Sparse-learning-based High-order Dynamic Functional Connectivity Networks for Brain Disease Classification.
ICMLSC(2023)
摘要
Dynamic functional connectivity network (DFCN) derived from resting-state functional magnetic resonance imaging (rs-fMRI), which characterizes the dynamic interaction between brain regions, has been applied to classification of brain diseases. However, existing studies usually focus on dynamic changes of low-order (i.e., pairwise) correlation of brain regions, thus neglecting their high-order dynamic information that could be important for brain disease diagnosis. Therefore, in this paper, we first propose a novel sparse learning based high-order DFCNs construction method, and then build a novel learning framework to extract high-level and high-order temporal features from the constructed high-order DFCNs for brain disease classification. The experimental results on 174 subjects from from the Alzheimer’s Disease Neuroimaging Initiative (ADNI) demonstrate the effectiveness of our proposed method in comparison with state-of-the-art methods.
更多查看译文
AI 理解论文
溯源树
样例
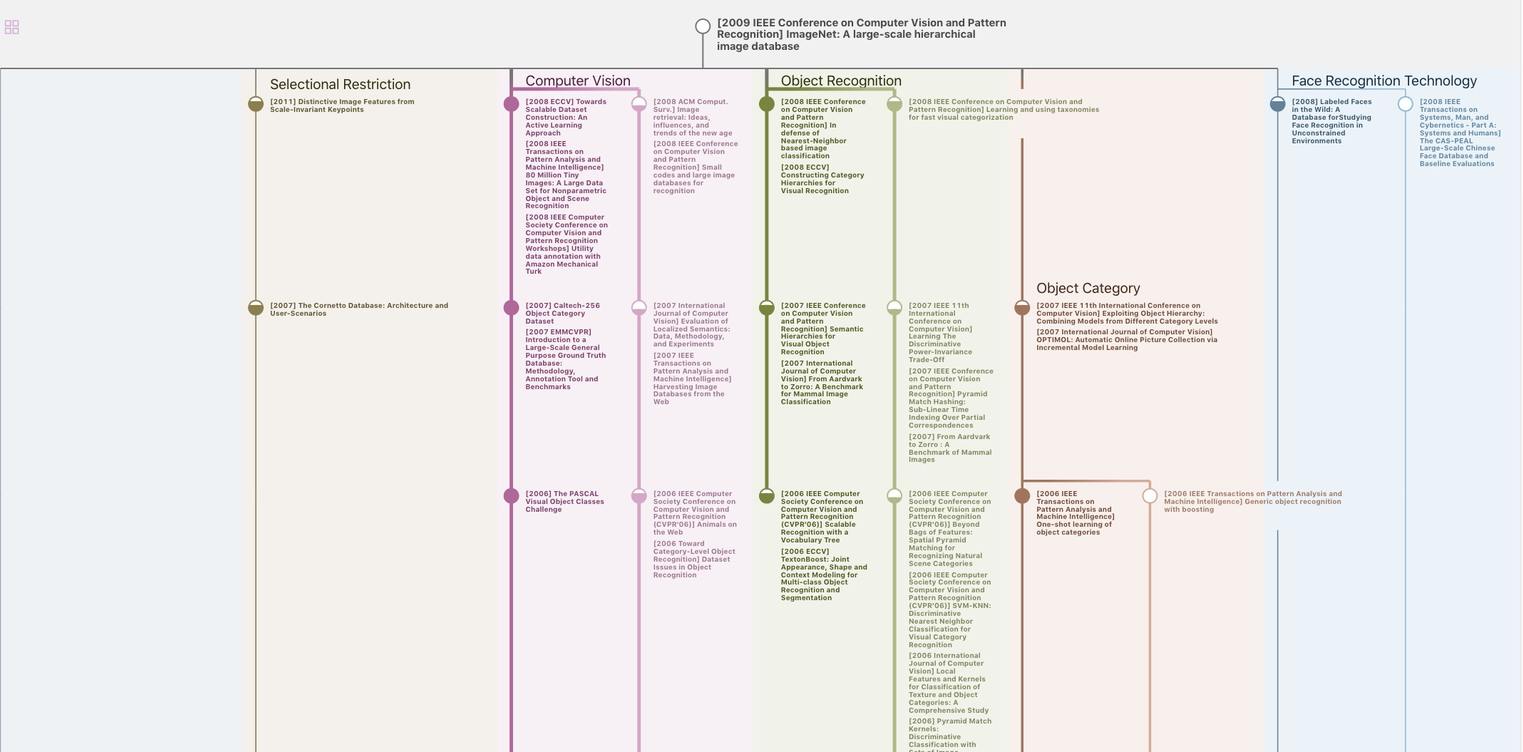
生成溯源树,研究论文发展脉络
Chat Paper
正在生成论文摘要