BERT for Complex Systematic Review Screening to Support the Future of Medical Research.
AIME(2023)
Abstract
This work presents a Natural Language Processing approach to screen complex datasets of medical articles to provide timely and efficient response to pressing issues in medicine. The approach is based on the Bidirectional Encoder Representation from Transformers (BERT) to screen the articles using their titles and abstracts. Systematic review screening is a classification task aiming at selecting articles fulfilling the criteria for the next step of the review. A number of BERT models are fine-tuned for this classification task. Two challenging space medicine systematic review datasets that include human, animal, and in-vitro studies are used for the evaluation of the models. Backtranslation is used as a data augmentation technique to handle the class imbalance and a performance comparison of the models on the original and augmented data is presented. The BERT models provide an accessible solution for screening systematic reviews, which are considered complex and time-consuming. The proposed approach can change the workflow of conducting these types of reviews, especially in response to urgent policy and practice questions in medicine. The source code and datasets are available on GitHub: https://github.com/ESA-RadLab/BERTCSRS .
MoreTranslated text
Key words
complex systematic review,systematic review,medical research,bert,screening
AI Read Science
Must-Reading Tree
Example
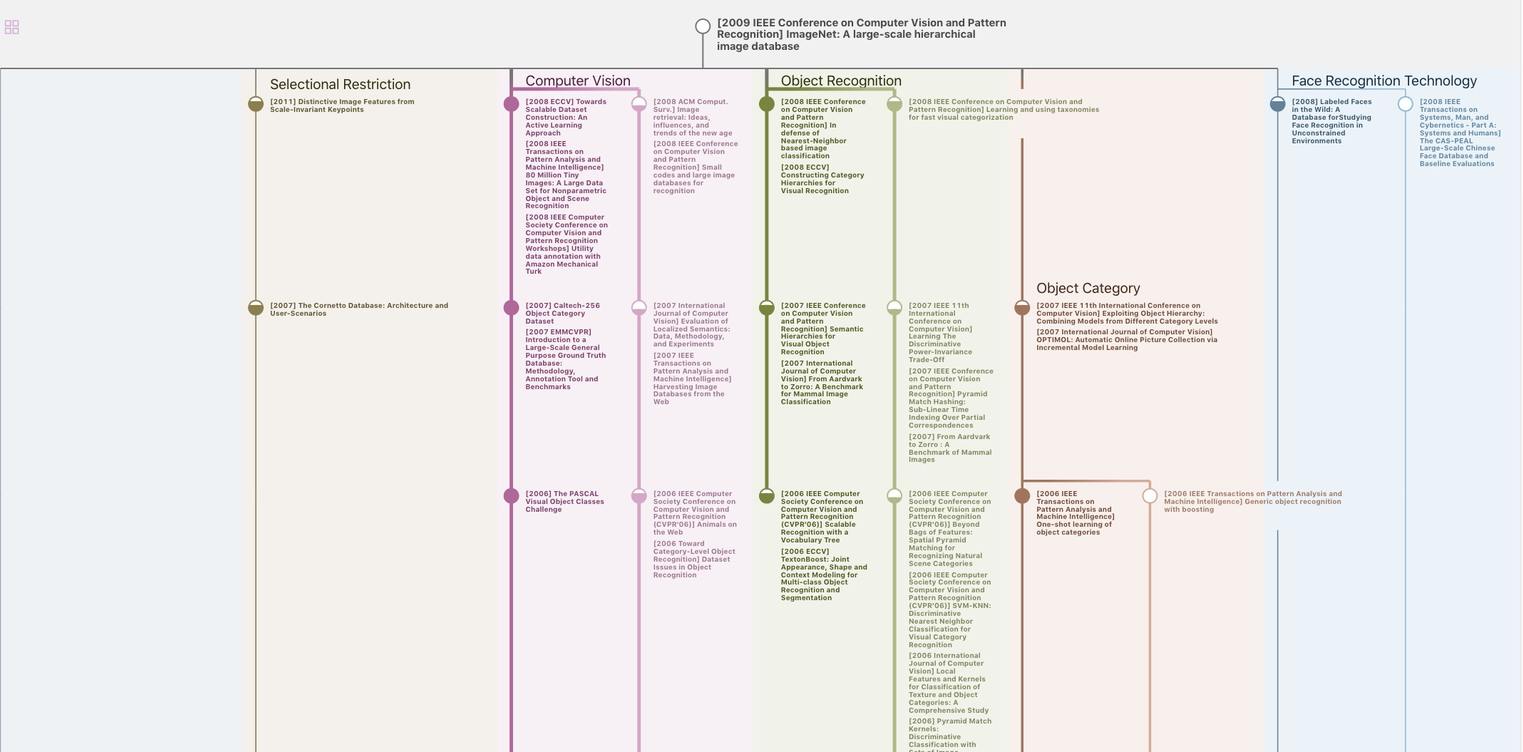
Generate MRT to find the research sequence of this paper
Chat Paper
Summary is being generated by the instructions you defined