The Impact of Importance-Aware Dataset Partitioning on Data-Parallel Training of Deep Neural Networks.
DAIS(2023)
摘要
Deep neural networks used for computer vision tasks are typically trained on datasets consisting of thousands of images, called examples. Recent studies have shown that examples in a dataset are not of equal importance for model training and can be categorized based on quantifiable measures reflecting a notion of “hardness” or “importance”. In this work, we conduct an empirical study of the impact of importance-aware partitioning of the dataset examples across workers on the performance of data-parallel training of deep neural networks. Our experiments with CIFAR-10 and CIFAR-100 image datasets show that data-parallel training with importance-aware partitioning can perform better than vanilla data-parallel training, which is oblivious to the importance of examples. More specifically, the proper choice of the importance measure, partitioning heuristic, and the number of intervals for dataset repartitioning can improve the best accuracy of the model trained for a fixed number of epochs. We conclude that the parameters related to importance-aware data-parallel training, including the importance measure, number of warmup training epochs, and others defined in the paper, may be considered as hyperparameters of data-parallel model training.
更多查看译文
关键词
dataset,neural networks,importance-aware,data-parallel
AI 理解论文
溯源树
样例
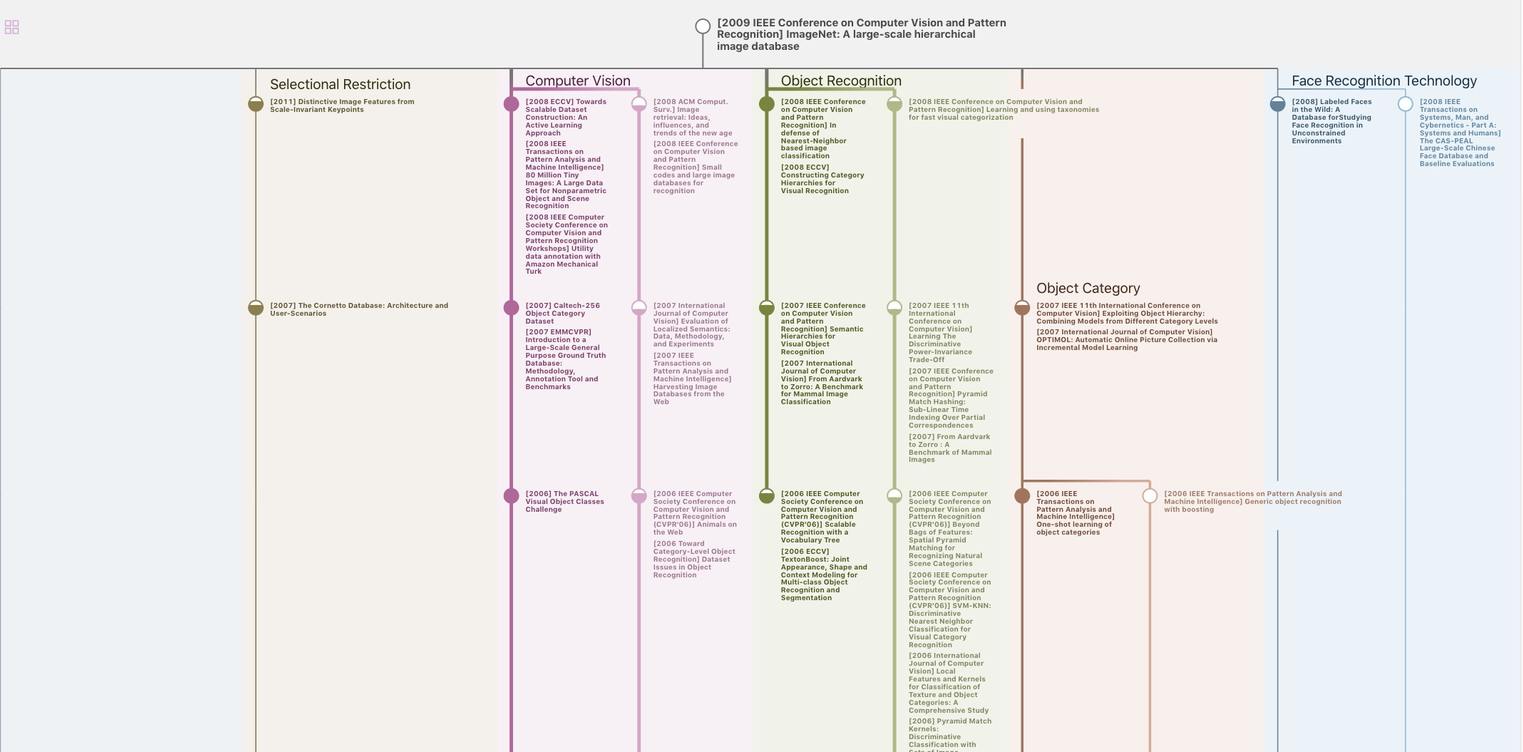
生成溯源树,研究论文发展脉络
Chat Paper
正在生成论文摘要