A Missing Piece in the Puzzle: Considering the Role of Task Complexity in Human-AI Decision Making
UMAP(2023)
摘要
Recent advances in the performance of machine learning algorithms have led to the adoption of AI models in decision making contexts across various domains such as healthcare, finance, and education. Different research communities have attempted to optimize and evaluate human-AI team performance through empirical studies by increasing transparency of AI systems, or providing explanations to aid human understanding of such systems. However, the variety in decision making tasks considered and their operationalization in prior empirical work, has led to an opacity around how findings from one task or domain carry forward to another. The lack of a standardized means of considering task attributes prevents straight-forward comparisons across decision tasks, thereby limiting the generalizability of findings. We argue that the lens of 'task complexity' can be used to tackle this problem of under-specification and facilitate comparison across empirical research in this area. To retrospectively explore how different HCI communities have considered the influence of task complexity in designing experiments in the realm of human-AI decision making, we survey literature and provide an overview of empirical studies on this topic. We found a serious dearth in the consideration of task complexity across various studies in this realm of research. Inspired by Robert Wood's seminal work on the construct, we operationalized task complexity with respect to three dimensions (component, coordinative, and dynamic) and quantified the complexity of decision tasks in existing work accordingly. We then summarized current trends and proposed research directions for the future. Our study highlights the need to account for task complexity as an important design choice. This is a first step to help the scientific community in drawing meaningful comparisons across empirical studies in human-AI decision making and to provide opportunities to generalize findings across diverse domains and experimental settings.
更多查看译文
AI 理解论文
溯源树
样例
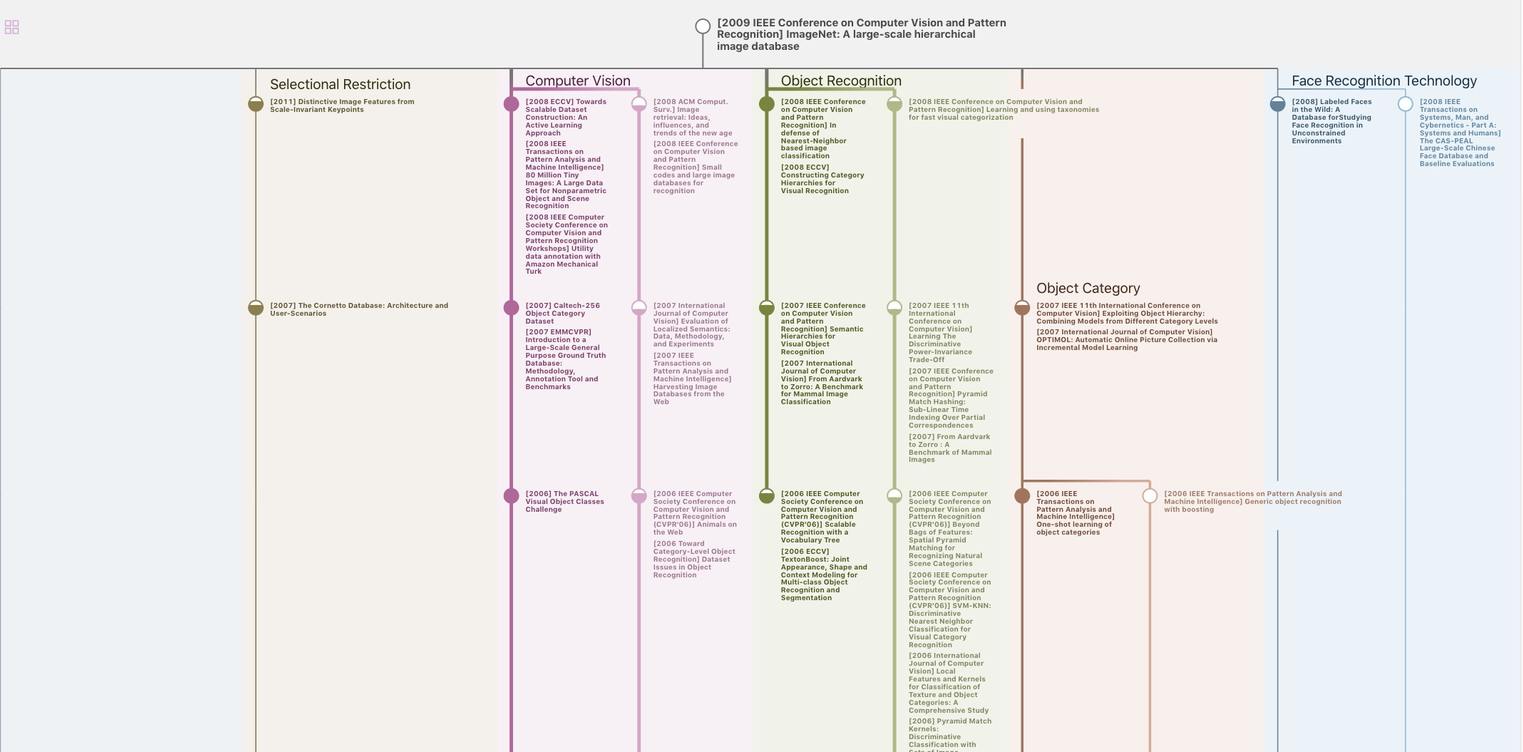
生成溯源树,研究论文发展脉络
Chat Paper
正在生成论文摘要