SCL-Stega: Exploring Advanced Objective in Linguistic Steganalysis using Contrastive Learning.
IH&MMSec(2023)
摘要
Text steganography is becoming increasingly secure by eliminating the distribution discrepancy between normal and stego text. On the other hand, the existing cross-entropy-based steganalysis models struggle to distinguish subtle distribution differences and lack robustness regarding confusable samples. To enhance steganalysis accuracy on hard-to-detect samples, this paper draws on contrastive learning to design a text steganalysis framework incorporating supervised contrastive loss into the training process. This framework improves feature representation by pushing apart embeddings from different classes while pulling closer embeddings from the same class. The experimental results show that our method makes remarkable improvement compared to the four baseline models. Additionally, as the embedding rate increases, our method's advantages become increasingly apparent, with maximum improvements of 13.98%, 12.47%, and 13.65% over the baseline methods across three common linguistic steganalysis datasets, Twitter, IMDB, and News, respectively. Our code is available at https://github.com/katelinglt/SCL-Stega.
更多查看译文
关键词
Deep Neural Network, Linguistic Steganalysis, Supervised Contrastive Learning, Pre-trained Language Model
AI 理解论文
溯源树
样例
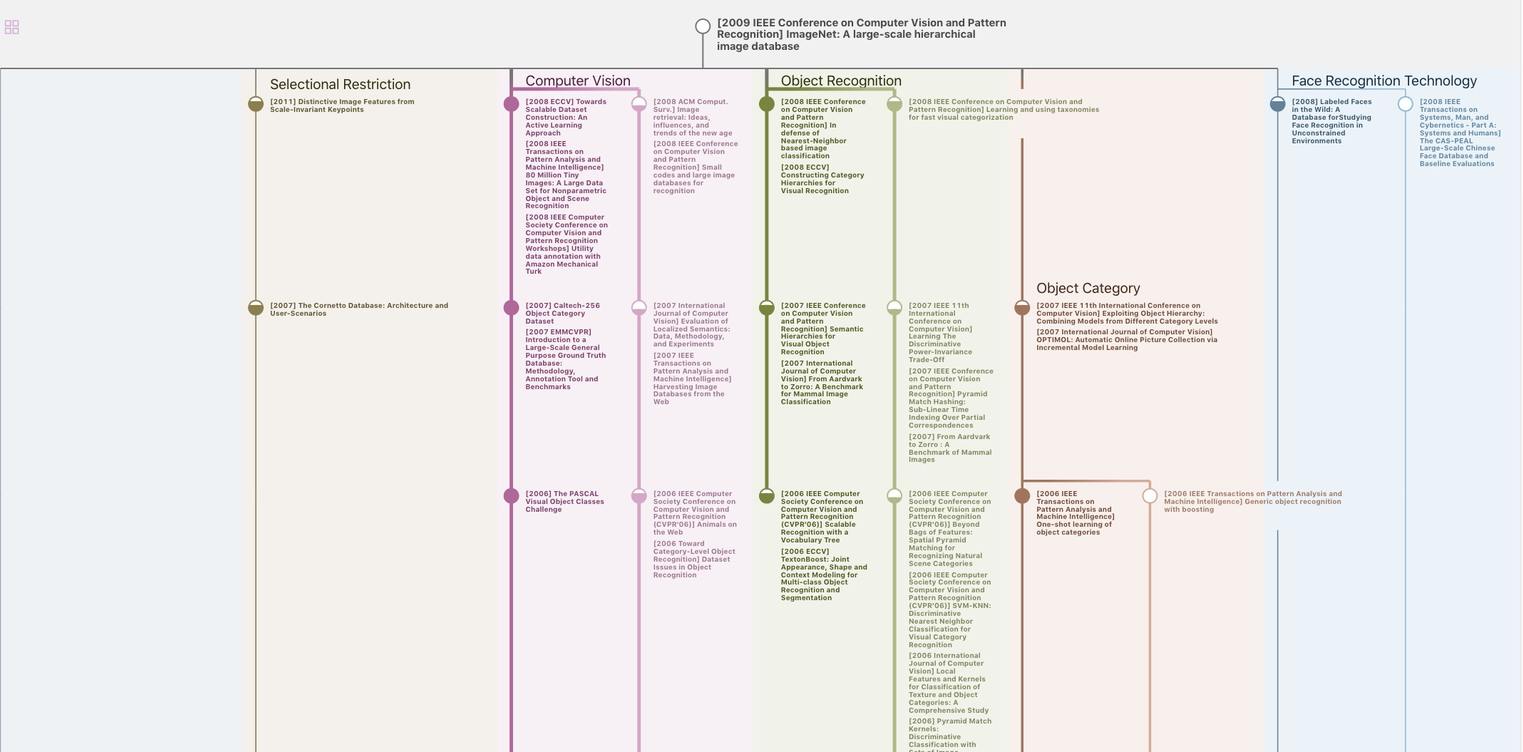
生成溯源树,研究论文发展脉络
Chat Paper
正在生成论文摘要