Batch Integrated Gradients: Explanations for Temporal Electronic Health Records.
AIME(2023)
摘要
eXplainable Artifical Intelligence (XAI) is integral for the usability of black-box models in high-risk domains. Many problems in such domains are concerned with analysing temporal data. Namely, we must consider a sequence of instances that occur in time, and explain why the prediction transitions from one time point to the next. Currently, XAI techniques do not leverage the temporal nature of data and instead treat each instance independently. Therefore, we introduce a new approach advancing the Integrated Gradients method developed in the literature, namely the Batch-Integrated Gradients (Batch-IG) technique that (1) produces explanations over a temporal batch for instance-to-instance state transitions and (2) takes into account features that change over time. In Electronic Health Records (EHRs), we see patient records can be stored in temporal sequences. Thus, we demonstrate Batch-Integrated Gradients in producing explanations over a temporal sequence that satisfy proposed properties corresponding to XAI for EHR data.
更多查看译文
关键词
integrated gradients,records,health
AI 理解论文
溯源树
样例
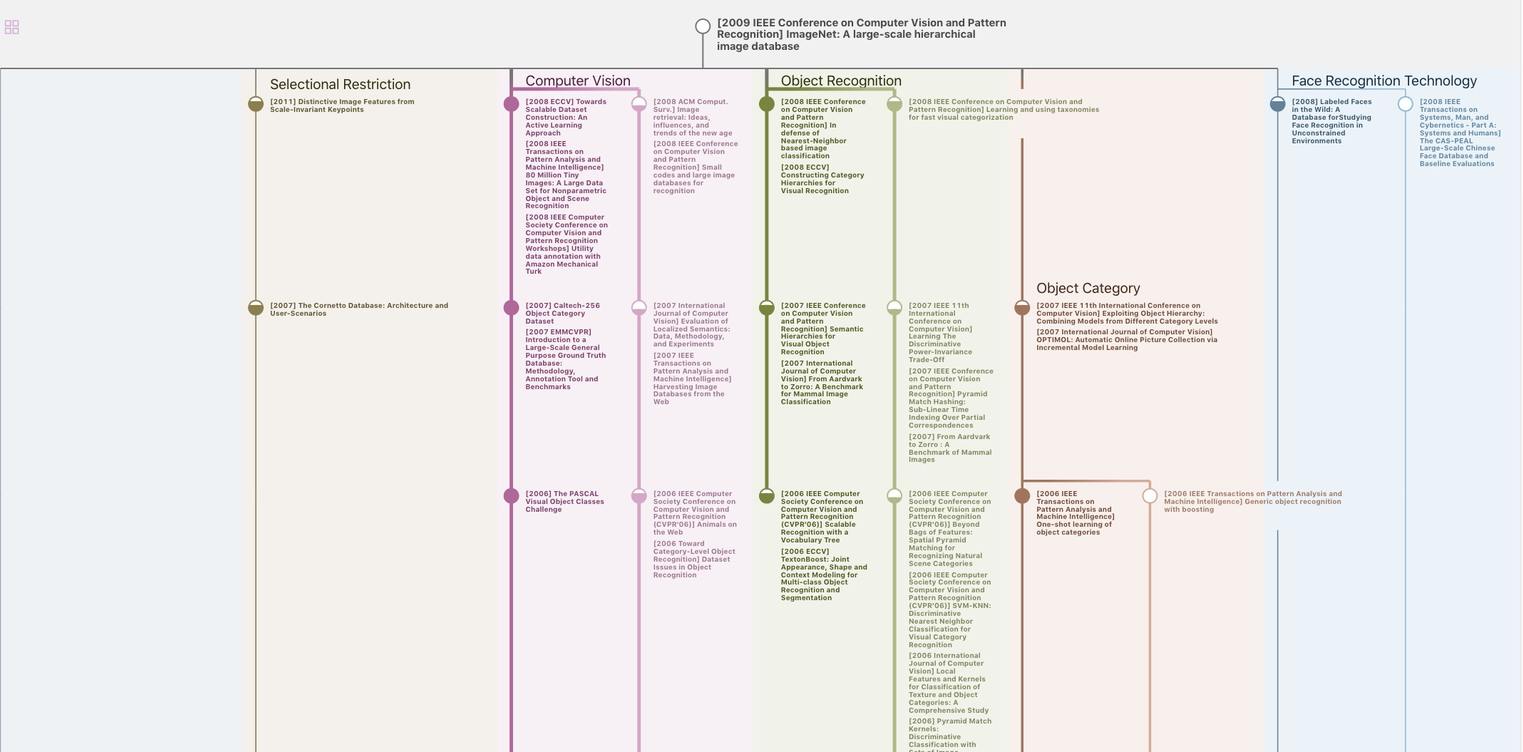
生成溯源树,研究论文发展脉络
Chat Paper
正在生成论文摘要