How can we model climbers' future visits from their past records?
2023 ADJUNCT PROCEEDINGS OF THE 31ST ACM CONFERENCE ON USER MODELING, ADAPTATION AND PERSONALIZATION, UMAP 2023(2023)
摘要
Outdoor sport climbing is one of the major outdoor sports in Northern Italy due to the vast number of rock climbing places named crags. New crags appear yearly, creating an information overload problem for climbers when they plan where to climb on their forthcoming trips. As such, climbing crags recommender systems address this problem, suggesting crags based on the number of routes similar to those liked by the user in the past. At the same time, people are interested not only in the climbing routes but they have other objectives; for instance, they plan to teach their children to climb while traveling, or prefer to visit the place only if it has a parking space since they travel by car. To better understand and model future climbers' choices in outdoor sport climbing environments, we first define crags characteristics that primarily impact a user's behavior. Secondly, we propose to model climbers' profiles and their preferences for crags' characteristics as Pearson correlation computed with the number of past users' visits. Thirdly, we developed and evaluated the recommender system called Visit&Climb, where user tastes are projected into an interactive preferences elicitation panel, which users can further employ to adjust their profile with the sliders. The recommendations are then supplied as the top-visited crags by the most similar user. For the evaluation, we ran several offline experiments: we compared different models (regression-based, matrix factorization, and collaborative filtering) to predict visits recorded in 99 crags by 106 climbers in Arco (Italy). During these experiments, we measured standard metrics such as MaP@k, Recall@k, and NDCG@k for top-k ranking quality. The offline evaluation showed that the Visit&Climb system provides more accurate recommendations than the Baseline model, which utilizes users' previous records for future prediction. Plus, it delivers a comparable accuracy level to other systems in this domain. Moreover, unlike the other solutions, this developed method visualizes the users' profiles and allows modification of their tastes, solving a cold-start issue. The recommender system proposed in this work can confidently model climbers' future visits by their past logs.
更多查看译文
关键词
user modeling,ranking aggregation,sport climbing,top-K recommender systems
AI 理解论文
溯源树
样例
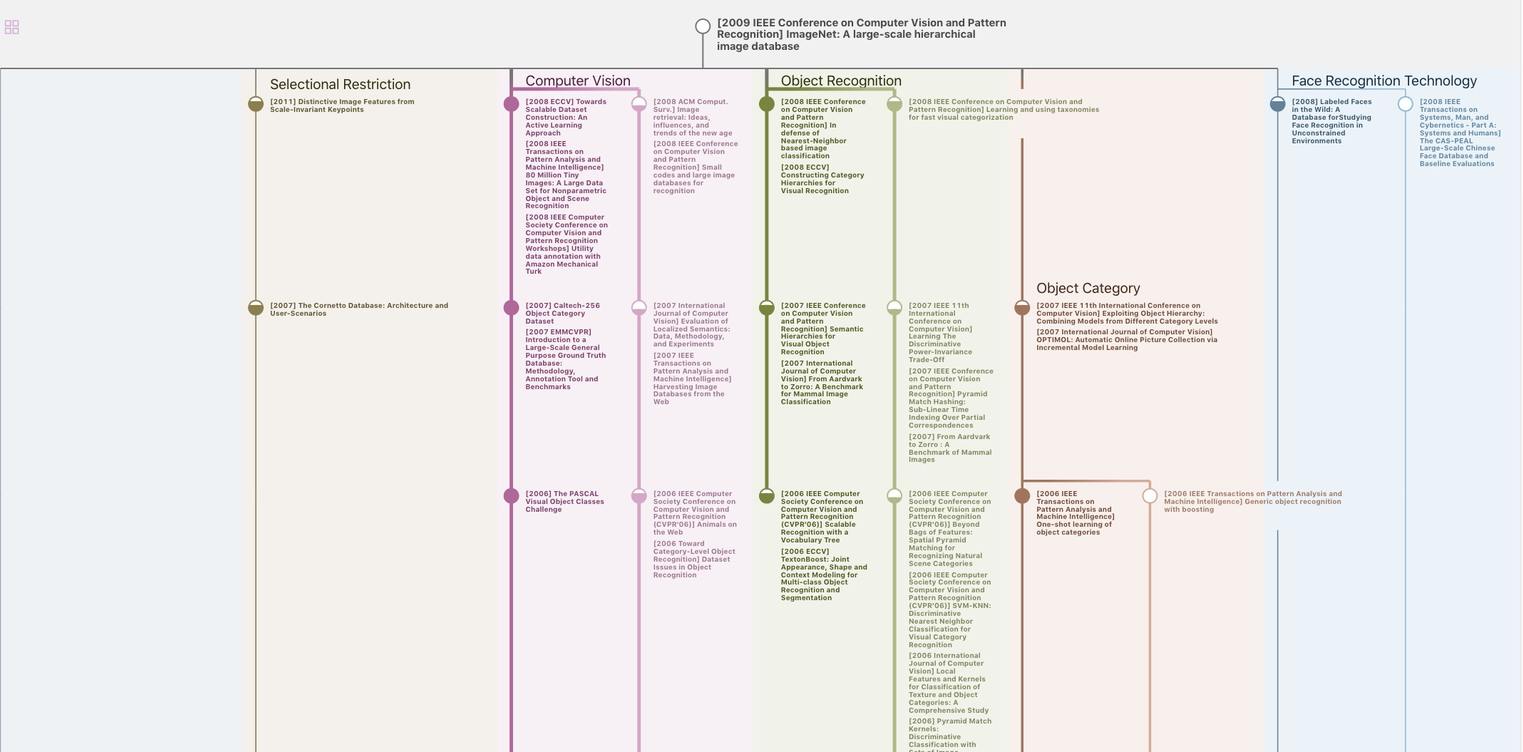
生成溯源树,研究论文发展脉络
Chat Paper
正在生成论文摘要