Federated Learning Aided Deep Convolutional Neural Network Solution for Smart Traffic Management.
NOMS(2023)
摘要
Machine learning models, especially neural network (NN) classifiers, have shown tremendous potential of being used in complex tasks such as image classification, object detection and video analytics. However, to be adopted in the real-world applications, there are still problems to be answered. One of these problems is that training machine learning models, especially NN models, requires a certain level of computation and data processing. Other problems are the limited bandwidth of the network and the possibility of exposing the privacy of the users to attacks if the training data (specially video) is going to be transferred through the network. To mitigate these problems, researchers recently proposed the concept of federated learning.In this paper, we build a video analytic application for traffic management and train it using federated learning. More specifically, each traffic surveillance camera combined with its co-located small PC are seen as the worker node in federated learning. In this way, the NN model in each node can be trained on data collected from all nodes without transmitting and sharing with a central server, which resolves all of the above mentioned problems. The performance of the trained NN model is evaluated via experiments under different open sourced datasets to demonstrate that the proposed work has the potential to enhance the detection accuracy (mAP) over 40%.
更多查看译文
关键词
Machine Learning,Neural Network,Traffic Video Analytic
AI 理解论文
溯源树
样例
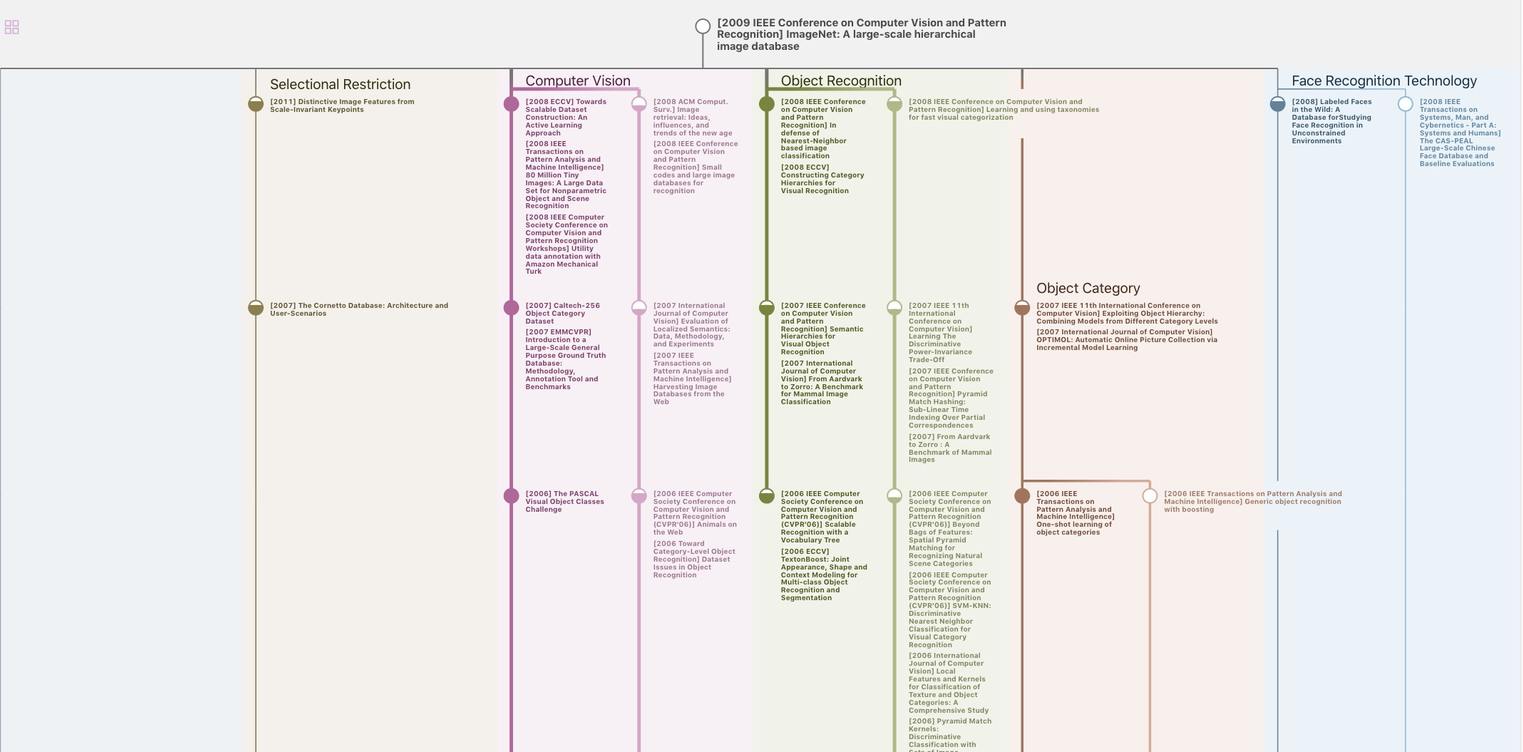
生成溯源树,研究论文发展脉络
Chat Paper
正在生成论文摘要