Limits of Data Driven Steganography Detectors.
IH&MMSec(2023)
摘要
While deep learning has revolutionized image steganalysis in terms of performance, little is known about howmuch modern data driven detectors can still be improved. In this paper, we approach this difficult and currently wide open question by working with artificial but realistic looking images with a known statistical model that allows us to compute the detectability of modern content-adaptive algorithms with respect to the most powerful detectors. Multiple artificial image datasets are crafted with different levels of content complexity and noise power to assess their influence on the gap between both types of detectors. Experiments with SRNet as the heuristic detector indicate that independent noise contributes less to the performance gap than content of the same MSE. While this loss is rather small for smooth images, it can be quite large for textured images. A network trained on many realizations of a fixed textured scene will, however, recuperate most of the loss, suggesting that networks have the capacity to approximately learn the parameters of a cover source narrowed to a fixed scene.
更多查看译文
关键词
Steganography, steganalysis, deep learning, artificial source, performance limit
AI 理解论文
溯源树
样例
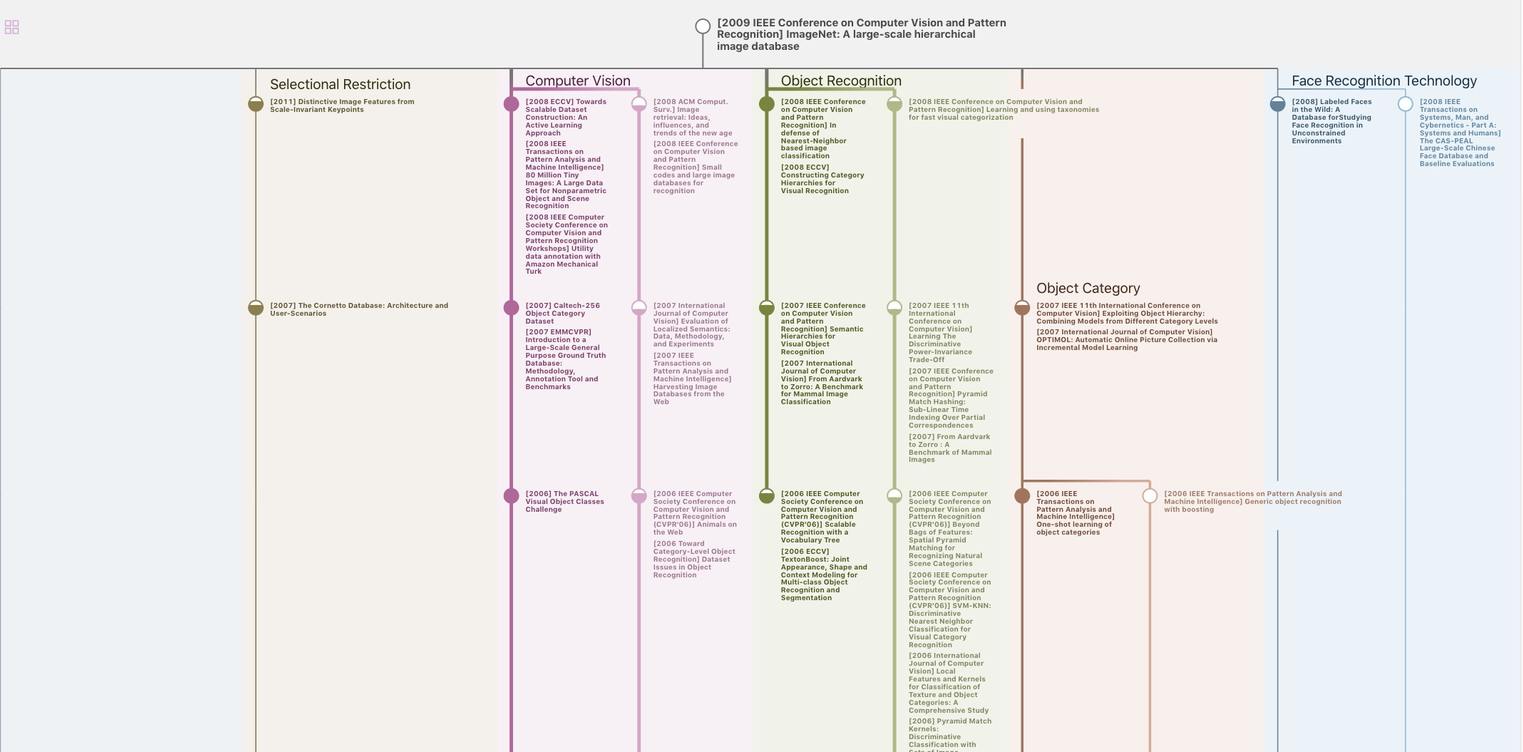
生成溯源树,研究论文发展脉络
Chat Paper
正在生成论文摘要