Investigating the effectiveness of persuasive justification messages in fair music recommender systems for users with different personality traits
UMAP(2023)
摘要
In recent decades, music recommender systems have become increasingly popular and have attracted a lot of research attention. While there has been significant progress in algorithm design to improve the quality of recommendations for listeners, there are new research challenges arising in large scale systems which have to consider the interests of both listeners and artists. To ensure a sustainable community of artists and a diversity of genres, artists, and songs, the recommender needs to ensure that new artists have a chance to be heard and rated. So, in addition to the objective of optimizing the recommendation to the preferences and enjoyment of the listener, a large scale MRS has a "fairness" objective to provide new artists (the protected group) with an opportunity to be heard. Previous research shows that using persuasive explanations can increase user acceptance of the recommended items. We propose to use persuasive justification messages for songs of new artists to influence user acceptance and satisfaction with these recommendations. The messages are designed to implement the six popular Cialdini persuasive strategies. We explore the effects of different persuasive messages on users with different Big-5 (OCEAN) personality types in an online study (n=205). The findings show that users with different personality traits are receptive to different persuasive messages and suggest how to personalize the persuasive justifications to amplify their effect for users with different personalities. These results can guide the development of personalized/adaptive persuasive recommendation justifications for fair music recommender systems leading to a better user satisfaction and mitigating the "rich get richer" effect in large-scale music recommender systems, ensuring diversity of content and sustainability of the community.
更多查看译文
关键词
Music recommender systems,Fairness,User experience,Personality,Persuasion
AI 理解论文
溯源树
样例
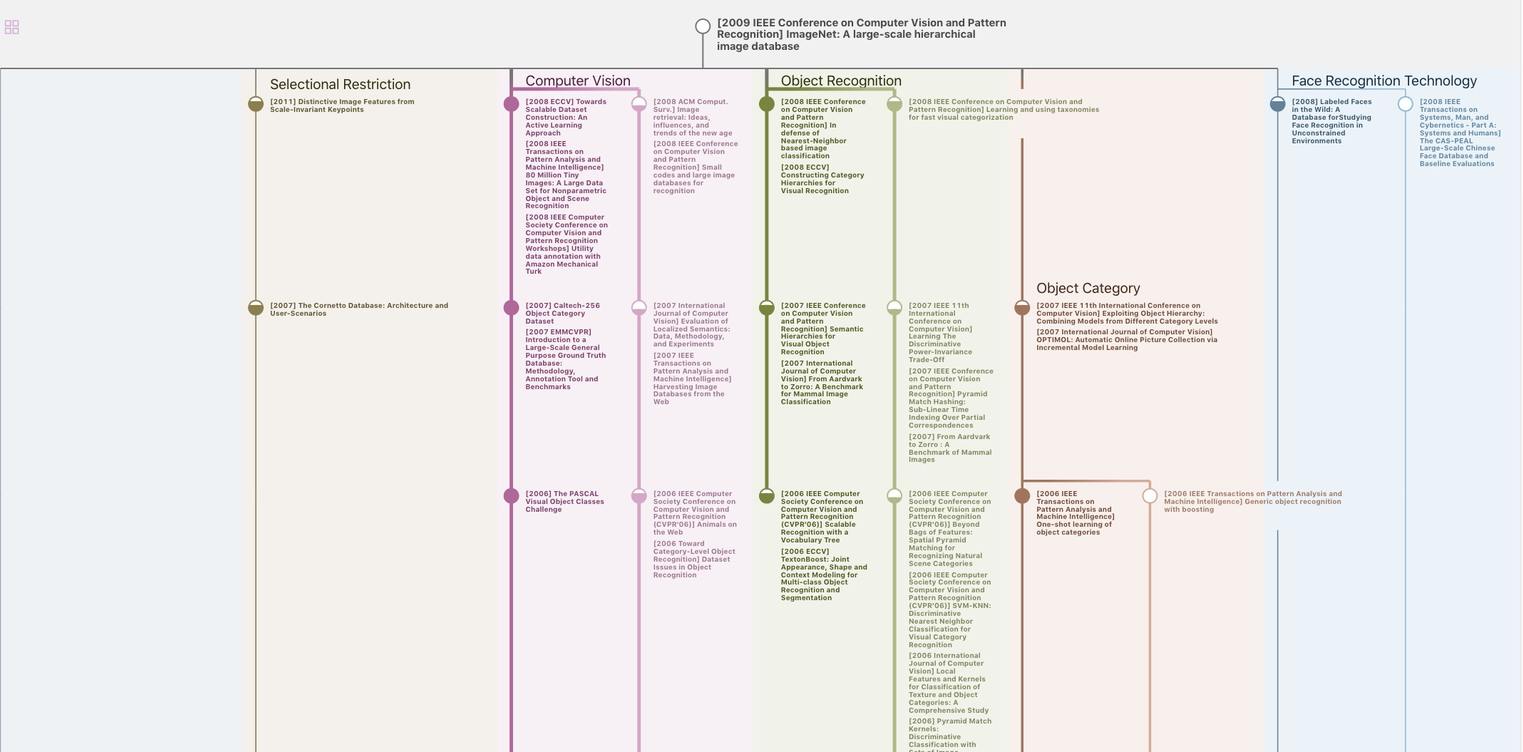
生成溯源树,研究论文发展脉络
Chat Paper
正在生成论文摘要