Efficient Deep-Learning Models for Future Blockage and Beam Prediction for mmWave Systems.
NOMS(2023)
摘要
Massive multiple-input multiple-output (mMIMO) and millimeter waves (mmWaves) are considered to be key technologies for 5G and beyond wireless communications. Massive-MIMO at mmWave frequencies is coupled with advanced beamforming algorithms, to meet the high data rates and stringent latency requirements. The sensitivity of mmWaves to physical obstacles causes significant attenuation of signal leading to link failure between the user and base station (BS). Hence, uninterrupted connectivity to a user can be established via proactive handovers (HOs) between BSs using deep-learning (DL) models for future blockage and beam prediction. In this paper, we present a data-driven neural network approach using Convolution neural networks (CNN) and recurrent neural network (RNN) models for the prediction of future blockages and beams to enable proactive HO and to facilitate seamless connectivity in 5G and beyond ultra-dense networks (UDNs). We validated our proposed deep-learning models’ efficacy for blockage and beam prediction. The evaluation results of CNN and RNN models demonstrate an accuracy of 99% for blockage predictions and more than 90% in the case of beam predictions, with low computational complexity. The proposed DL models have the potential for commercial deployments in next-generation radio access networks (RAN) systems like O-RAN, vRAN, and C-RAN.
更多查看译文
关键词
Deep learning,millimeter wave,beam prediction,blockage prediction,handover
AI 理解论文
溯源树
样例
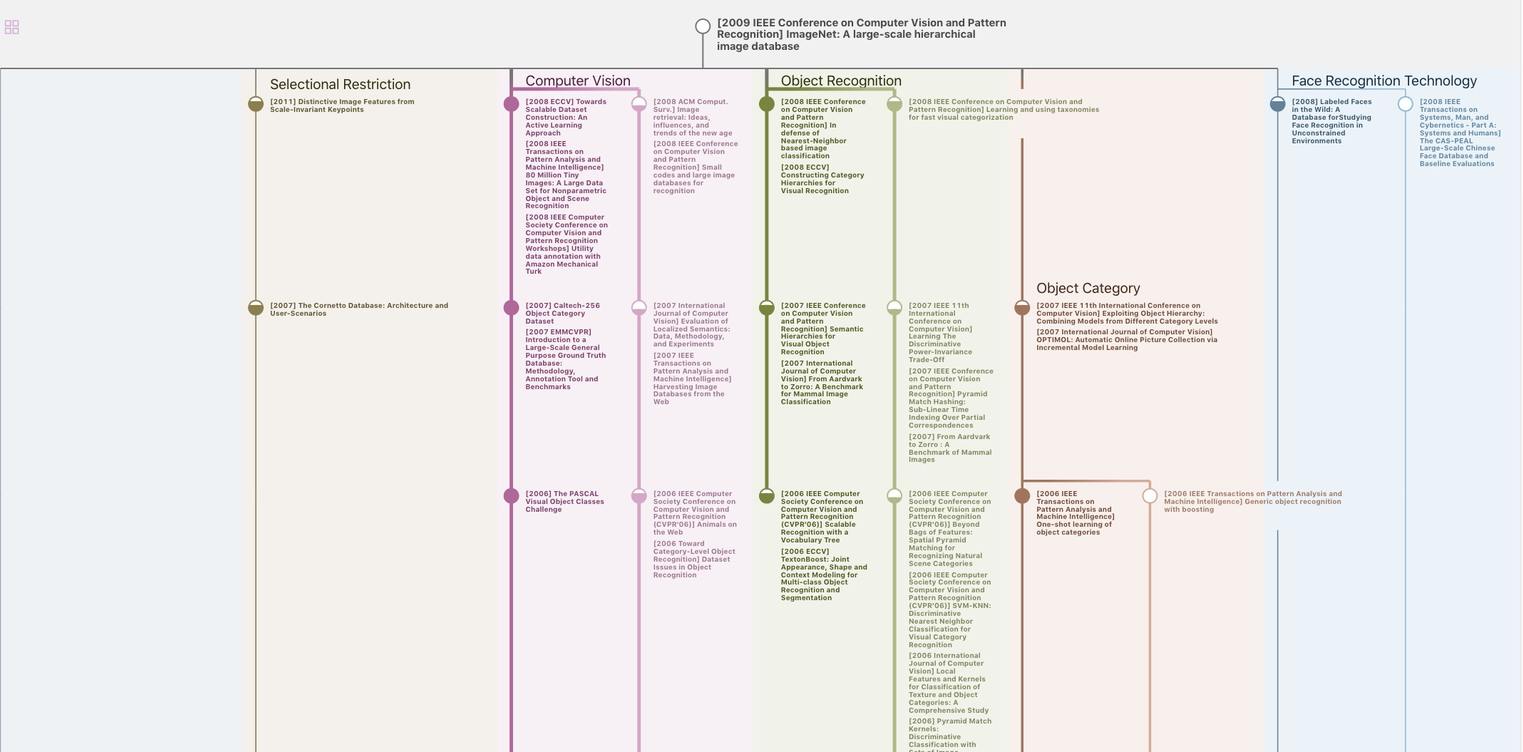
生成溯源树,研究论文发展脉络
Chat Paper
正在生成论文摘要