A Novel Poisoning Attack on Few-Shot based Network Intrusion Detection.
NOMS(2023)
摘要
With the advancement of Machine Learning (ML) algorithms, more organizations started using Machine Learning based Intrusion Detection Systems (ML-IDSs) to mitigate cyberattacks. However, the lack of training datasets is a major challenge when creating those systems. Therefore, using pre-trained models and small amount of labeled network data or few-shots from internal sources are possible solutions to overcome this challenge. However, using pretrained models or external datasets introduces the risk of poisoned machine learning models. This work investigates a novel poisoning attack that creates a diverse mini cluster of attacks and normal instances around an attack instance, then use the instances in that cluster to poison that instance. The poisoned instances are then injected into training data. A trained model is then created by projecting a labeled data from a poisoned source and the few labeled shots from the target organization. An anomaly-based intrusion detection model is utilized to examine the effectiveness of the introduced approach under the proposed poisoning attack. The results have shown that the attack is effective in the context of few-shot IDS learning.
更多查看译文
AI 理解论文
溯源树
样例
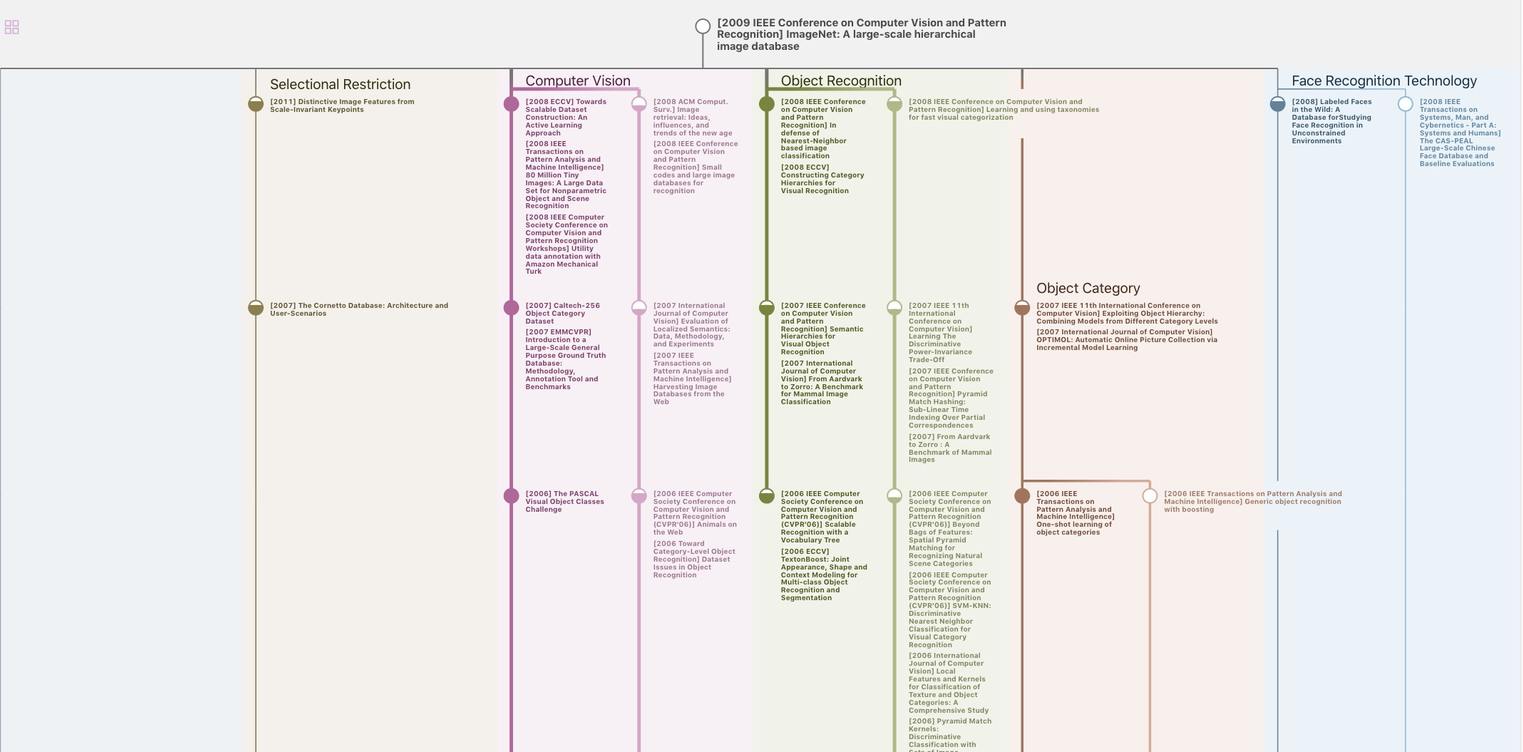
生成溯源树,研究论文发展脉络
Chat Paper
正在生成论文摘要