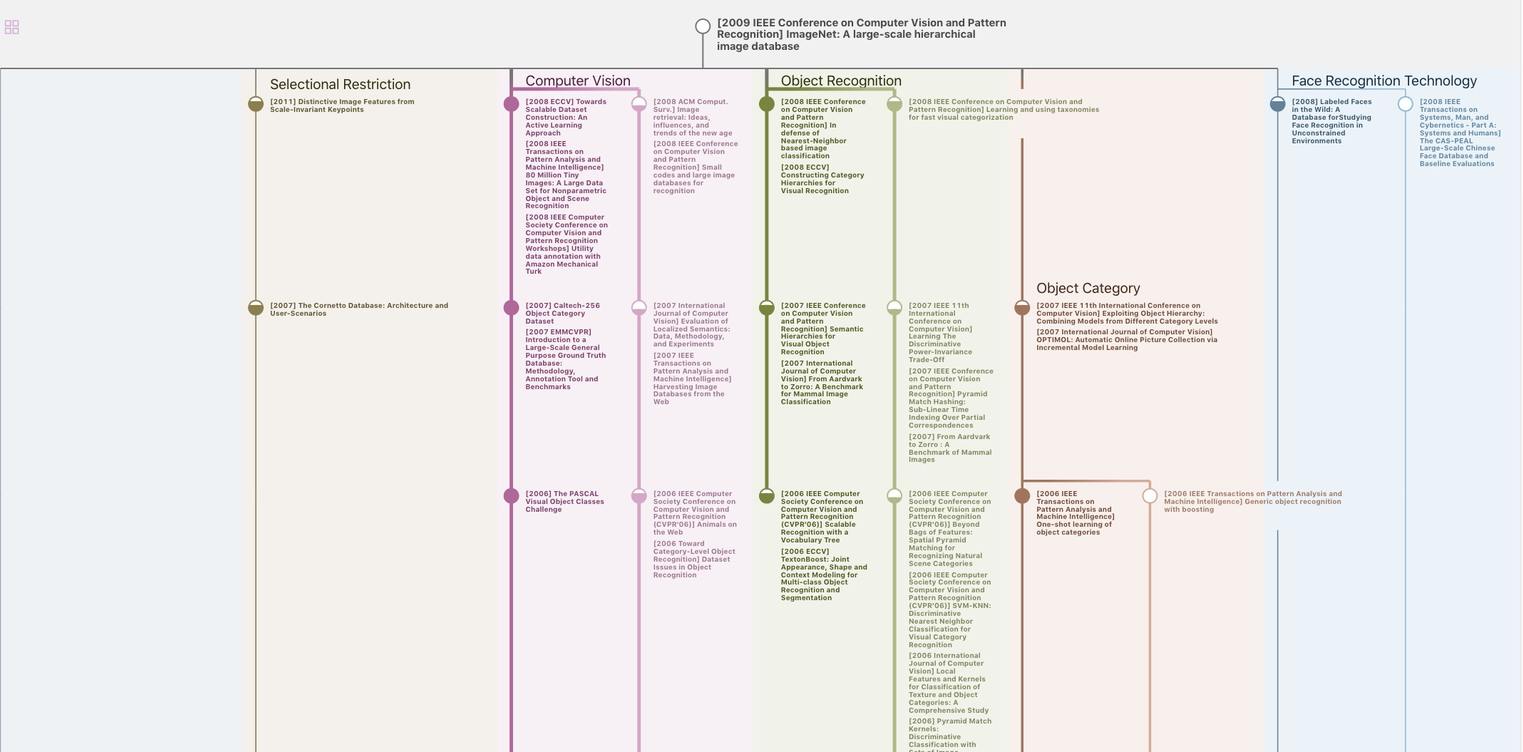
生成溯源树,研究论文发展脉络
Chat Paper
正在生成论文摘要