Domain Adaptation for Network Performance Modeling with and without Labeled Data
NOMS(2023)
Abstract
Network performance modeling using machine learning (ML) has proven to be essential for proactive network and service management. Dynamic changes and re-configurations in the network infrastructure can impact the data distribution, causing degradation in the performance of the deployed ML models. To adapt to such changes, traditional approaches suggest that the models must be updated using new labeled data from the operational network. Unfortunately, these labels can be costly or impossible to obtain.In this paper, we propose a customized approach named cDANN using domain adaptation to target these challenges for network performance modeling. We report on an empirical study where we evaluate the impact of labeled data availability, ranging from no available labels to a fully labeled data set. We specifically study scenarios for network performance modeling using two realistic data sets; one obtained from a cloud testbed environment and the other from a 5G - mm Wave testbed. Our results show that incorporating unlabeled samples reduces the need for costly collection of labeled data. The proposed method either radically improves or performs on par with the baselines.
MoreTranslated text
Key words
Network Performance,5G,Machine Learning,Transfer Learning,Domain Adaptation
AI Read Science
Must-Reading Tree
Example
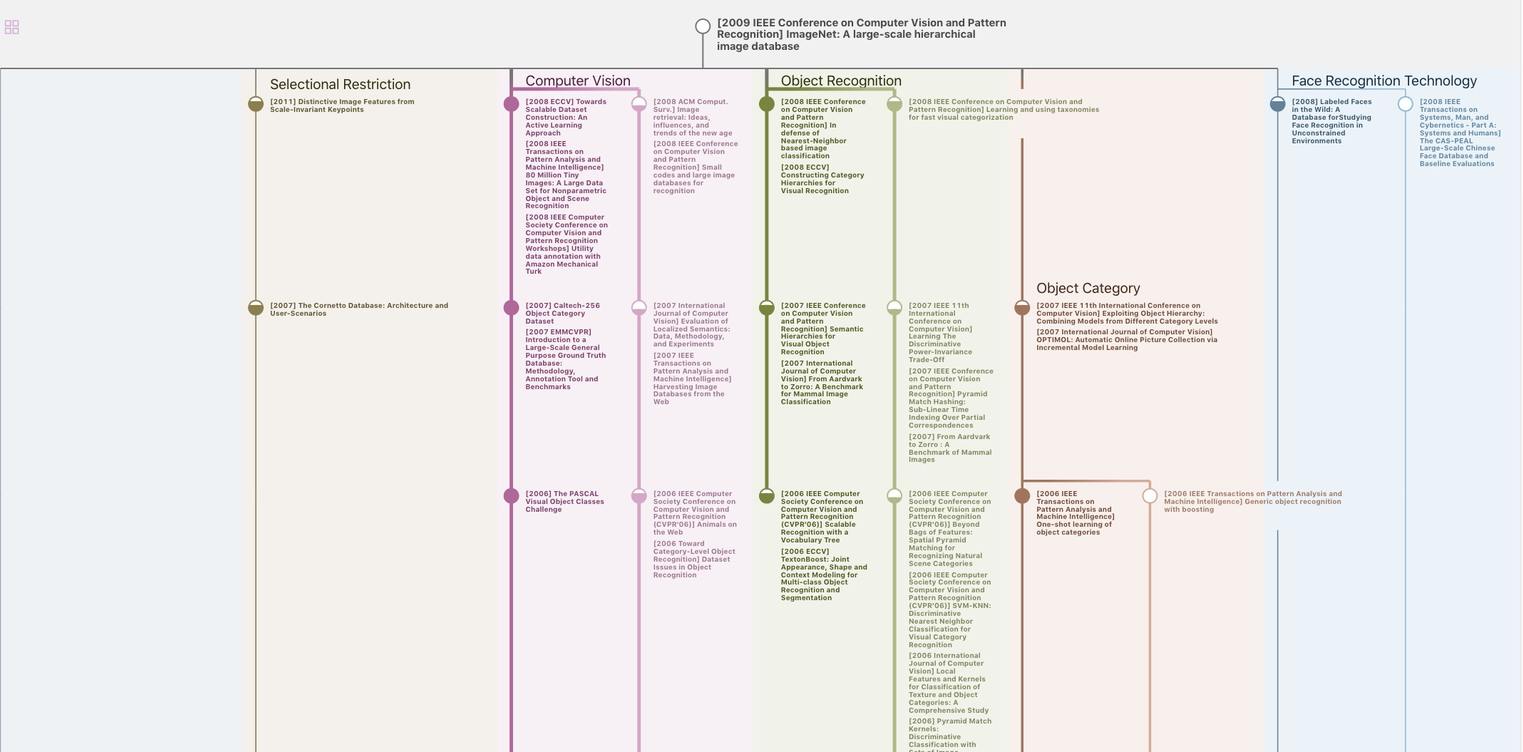
Generate MRT to find the research sequence of this paper
Chat Paper
Summary is being generated by the instructions you defined