Community Selection for Multivariate KPI Predictions in a 2-Tier System.
NOMS(2023)
摘要
Efficient predictions of Key Performance Indicators (KPIs) are essential for efficient management of complex computing and communication systems. Specific management operations often concern only a small number of KPIs, the so-called “targets”. Since different components are interconnected with each other in the systems, we can extract useful information from related KPIs to improve the predictions of the targets. We refer to the set of a target and its related KPIs as a community. In this work, we propose four community selection methods. The first two methods use Pearson correlations-based filters for feature selection over local sets of KPIs, while the two others combine the feature selection with graph summarization techniques to make use of common dependency patterns across the network topology. We apply these methods to some existing prediction methods, namely Single Layer Perceptron, Vector Autoregressive model and Amazon DeepAR, and demonstrate the improvement of prediction accuracies. We then compare our methods with the Graph Time Series, a Graph Neural Network approach that explicitly exploit the relationships between multiple variables. We show that our methods have higher accuracies on imperfect real-world data.
更多查看译文
关键词
time series,prediction,multivariate,feature selection,community selection
AI 理解论文
溯源树
样例
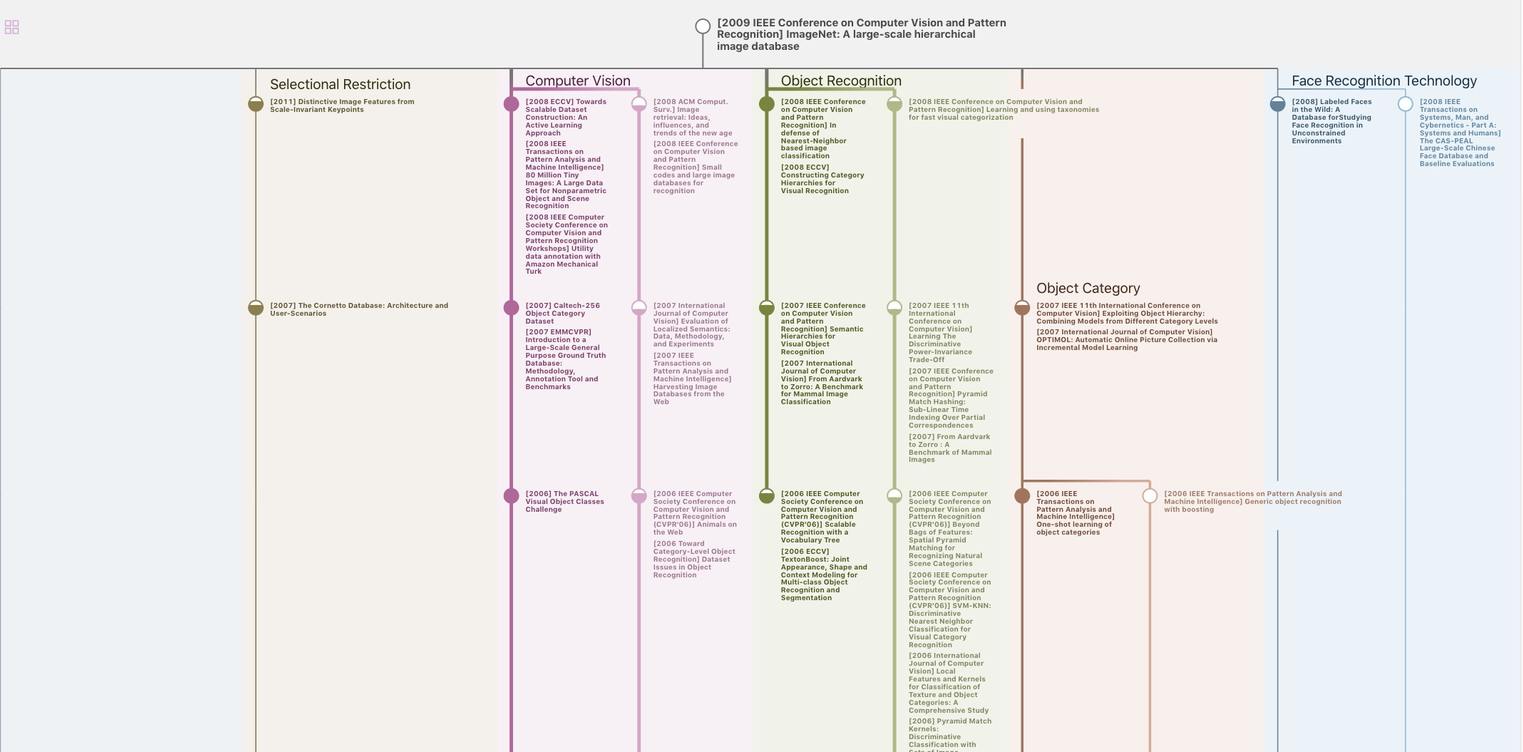
生成溯源树,研究论文发展脉络
Chat Paper
正在生成论文摘要